ChatGPT vs. Human Annotators: A Comprehensive Analysis of ChatGPT for Text Annotation.
International Conference on Machine Learning and Applications(2023)
摘要
In recent years, the field of Natural Language Processing (NLP) has witnessed a groundbreaking transformation with the emergence of large language models (LLMs). ChatGPT stands out as an example among these LLM models captivating considerable public interest due to its impressive language generation capabilities. Researchers have been exploring the potential of using ChatGPT for data annotation tasks, aiming to discover more timesaving and cost-effective approaches. In this paper, we present a comprehensive evaluation of ChatGPT's data annotation capabilities across ten diverse datasets covering various subject areas and varied number of classes. To ensure the quality of our evaluation, we leveraged datasets that were previously annotated by human experts, providing a reliable benchmark for comparison. Through rigorous experimentation, we assessed the impact of different prompt strategies and model configurations on the annotation performance. Our findings emphasize the capability of ChatGPT in handling most data annotation tasks achieving average accuracy of 78.2% across various tasks. The banking queries dataset stands out with an impressive 95.9% accuracy, while emotions classification presents challenges, yielding an accuracy of 57.5%. Our evaluation also highlights the impact of prompt strategies on annotation performance and reveals significant performance differences between GPT models, with “gpt-4” achieving higher accuracy 79.2% on average compared to “gpt-3.5” of 74.6%. Our research provides valuable insights into the capabilities and limitations of ChatGPT in automating data annotation tasks.
更多查看译文
关键词
ChaptGPT,Data Annotation,Large Language Models
AI 理解论文
溯源树
样例
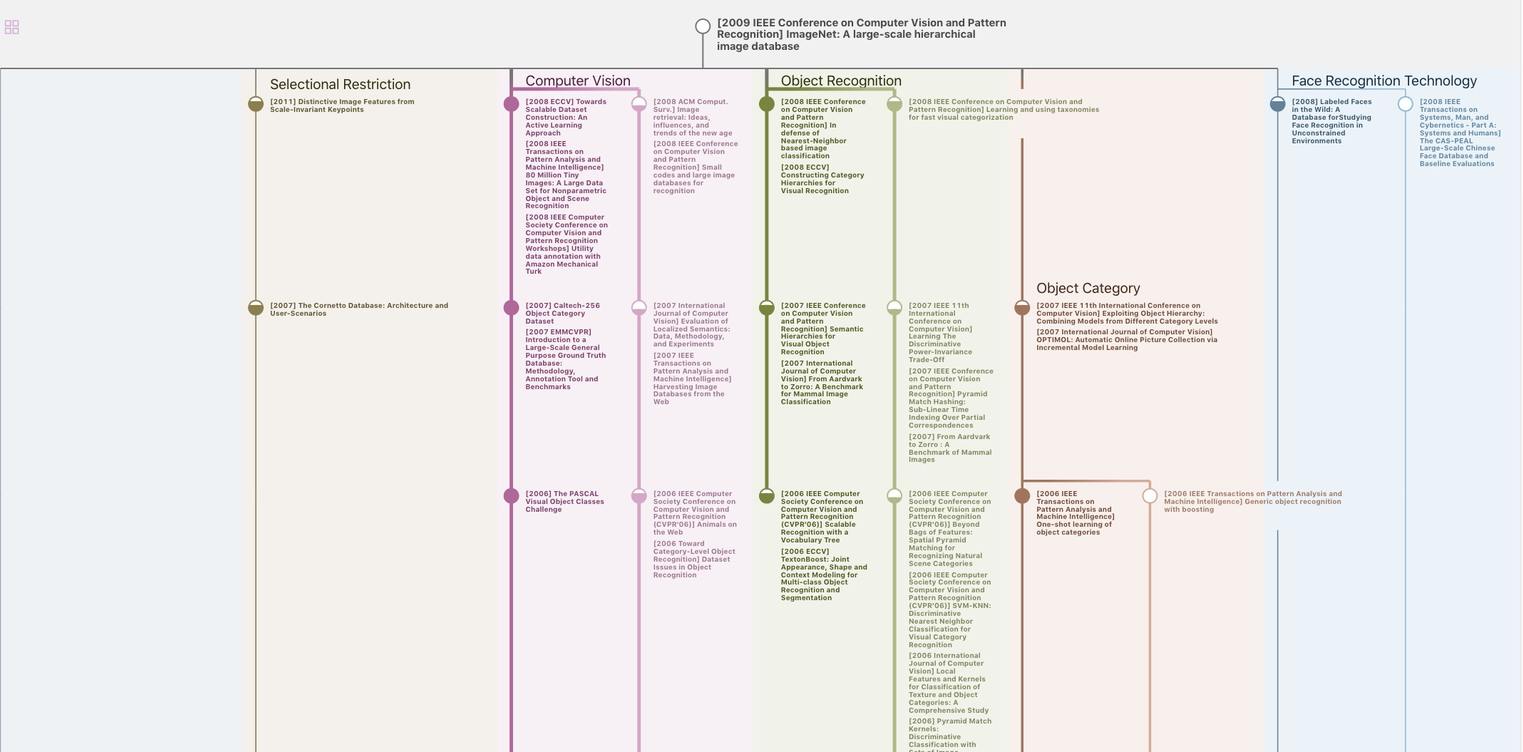
生成溯源树,研究论文发展脉络
Chat Paper
正在生成论文摘要