Blockchain-Based Federated Learning With Enhanced Privacy and Security Using Homomorphic Encryption and Reputation
IEEE Internet of Things Journal(2024)
摘要
Federated learning, leveraging distributed data from multiple nodes to train a common model, allows for the use of more data to improve the model while also protecting the privacy of original data. However, challenges still exist in ensuring privacy and security within the interactions. To address these issues, this paper proposes a federated learning approach that incorporates blockchain, homomorphic encryption, and reputation. Using homomorphic encryption, edge nodes possessing local data can complete the training of ciphertext models, with their contributions to the aggregation being evaluated by a reputation mechanism. Both models and reputations are documented and verified on the blockchain through consensus process, which then determines the rewards based on the incentive mechanism. This approach not only incentivizes participation in training, but also ensures the privacy of data and models through encryption. Additionally, it addresses security risks associated with both data and network attacks, ultimately leading to a highly accurate trained model. To enhance the efficiency of learning and the performance of the model, a joint adaptive aggregation and resource optimization algorithm is introduced. Finally, simulations and analyses demonstrate that the proposed scheme enhances learning accuracy while maintaining privacy and security.
更多查看译文
关键词
Federated learning,blockchain,Industrial Internet of Things (IIoT),privacy,security
AI 理解论文
溯源树
样例
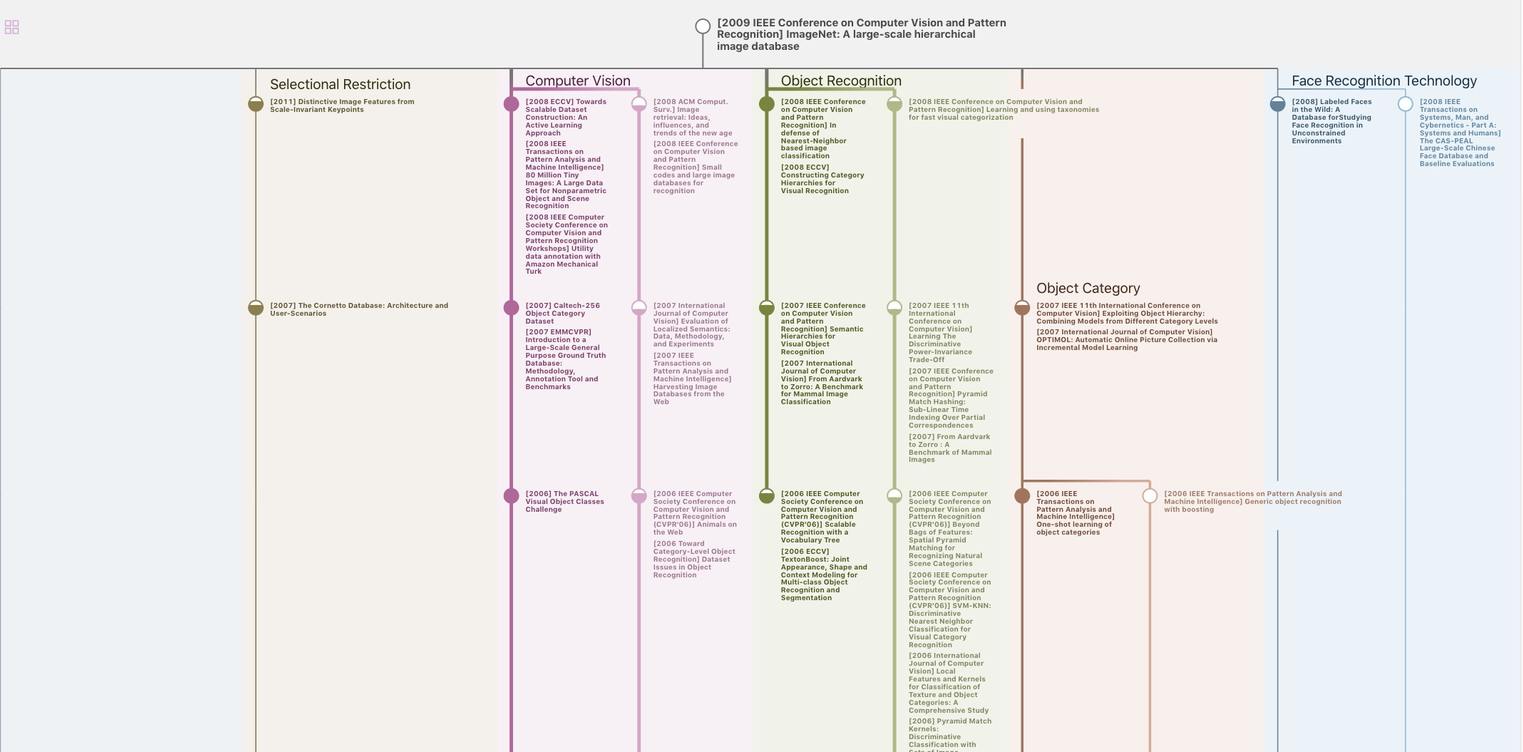
生成溯源树,研究论文发展脉络
Chat Paper
正在生成论文摘要