Few-shot Fault Detection Based on Meta Learning for Transmission Lines
2023 China Automation Congress (CAC)(2023)
摘要
Aiming at the problem of low detection accuracy caused by the small sample size and small size of component defects in the current transmission line inspection, a meta-learning-based few-shot defect detection model for transmission lines, Attention-Meta RCNN (AMR), is proposed. Firstly, the Feature Enhancement Pyramid Network FEPN is designed in the feature extraction stage, which fully fuses image features and improves the RPN's ability to classify positive and negative samples; In the Predictor-head Reweighting Network, Multi-spectral Feature Coding is used to convert the support set image features into class attention vectors; finally, the result of the subtraction of the support set features and query set features is introduced in the feature fusion to reduce the influence of random sampling of the support set. Through training and testing on the self-built transmission line defect dataset, the improved method achieves a mAP value of 76.1% when the number of defective parts is 30, of which the mAP value of defective parts is 70.2%, which has been effectively improved. It is proved that this method can effectively improve the problem of small samples and small size in transmission line defect detection.
更多查看译文
关键词
few-shot learning,meta learning,transmission lines,defects detection
AI 理解论文
溯源树
样例
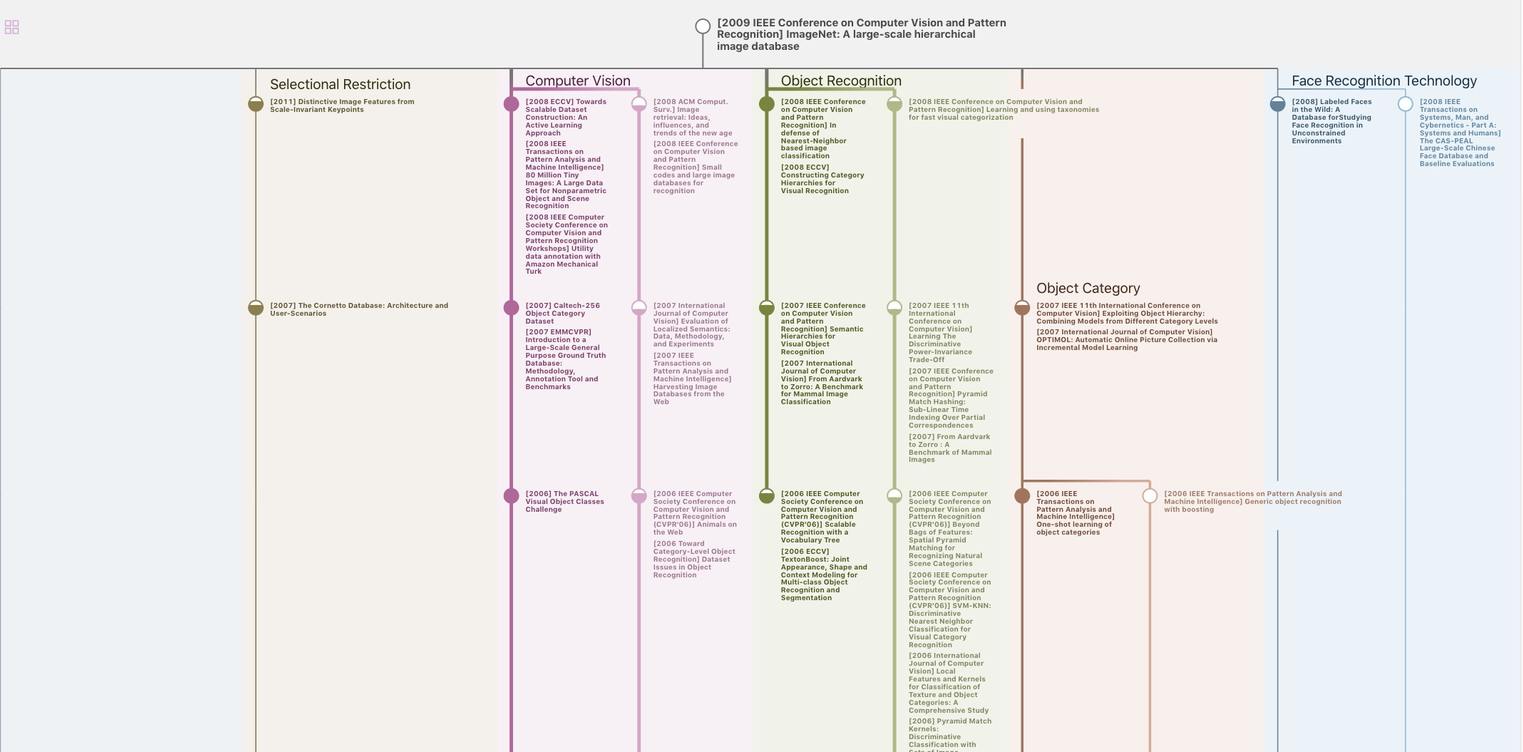
生成溯源树,研究论文发展脉络
Chat Paper
正在生成论文摘要