Development of a one-step office hysteroscopic lightweight artificial intelligence application for subfertility risk stratification of intrauterine adhesions
Fundamental Research(2024)
摘要
Intrauterine adhesions (IUAs) pose challenges to natural fertility and impede timely conception. Assisted reproductive technologies (ART) offer effective solutions but are often expensive in developing nations. This study introduces an Artificial intelligence (AI) application, MobilenetV3PH, featuring a proportional hazard (PH) neural network architecture to predict reproductive outcomes following hysteroscopic adhesiolysis in IUAs and to categorize subfertility risks. The present study leveraged 4922 second-look hysteroscopic images obtained from 555 patients post-hysteroscopic adhesiolysis, sourced from a prospective IUA clinical database (NCT05381376). The prospective cohort was randomly partitioned into training, validation, and test sets for model development, hyperparameter validation, and external validation. MobilenetV3PH achieved a noteworthy Area Under the Curve (AUC) of 0.920, outperforming alternative models significantly. The AI application seamlessly integrated into the hysteroscopic platform, demonstrating an average analysis time of 35.7 ± 5.6 seconds per patient. Notably, it excelled in predicting natural conception compared to conventional clinical scoring methods. The identification of high-risk IUAs patients who encounter difficulties in natural conception through AI-predicted subfertility risks revealed that these individuals might derive greater benefits from ART (HR = 4.616, P = 0.007) compared to their low-risk counterparts (HR = 0.628, P = 0.534). Overall, this AI tool exhibits promise for practical clinical applications, assisting in the prediction of postoperative difficulties in natural conception, patient risk stratification, and management strategies making, ultimately enhancing cost-effective interventions.
更多查看译文
关键词
Subfertility risk stratification,Intrauterine adhesions,image deep learning,lightweight,hysteroscopy
AI 理解论文
溯源树
样例
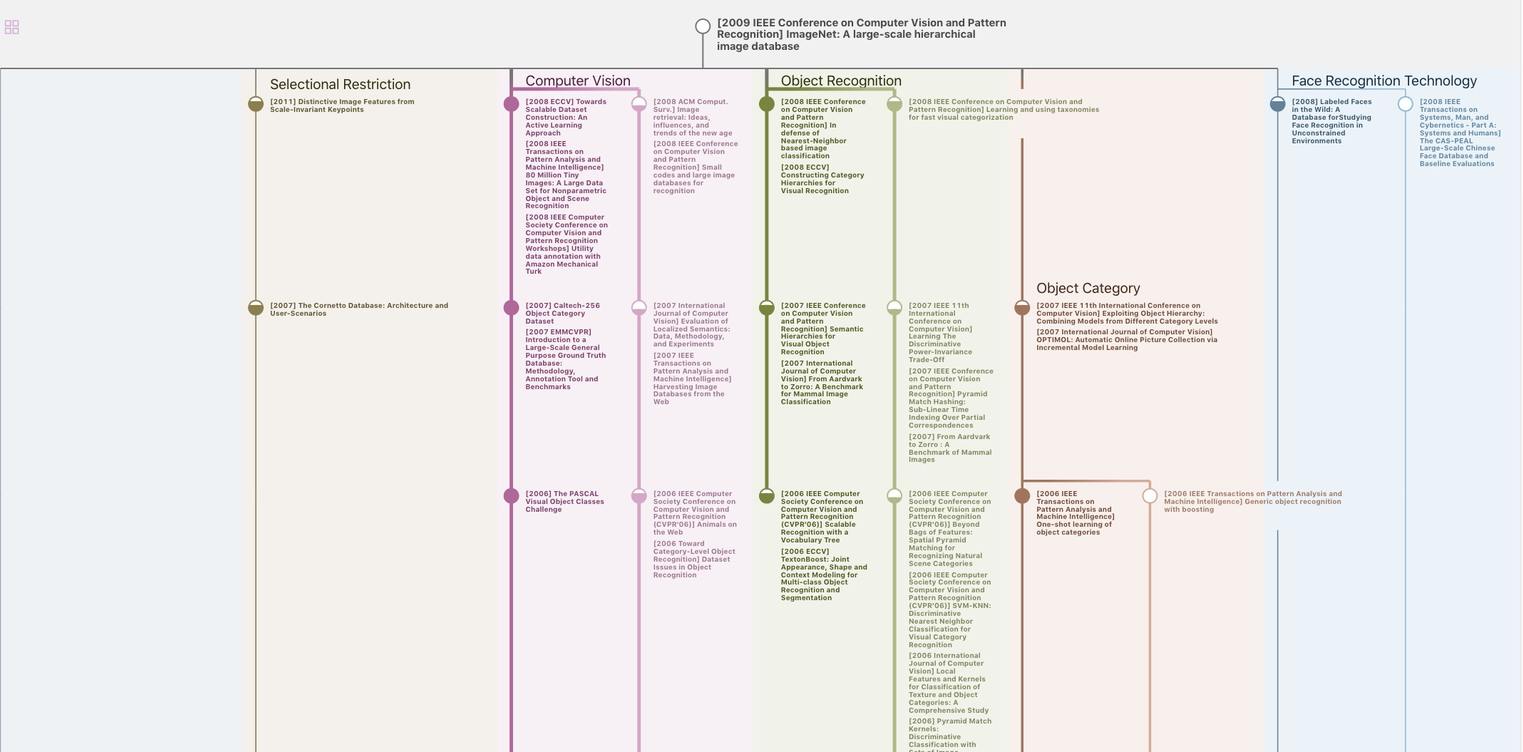
生成溯源树,研究论文发展脉络
Chat Paper
正在生成论文摘要