The Effectiveness of Machine Learning to Estimate the Risk of Failure in Brazilian Public Contracts.
International Conference on Machine Learning and Applications(2023)
摘要
Automatic risk estimation is paramount to prioritizing public contracts auditing efforts, and Machine Learning Risk Prediction (MLRP) models are a promising solution to the classification task of identifying high-risk contracts. Current approaches are focused on the federal level of government, at the same time, face limiting challenges such as the absence of exhaustive ground truth, the difficulty of gaining access to critical databases to build model features, as well as the absence of public literature on the relevance of proposed features. In this work, we attempt to propose MLRP models at the municipal level and overcome those issues by exploring the space of challenges and opportunities in applying MLRP to a setting of Brazilian public contracts. With grounds on the prosecutors' practical experience, we combine three data sources to produce a novel dataset that is more detailed and precise than those used in previous works, first to establish a baseline measuring the gains of applying MLRP to Brazilian Public contracts at the municipal level, second to compare the performance of MLRP and a sample of ad-hoc state-of-the-practice data. Next, we leverage semantic features from contract descriptions in order to evaluate the impact of the contract area on the model's prediction. Also, we experiment using urban and economic characteristics to improve model performance. We measure the impact of access to each datasource on model performance, quantifying the importance of non-open data for this task. Our results suggest that the ad-hoc approach at the firm level has little practical efficacy when evaluated through a more granular/actionable perspective. Contract-level MLRP may be a promising approach, especially when using economic indicators to characterize municipalities, such as GDP per capita. Also, we found no difference between the impact of each feature set on the models' predictions.
更多查看译文
关键词
Machine Learning,feature contribution,risk estimation,public contracts,public administration,Latent Dirichlet Allocation,topic modeling
AI 理解论文
溯源树
样例
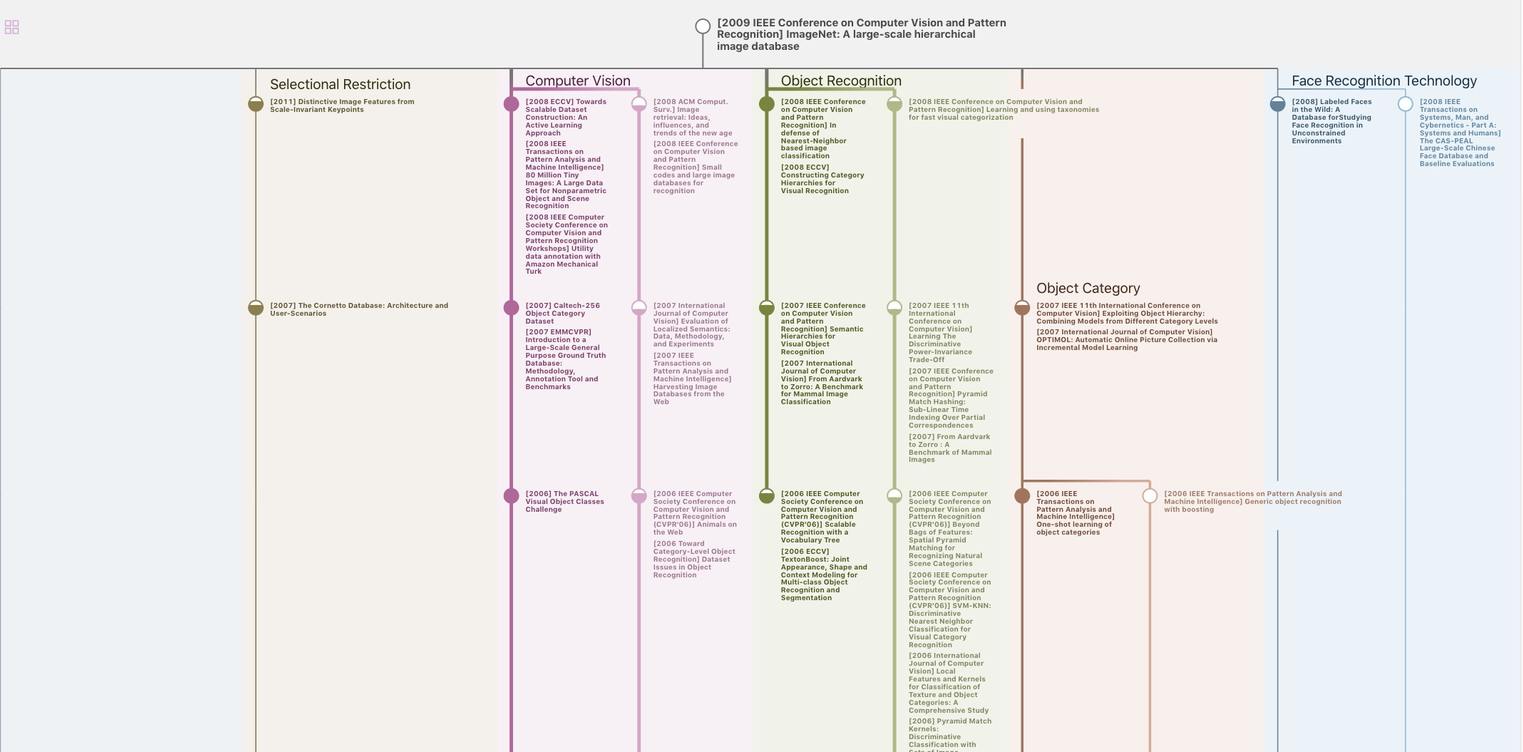
生成溯源树,研究论文发展脉络
Chat Paper
正在生成论文摘要