Mandarin orange (Citrus reticulata Blanco cv. Batu 55) ripeness parameters prediction using combined reflectance-fluorescence images and deep convolutional neural network (DCNN) regression model
Scientia Horticulturae(2024)
摘要
This paper presents a novel approach for predicting the ripeness parameters of Mandarin oranges (Citrus reticulata Blanco cv. Batu 55) using a Deep Convolutional Neural Network (DCNN) regression model. The proposed method leverages the combined reflectance-fluorescence images of the fruit. The use of reflectance-fluorescence imaging enables the extraction of more features and information, which contributes to the accurate assessment of fruit ripeness. A comparison between the prediction model using a single Red-Green-Blue (RGB) image from both measurement modes and a combination image from both modes has been made. CNN regression model images with XSE_ResNet50 architecture to predict soluble solid content (SSC), acidity, firmness, and brix-acid ratio. Experimental results demonstrate that the fluorescence image (3 channels input) and the combined reflectance-fluorescence image (6 channels as input) accurately predict maturity parameters. The fluorescence image combined with DCNN performed well in predicting acidity and brix-acid ratio with R2 training/testing of 0.83/0.78 and 0.94/0.88 consecutively. For the SSC and firmness prediction, the reflectance-fluorescence (6 channels) images provide the best accuracy with R2 testing of 0.86/0.81 and 0.91/0.84. This accuracy is higher compared to the conventional image features-based model. Overall, these results show that reflectance-fluorescence images in a visible wavelength regime combined with the DCNN model could enhance the prediction accuracy of citrus maturity parameters.
更多查看译文
关键词
Machine vision,Reflectance-fluorescence,Six-channels,CNN regression,Ripeness
AI 理解论文
溯源树
样例
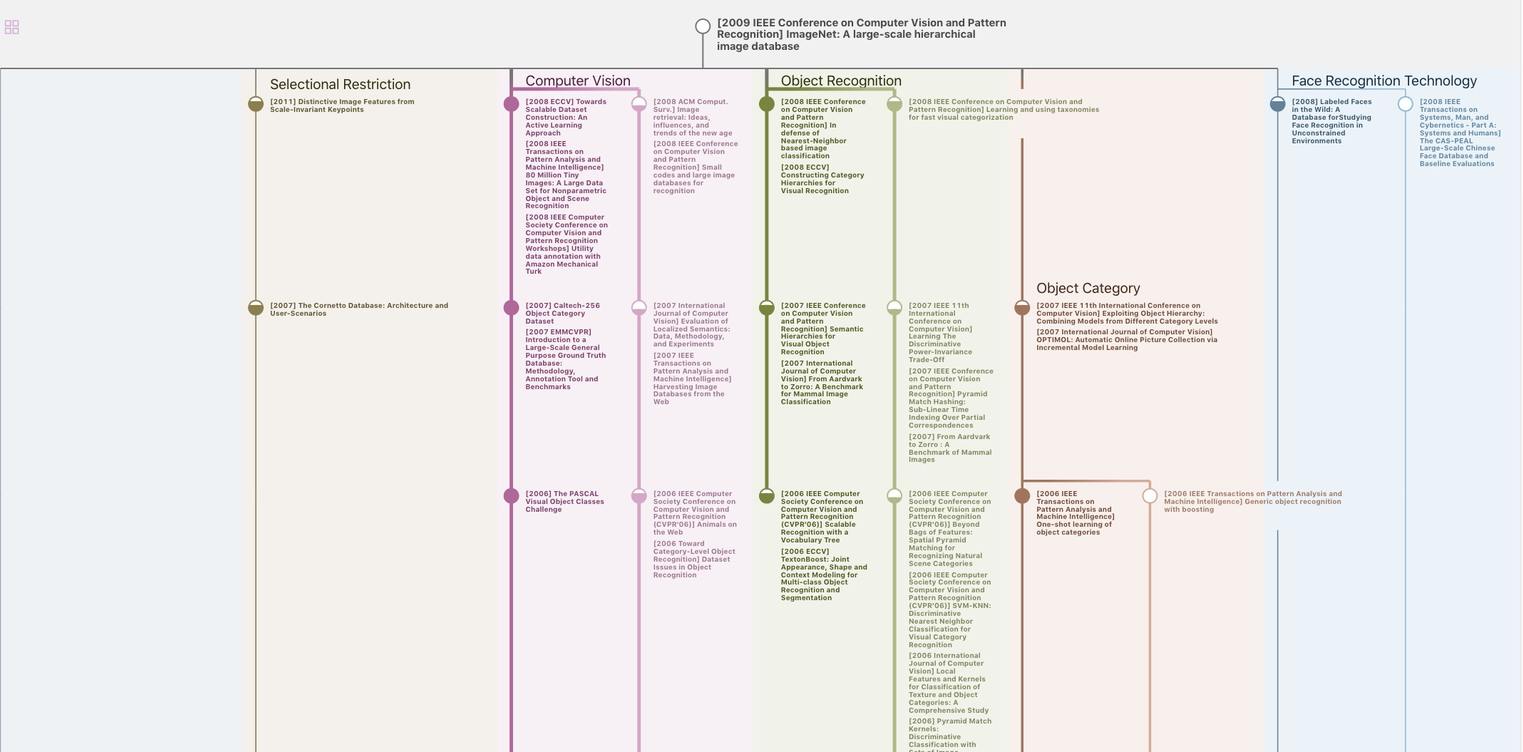
生成溯源树,研究论文发展脉络
Chat Paper
正在生成论文摘要