Eigendecomposition-Based Spatial-Temporal Attention for Brain Cognitive States Identification
ICASSP 2024 - 2024 IEEE International Conference on Acoustics, Speech and Signal Processing (ICASSP)(2024)
摘要
Functional magnetic resonance imaging (fMRI) leverages the blood-oxygen-level-dependent (BOLD) signals to gauge functional brain activation. Specifically, task-fMRI has become a predominant tool to investigate specific cerebral regions associated with diverse cognitive processes. A myriad of studies employing task-fMRI have harnessed both static functional connectivity (FC) and dynamic functional connectivity (dFC) to identify task-related biomarkers. However, while FC and dFC have proven their efficacy, they mostly necessitate manual determination of factors, including window size, stride, and FC metrics, which potentially undermine their analysis reliability. In response to these challenges, one can detect and classify task-related patterns directly from the fMRI signals without using connectivity matrices (i.e., FC and dFC). Here, we propose a novel eigendecomposition-based attention mechanism (EAM) that emphasizes task-related regions and time points in the original signal space. By virtue of this approach, our proposed model allow us to effectively extract a refined integrated feature representation for identifying the brain cognitive states from the fMRI signals and also lessens the burden of making heuristic decisions in measuring both FC and dFC. We validate the superiority and effectiveness of our proposed method with comprehensive evaluations conducted on the Human Connectome Project (HCP) dataset, which covers seven distinct cognitive tasks.
更多查看译文
关键词
Task-fMRI,Eigendecomposition,Attention,Human Connectome Project
AI 理解论文
溯源树
样例
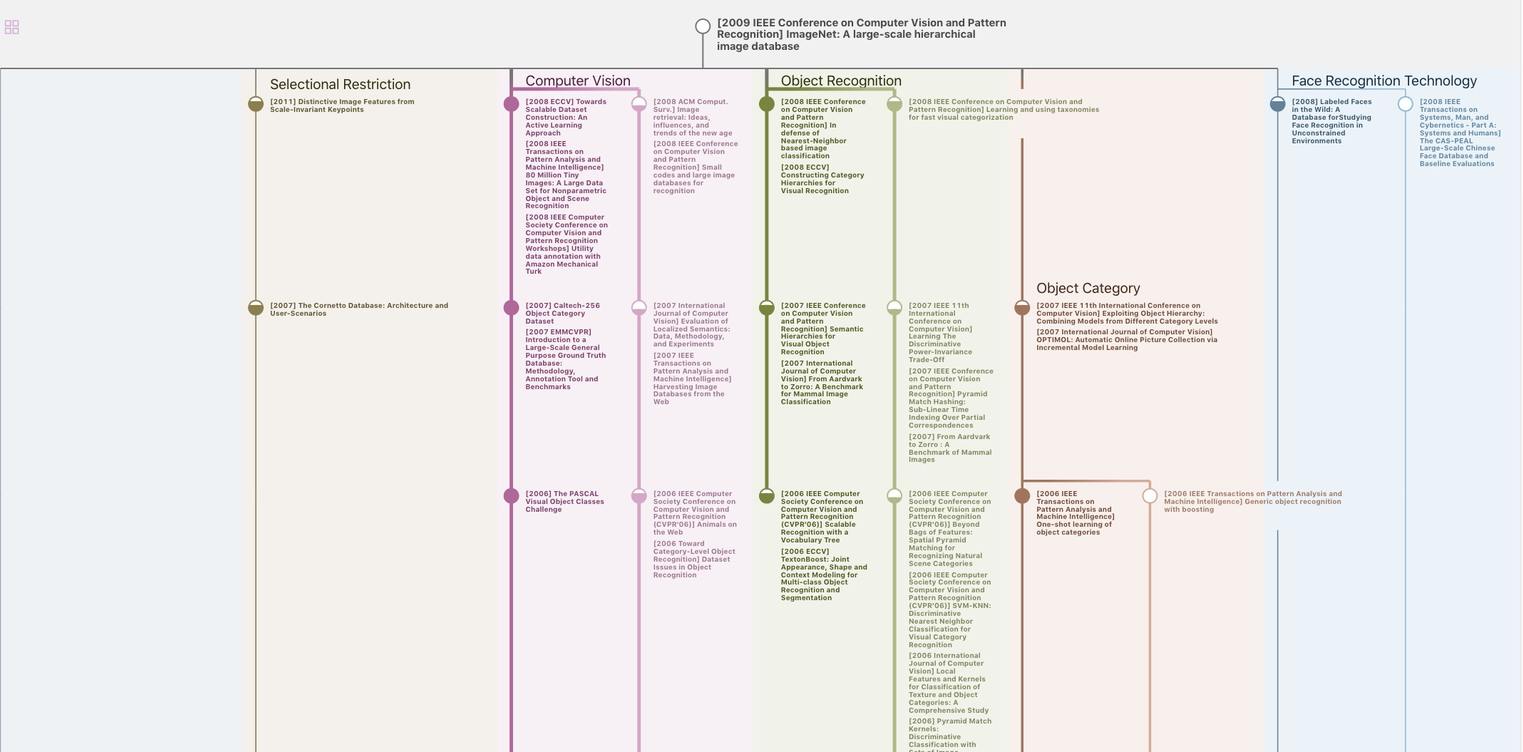
生成溯源树,研究论文发展脉络
Chat Paper
正在生成论文摘要