Pmmwdeconv: Unsupervised Data-Consistent Blind Passive Millimeterwave Image Deconvolution with Global Context Priors
ICASSP 2024 - 2024 IEEE International Conference on Acoustics, Speech and Signal Processing (ICASSP)(2024)
摘要
Passive millimeter-wave (PMMW) imaging is extensively employed in public security industries due to its privacy-safe and non-hazardous nature. Nevertheless, the quality of PMMW images is typically poor given blur and noise. Although the end-to-end learning-based image deconvolution methods have demonstrated promising results, they are highly sensitive to minor variations during tests, making them fail to recover small yet crucial details. On the other hand, the blur kernel estimation from textureless PMMW images is also challenging, since the mainstream methods usually adopt convolutional neural networks (CNNs) with local receptive fields to estimate a blur kernel adapted to the entire image. To this end, we propose a dataset-free method (PMMWDeconv) for blind PMMW image deconvolution by integrating the physical generative model into the deconvolution process. The proposed method mainly contains two subnetworks, kernel generator and image generator, and we leverage data consistency and global context priors to help the network in learning from the blurry PMMW image, where the post-processing and transformer are employed to fulfill these goals. Comprehensive experiments are performed to validate the performance of PMMWDeconv, and the results demonstrate that the proposed method surpasses state-of-the-art methods in both robustness and generalization.
更多查看译文
关键词
Image deconvolution,passive millimeter-wave image,neural network,deep image prior
AI 理解论文
溯源树
样例
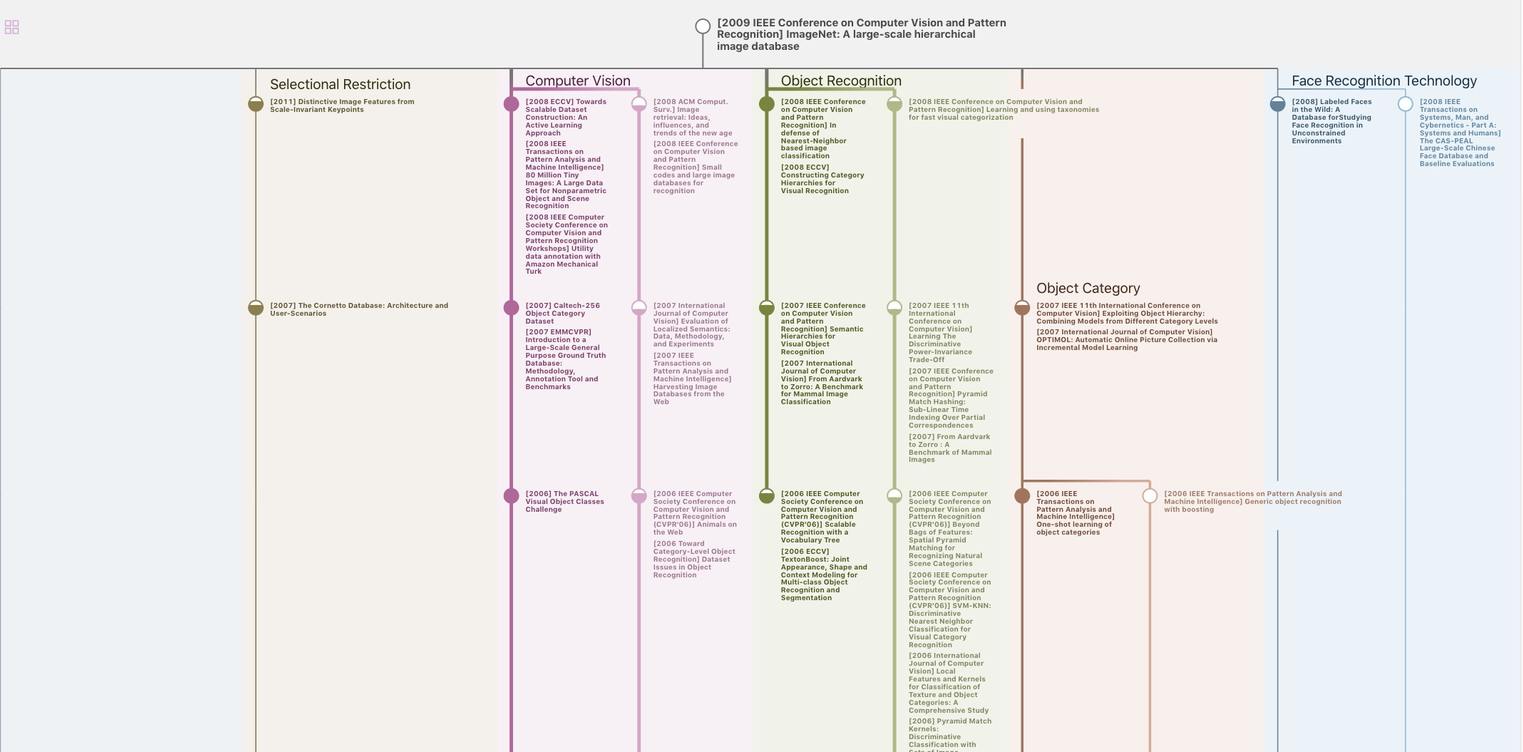
生成溯源树,研究论文发展脉络
Chat Paper
正在生成论文摘要