Domaindiff: Boost out-of-Distribution Generalization with Synthetic Data
ICASSP 2024 - 2024 IEEE International Conference on Acoustics, Speech and Signal Processing (ICASSP)(2024)
摘要
In contemporary machine learning, enhancing model generalization through diversified datasets is essential. Yet, collecting additional data often faces prohibitive costs and privacy constraints, with no guarantee of improved diversity. In this paper, we propose Domain-Diff, featuring a pivotal Word-to-Image Mapping (WIM) mechanism. WIM constructs precise mapping between prompts and images, where the prompts only comprise style and class words. It generates intra-domain data by employing identical prompts to produce source-style images, preserving style and class consistency, thereby diversifying the dataset. Expanding on this innovation, we fuse multiple WIMs and use the prompts with multiple style words to create inter-domain data, which captures a fusion style of multiple source domains. Inter-domain data significantly widens the training data distribution, amplifying diversity. Experimental results demonstrate DomainDiff’s transformative potential, improving model performance on real-world data compared to using only real data. These findings highlight DomainDiff’s utility in enhancing generalization across diverse real-world scenarios.
更多查看译文
关键词
Domain generalization,image generation,data distribution shift,model robustness
AI 理解论文
溯源树
样例
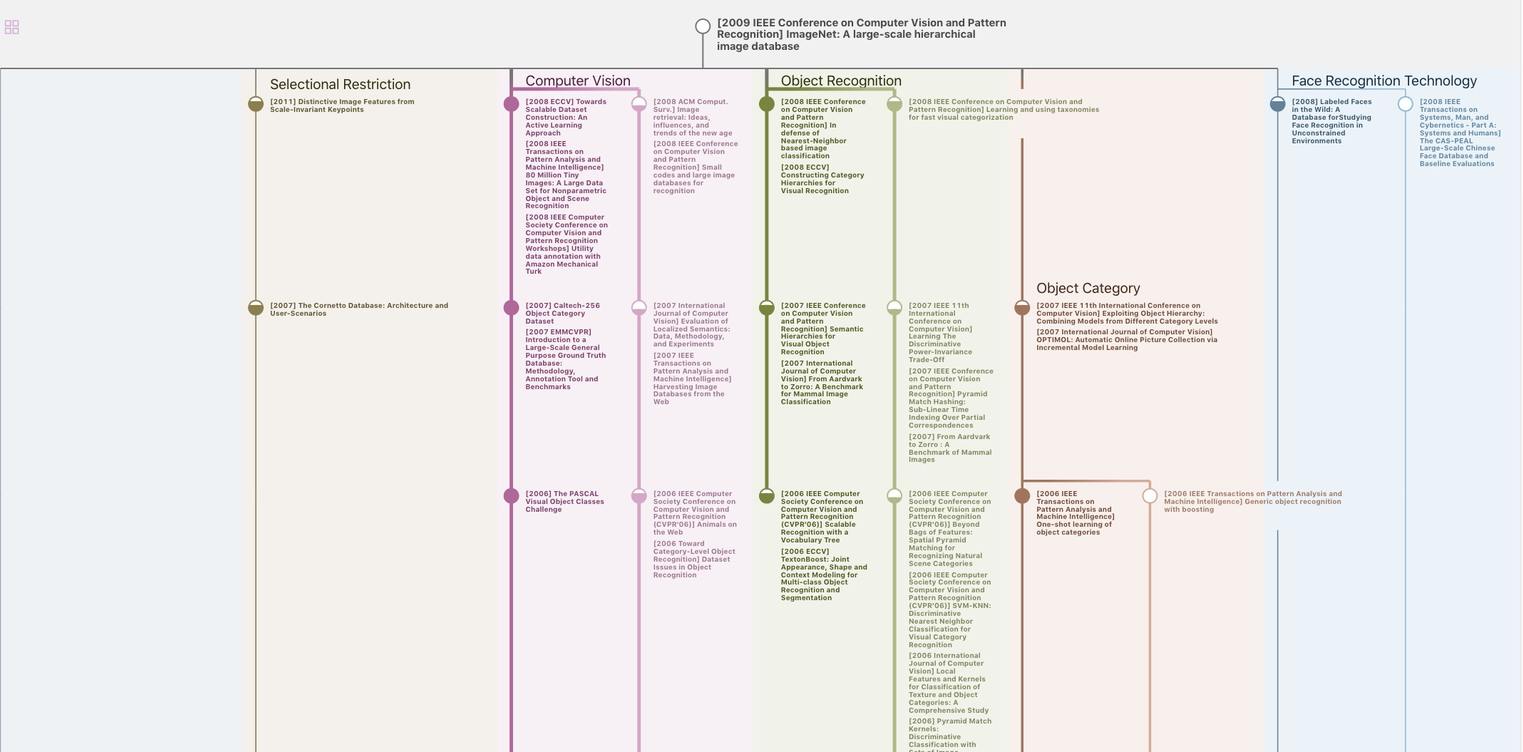
生成溯源树,研究论文发展脉络
Chat Paper
正在生成论文摘要