Class-wise Contrastive Prototype Learning for Semi-Supervised Classification under Intersectional Class Mismatch
IEEE Transactions on Multimedia(2024)
摘要
Traditional Semi-Supervised Learning (SSL) classification methods focus on leveraging unlabeled data to improve the model performance under the setting where labeled set and unlabeled set share the same classes. Nevertheless, the above-mentioned setting is often inconsistent with many real-world circumstances. Practically, both the labeled set and unlabeled set often hold some individual classes, leading to an intersectional class-mismatch setting for SSL. Under this setting, existing SSL methods are often subject to performance degradation attributed to these individual classes. To solve the problem, we propose a Class-wise Contrastive Prototype Learning (CCPL) framework, which can properly utilize the unlabeled data to improve the SSL classification performance. Specifically, we employ a supervised prototype learning strategy and a class-wise contrastive separation strategy to construct a prototype for each known class. To reduce the influence of the individual classes in unlabeled set (i.e., out-of-distribution classes), each unlabeled example can be weighted reasonably based on the prototypes during classifier training, which helps to weaken the negative influence caused by out-of-distribution classes. To reduce the influence of the individual classes in labeled set (i.e., private classes), we present a private assignment suppression strategy to suppress the improper assignments of unlabeled examples to the private classes with the help of the prototypes. Experimental results on four benchmarks and one real-world dataset show that our CCPL has a clear advantage over fourteen representative SSL methods as well as two supervised learning methods under the intersectional class-mismatch setting.
更多查看译文
关键词
Semi-supervised learning,intersectional class mismatch,prototype learning,contrastive learning,private assignment suppression
AI 理解论文
溯源树
样例
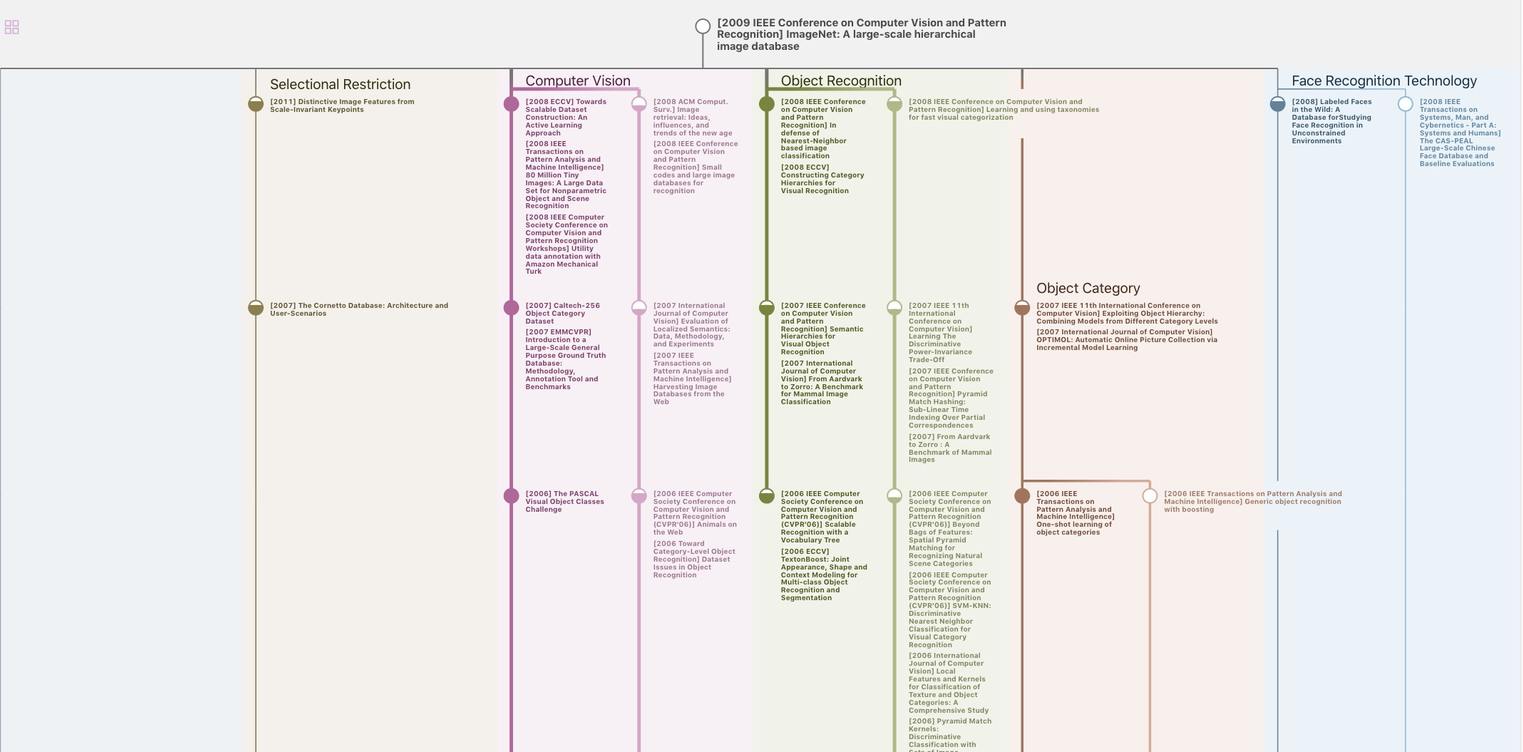
生成溯源树,研究论文发展脉络
Chat Paper
正在生成论文摘要