Inference of Genetic Effects via Approximate Message Passing
ICASSP 2024 - 2024 IEEE International Conference on Acoustics, Speech and Signal Processing (ICASSP)(2024)
摘要
Efficient utilization of large-scale biobank data is crucial for inferring the genetic basis of disease and predicting health outcomes from the DNA. Yet we lack efficient, accurate methods that scale to data where electronic health records are linked to whole genome sequence information. To address this issue, our paper develops a new algorithmic paradigm based on Approximate Message Passing (AMP), which is specifically tailored for genomic prediction and association testing. Our method yields comparable out-of-sample prediction accuracy to the state of the art on UK Biobank traits, whilst dramatically improving computational complexity, with a 8x-speed up in the run time. In addition, AMP theory provides a joint association testing framework, which outperforms the currently used REGENIE method, in roughly a third of the compute time. This first, truly large-scale application of the AMP framework lays the foundations for a far wider range of statistical analyses for hundreds of millions of variables measured on millions of people.
更多查看译文
关键词
Genome-wide association studies,Approximate message passing,High-dimensional inference,Expectation-maximization
AI 理解论文
溯源树
样例
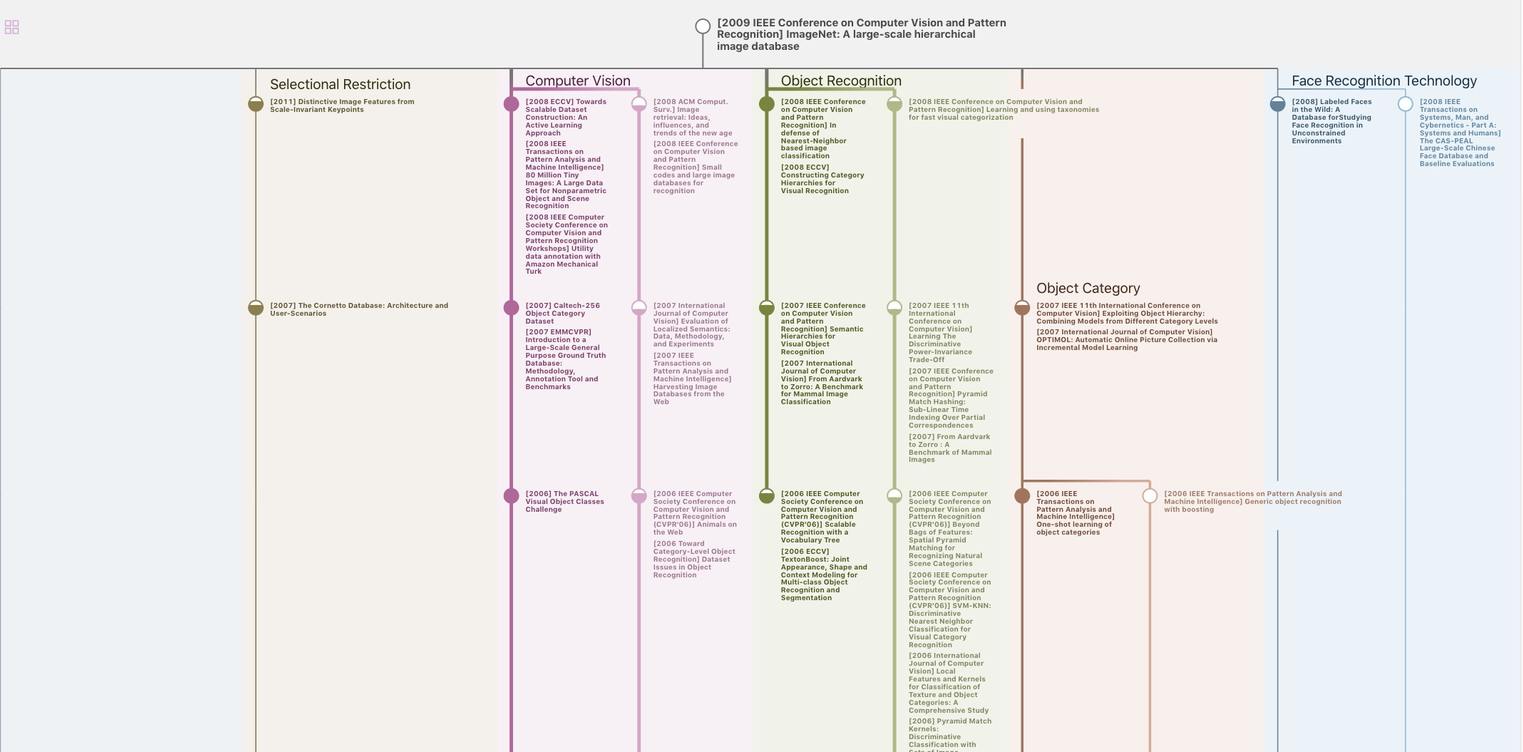
生成溯源树,研究论文发展脉络
Chat Paper
正在生成论文摘要