BitFormer: Transformer-Based Neural Network for Bitrate Prediction in Real-Time Communications.
Consumer Communications and Networking Conference(2024)
摘要
In recent years, an exponential upsurge in the global proliferation of Real-Time Communications (RTC) applications has been witnessed, due to the prosperous development of networks and further fueled by the ramifications of the COVID-19 pandemic. Consequently, the imperative for development of intelligent, resilient, and scalable network infrastructures and technologies has grown significantly. Real-time bitrate prediction could play a crucial role, offering network observability and bolstering proactive system management. By accurately forecasting bitrate, it becomes possible to implement improvements at either application level or network level, such as swift and appropriate bandwidth adaptation. In this paper, we propose a novel Transformer-based deep learning framework called BitFormer designed to predict the short-term bitrate. Our work is based on extensive traffic data collected under various conditions using two prevalent RTC applications, and our model relies solely on packet-level information, which contains the fundamental traffic characteristics and facilitates effortless feature extraction. Through comprehensive evaluations and comparisons, we achieve a superior accuracy of 74% in identifying peak bitrates, while simultaneously ensuring commendable overall performance.
更多查看译文
关键词
Networking,packet level,Real-time communications,RTP,machine learning,deep learning
AI 理解论文
溯源树
样例
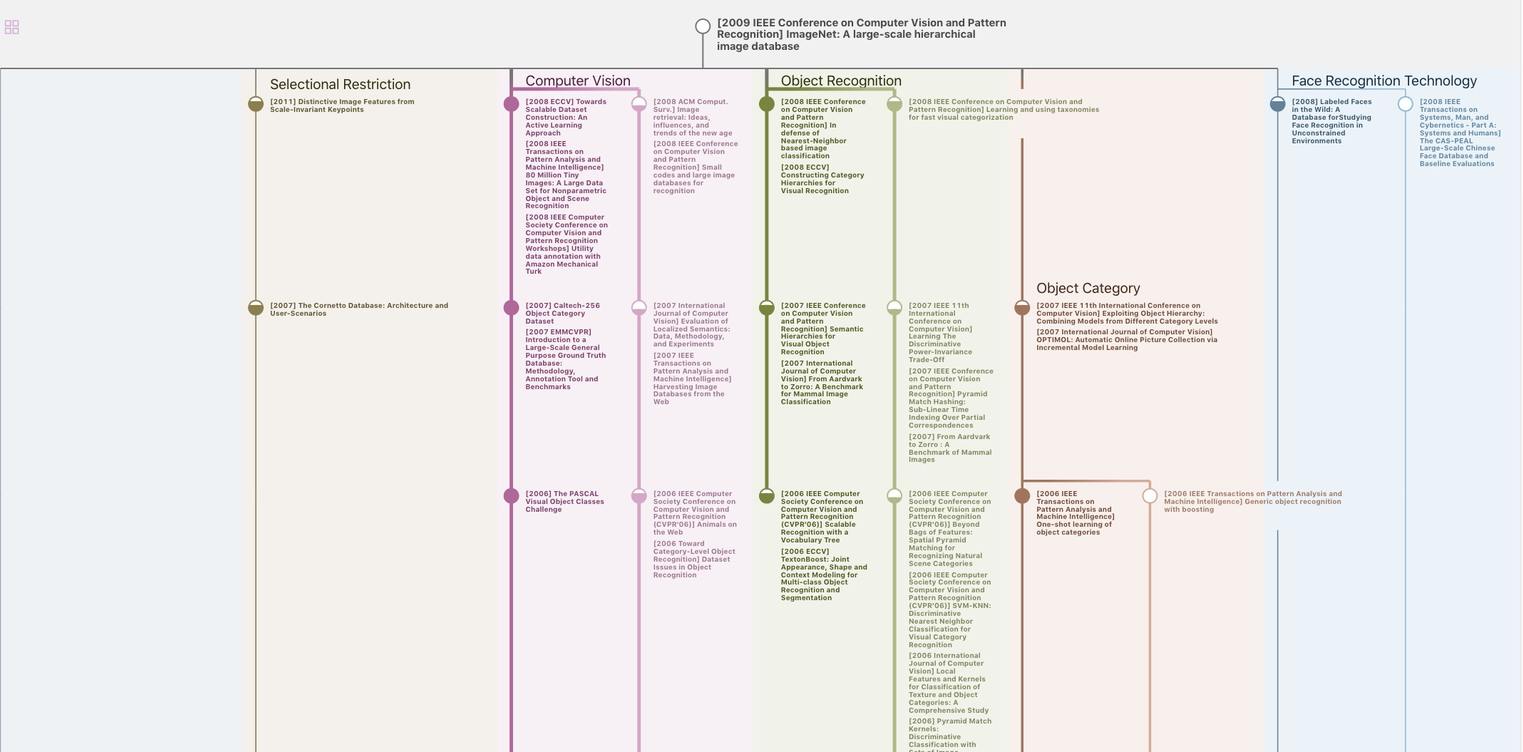
生成溯源树,研究论文发展脉络
Chat Paper
正在生成论文摘要