Enhancing Short-and Long-Term Sea Surface Temperature Forecasting with a Static and Dynamic Learnable Personalized Graph Convolution Network
ICASSP 2024 - 2024 IEEE International Conference on Acoustics, Speech and Signal Processing (ICASSP)(2024)
摘要
Sea surface temperature (SST) plays an important role in our Earth’s atmosphere, wielding significant influence over both local and global climates and profoundly impacting ecosystems. However, this task presents unique challenges due to the inherent complexity and uncertainty within ocean systems. Recently, deep learning techniques, such as Graph Neural Networks (GNNs), have been employed to address SST forecasting. These methods often grapple with substantial limitations in capturing dynamic spatiotemporal relationships between signals. To tackle this issue, this paper introduces the Static and Dynamic Learnable Personalized Graph Convolution Network (SD-LPGC). This innovative approach comprises two distinct graph learning layers designed to model both stable long-term and short-term evolutionary patterns within multivariate SST signals. Additionally, a learnable personalized convolution layer is integrated to fuse these insights. Our experiments, conducted on real SST datasets, highlight the state-of-the-art performance of the proposed SD-LPGC approach in the realm of SST forecasting, demonstrating its potential to revolutionize this critical task.
更多查看译文
关键词
Temperature forecasting,CNN,GNN,Personalized convolution,TCN
AI 理解论文
溯源树
样例
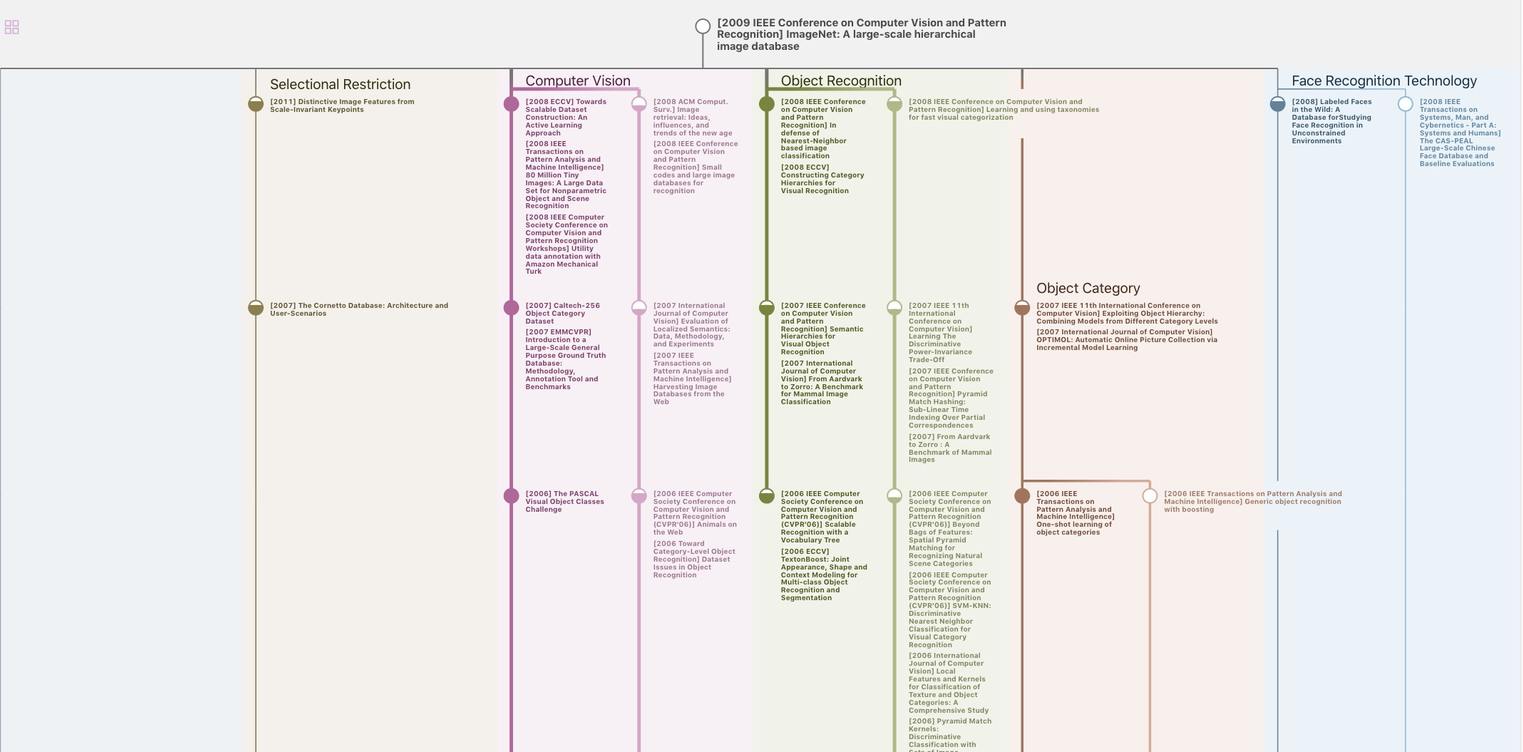
生成溯源树,研究论文发展脉络
Chat Paper
正在生成论文摘要