MADRL-Based Joint Radio Resource Optimization for D2D at the Cell Edge in UDN
2023 5th International Conference on Electronics and Communication Technologies (ECT(2023)
摘要
Ultra-dense network (UDN) effectively reuses spectrum resources by increasing the number of communication links per unit area. It has become an important means to enhance the capacity of next-generation mobile communication systems. Device-to-device (D2D) communication enables adjacent devices to establish communication without resorting to a base station, which further improves spectrum efficiency in UDN scenarios. In this paper, we consider a D2D-enabled UDN scenario where the D2D pair can optimize the performance of the entire network by adjusting its selection of access points, spectrum and transmitting power. Facing the challenging task, we leverage multi-agent deep reinforcement learning (MADRL) and propose the MADRL joint optimization (MADRJO) algorithm to dynamically solve the problem. In addition, we introduce the self-attention mechanism and the multi-environment parallel training mechanism to optimize the training efficiency. The performance of the MADRJO algorithm in the simulation experiment proves that it can effectively optimize the average information rate and QoS metrics in the communication network, and can well adapt to the changes of the network environment.
更多查看译文
关键词
multi-agent deep reinforcement learning (MADRL),ultra-dense network (UDN),device-to-device (D2D),user association,spectrum allocation,power control,heterogeneous network
AI 理解论文
溯源树
样例
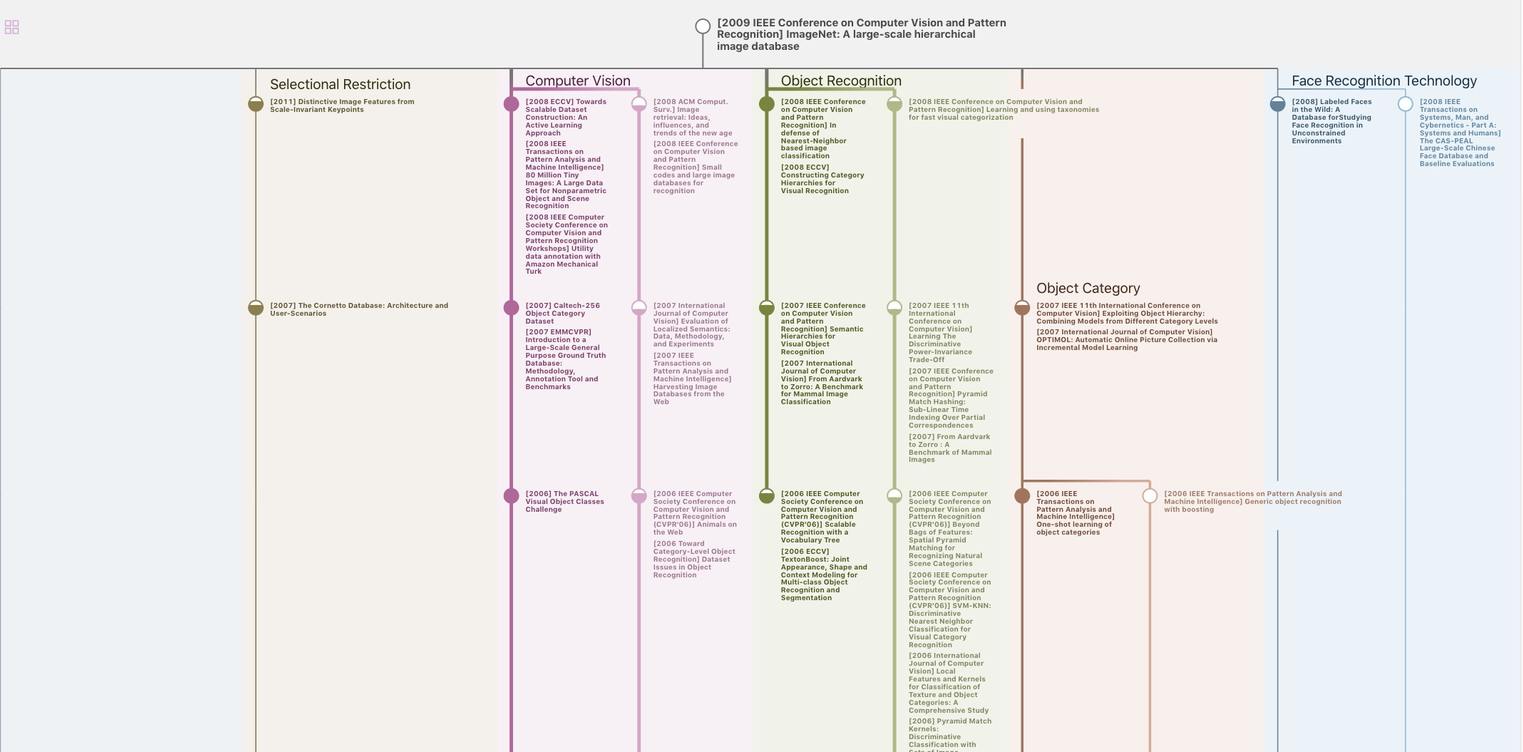
生成溯源树,研究论文发展脉络
Chat Paper
正在生成论文摘要