NAIR: An Efficient Distributed Deep Learning Architecture for Resource Constrained IoT System
IEEE Internet of Things Journal(2024)
摘要
The distributed deep learning architecture can support the front-deployment of deep learning systems in resource constrained IoT devices and is attracting increasing interest. However, most ready-to-use deep models are designed for centralized deployment without considering the transmission loss of the intermediate representation inside the distributed architecture. This oversight significantly affects the inference performance of distributed deployed deep models. To alleviate this problem, a state-of-the-art work chooses to retrain the original model to form an intermediate representation with ordered importance and yields better inference accuracy under constrained transmission bandwidth. This paper first reveals that this solution is essentially a pruning-like solution, where unimportant information is adaptively pruned to fit within the limited bandwidth. With this understanding, a novel scheme named Naturally Aggregated Intermediate Representation (NAIR) has been proposed, which aims to naturally amplify the difference of importance embedded in the intermediate representation from a mature deep model and reassemble the intermediate representation into a hierarchy of importance from high-to-low to accommodate the transmission loss. As a result, this method shows further improved performance in various scenarios, avoids compromising the overall inference performance of the system, and saves astronomical retraining and storage costs. The effectiveness of NAIR has been validated through extensive experiments, achieving a 112% improvement in performance compared to the state-of-the-art work.
更多查看译文
关键词
Distributed Deep Learning,Convolutional Neural Network,Pruning,Transmission Loss
AI 理解论文
溯源树
样例
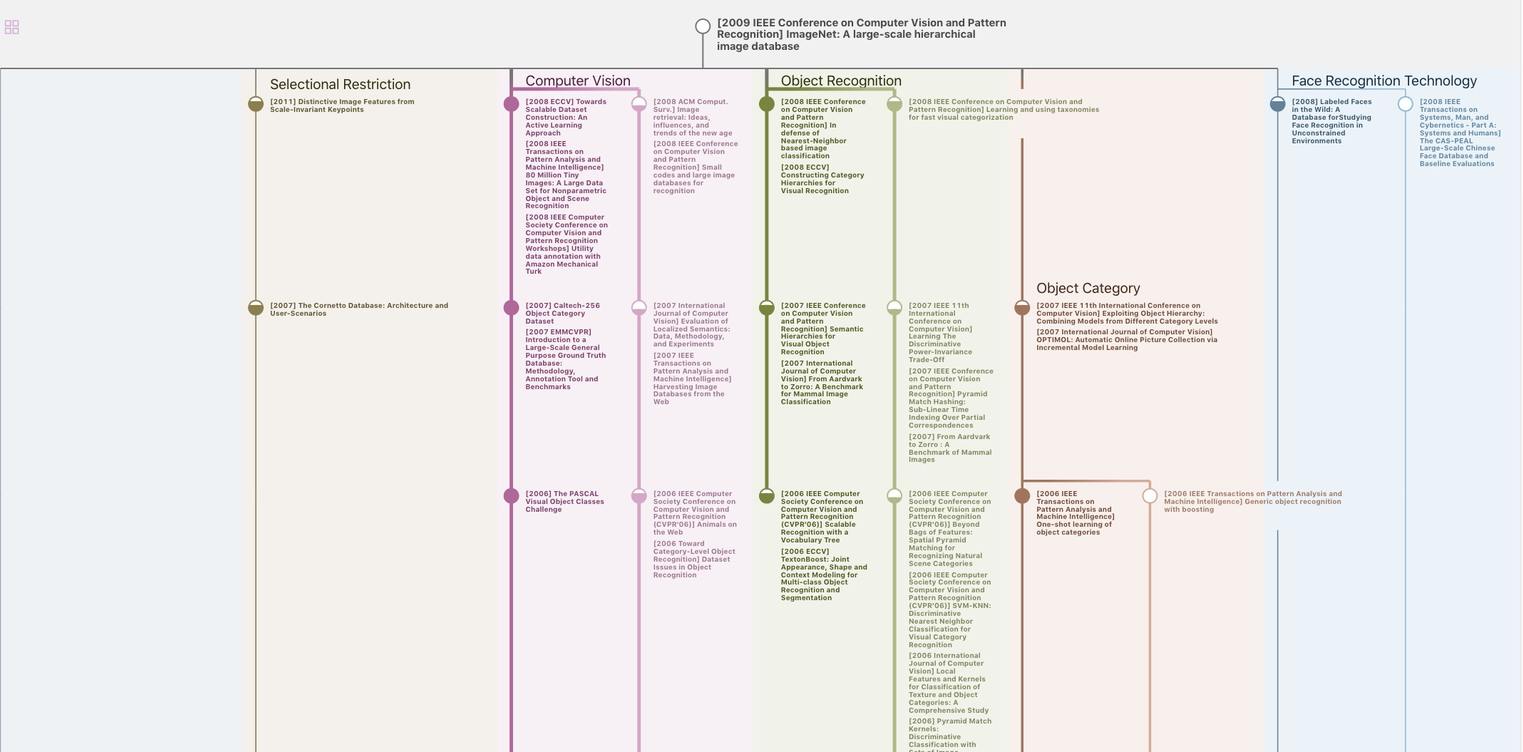
生成溯源树,研究论文发展脉络
Chat Paper
正在生成论文摘要