Boosting Pruned Networks with Linear Over-Parameterization
IEEE International Conference on Acoustics, Speech, and Signal Processing(2024)
Key words
structured pruning,fine-tuning,over-parameterization,knowledge distillation
AI Read Science
Must-Reading Tree
Example
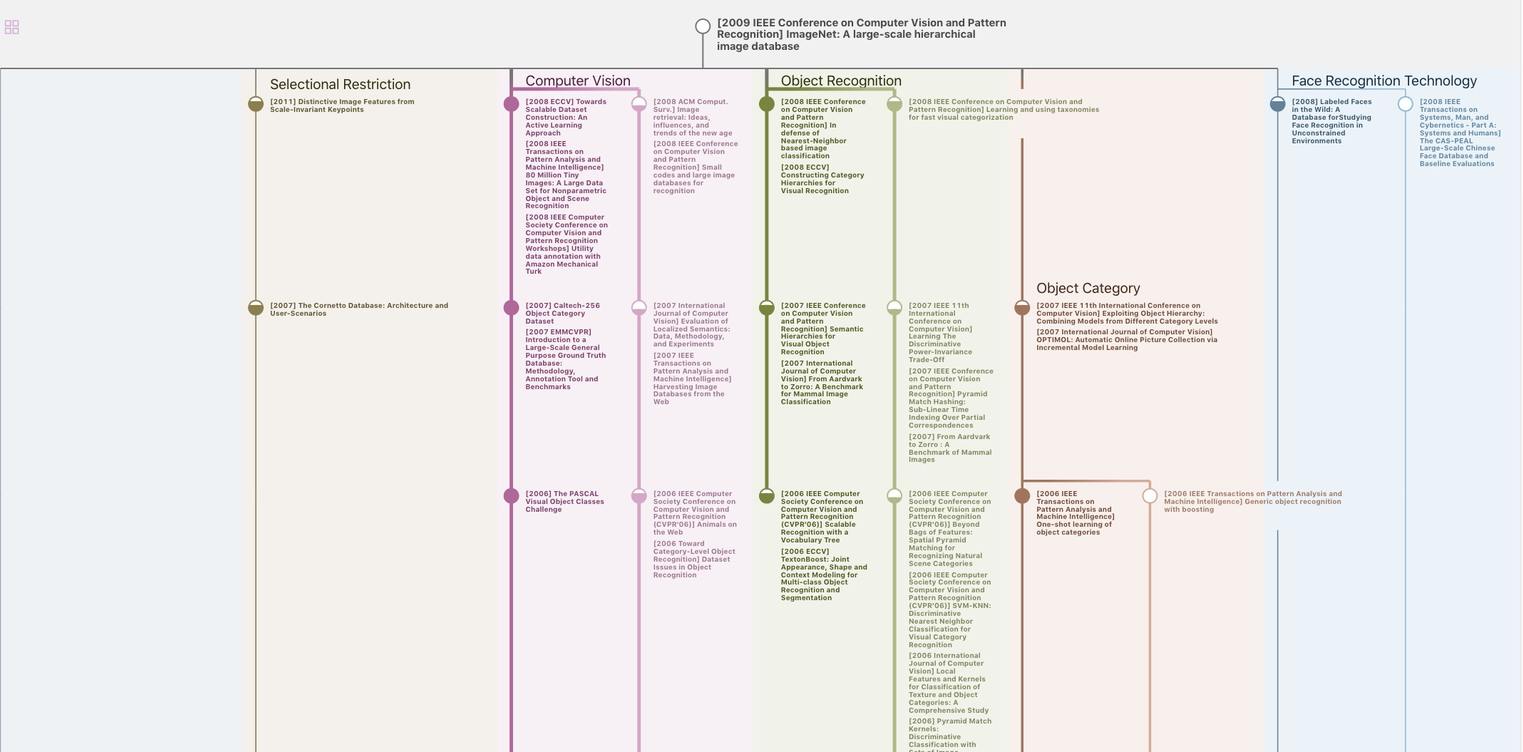
Generate MRT to find the research sequence of this paper
Chat Paper
Summary is being generated by the instructions you defined