Reinforcement Learning and Transformer for Fast Magnetic Resonance Imaging Scan
IEEE Transactions on Emerging Topics in Computational Intelligence(2024)
摘要
A major drawback in Magnetic Resonance Imaging (MRI) is the long scan times necessary to acquire complete K-space matrices using phase encoding. This paper proposes a transformer-based deep Reinforcement Learning (RL) framework (called TITLE) to reduce the scan time by sequentially selecting partial phases in real-time so that a slice can be accurately reconstructed from the resultant slice-specific incomplete K-space matrix. As a deep learning based slice-specific method, the TITLE method has the following characteristic and merits: (1) It is real-time because the decision of which phase to be encoded in next time can be made within the period between the time at which an echo signal is obtained and the time at which the next 180° RF pulse is activated. (2) It exploits the powerful feature representation ability of transformer, a self-attention based neural network, for predicting phases with the mechanism of deep reinforcement learning. (3) Both historically selected phases (called phase-indicator vector) and the corresponding undersampled image of the slice being scanned are used for extracting features by transformer. Experimental results on the fastMRI dataset demonstrate that the proposed method is 150 times faster than the state-of-the-art reinforcement learning based method and outperforms the state-of-the-art deep learning based methods in reconstruction accuracy. The source codes are available.
更多查看译文
关键词
Scan trajectory,fast MRI,deep reinforcement learning,magnetic resonance imaging,transformer
AI 理解论文
溯源树
样例
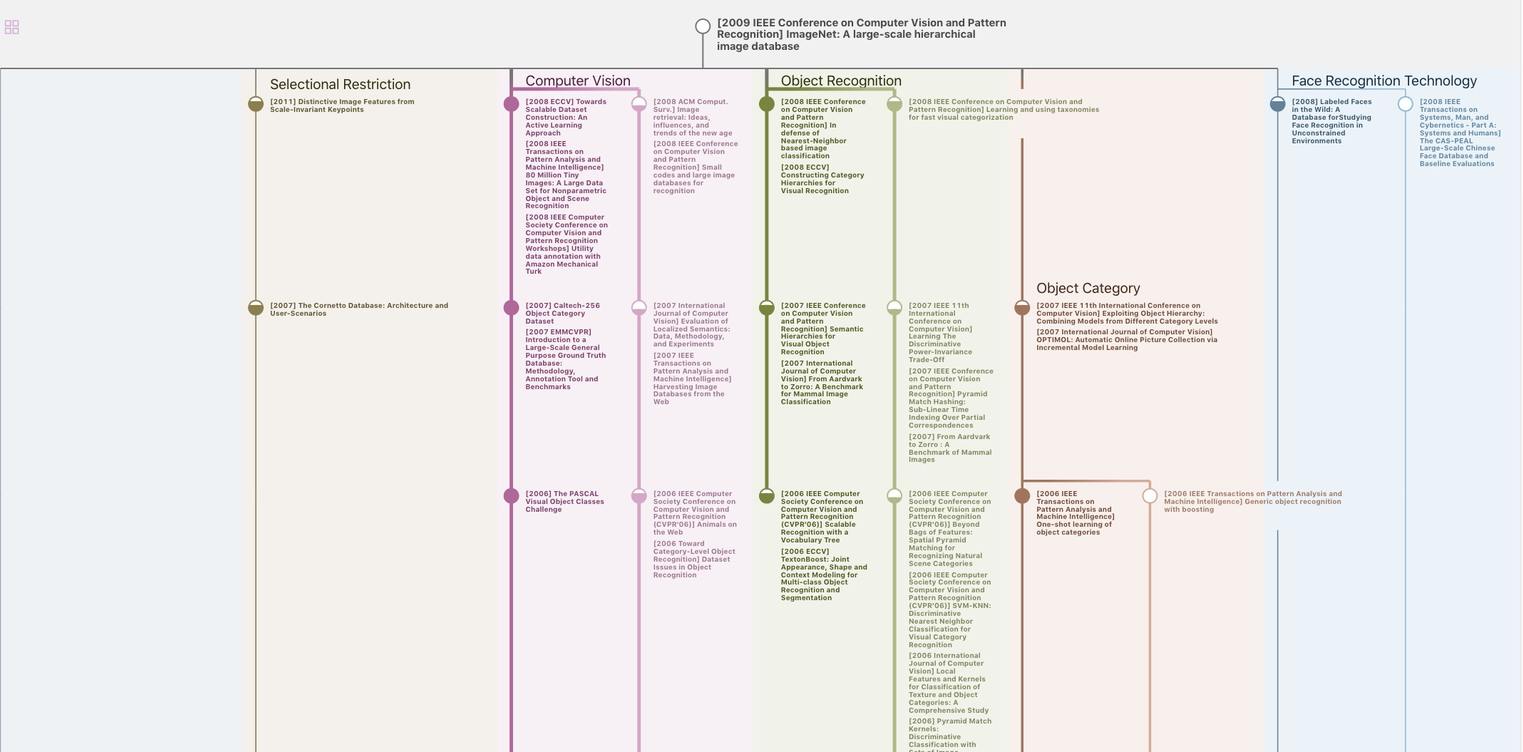
生成溯源树,研究论文发展脉络
Chat Paper
正在生成论文摘要