Out-of-Distribution Detection for Learning-Based Chest X-Ray Diagnosis
ICASSP 2024 - 2024 IEEE International Conference on Acoustics, Speech and Signal Processing (ICASSP)(2024)
摘要
Deep learning has shown prominence in chest radiography interpretation, which is critical in evaluating various lung and chest diseases, such as pneumonia, emphysema, and tuberculosis. Deploying machine learning model, it is important to detect out-of-distribution (OOD) inputs, which are distinct from the training data. For example, a model trained on conventional pneumonia data may not be able to diagnose COVID-19 accurately, letting alone the vast body of possible abnormalities and variation in medical imaging. Thus, a trustworthy diagnosing model should be alerted with such OOD inputs to avoid the overconfidence with samples and therefore the risk of misdiagnosis and severe medical consequences. In this paper, we study the problem of OOD detection for deep learning-based chest X-ray imaging by proposing a distance-based OOD detection method. We show that our method (1) inherits the merits of distance-based methods in being applicable to different models and training losses; and (2) adopts probabilistic score functions with fewer statistics to mitigate the potential distribution assumption on training data. We conduct extensive experiments on benchmarks to demonstrate the superiority of our method. In particular, on challenging tasks, such as Chest X-ray 14 vs. COVID-19, our method achieves 12.62% lower FPR than the state-of-the-art solutions.
更多查看译文
关键词
Out-of-distribution,chest X-ray,deep learning
AI 理解论文
溯源树
样例
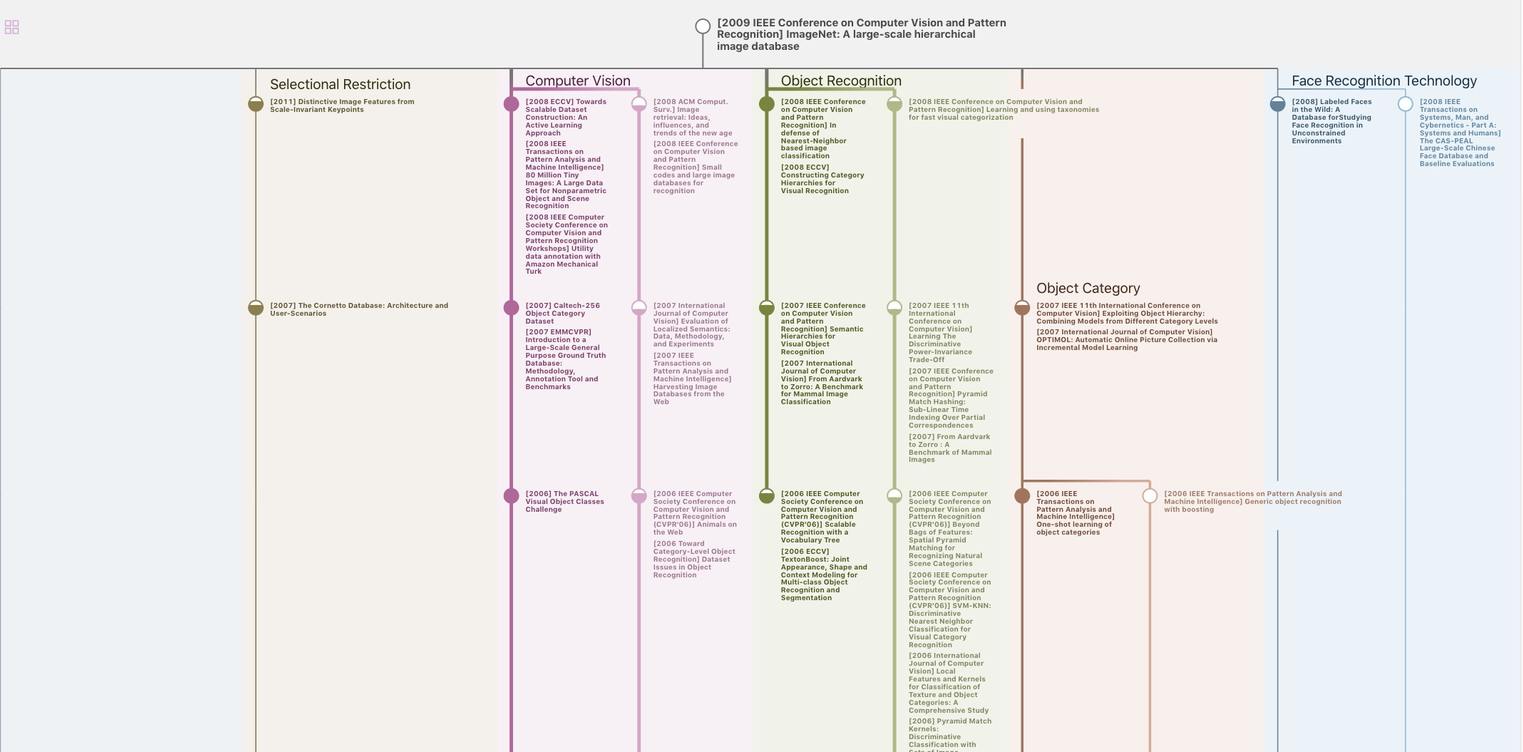
生成溯源树,研究论文发展脉络
Chat Paper
正在生成论文摘要