Unsupervised Continual Learning of Image Representation Via Rememory-Based Simsiam
ICASSP 2024 - 2024 IEEE International Conference on Acoustics, Speech and Signal Processing (ICASSP)(2024)
摘要
Unsupervised continual learning (UCL) of image representation has garnered attention due to practical need. However, recent UCL methods focus on mitigating the catastrophic forgetting with a replay buffer (i.e., rehearsal-based strategy), which needs much extra storage. To overcome this drawback, we propose a novel rememory-based SimSiam (RM-SimSiam) method to reduce the dependency on replay buffer. The core idea of RM-SimSiam is to store and remember the old knowledge with a data-free historical module instead of replay buffer. Specifically, this historical module is designed to store the historical average model of all previous models (the memory process) and then transfer the knowledge of the historical average model to the new model (the rememory process). To further improve the rememory ability of RMSimSiam, we devise an enhanced SimSiam-based contrastive loss by aligning the representations outputted by the historical and new models. Extensive experiments on three benchmarks demonstrate the effectiveness of our RM-SimSiam.
更多查看译文
关键词
Representation learning,Unsupervised continual learning,Rememory,Catastrophic forgetting
AI 理解论文
溯源树
样例
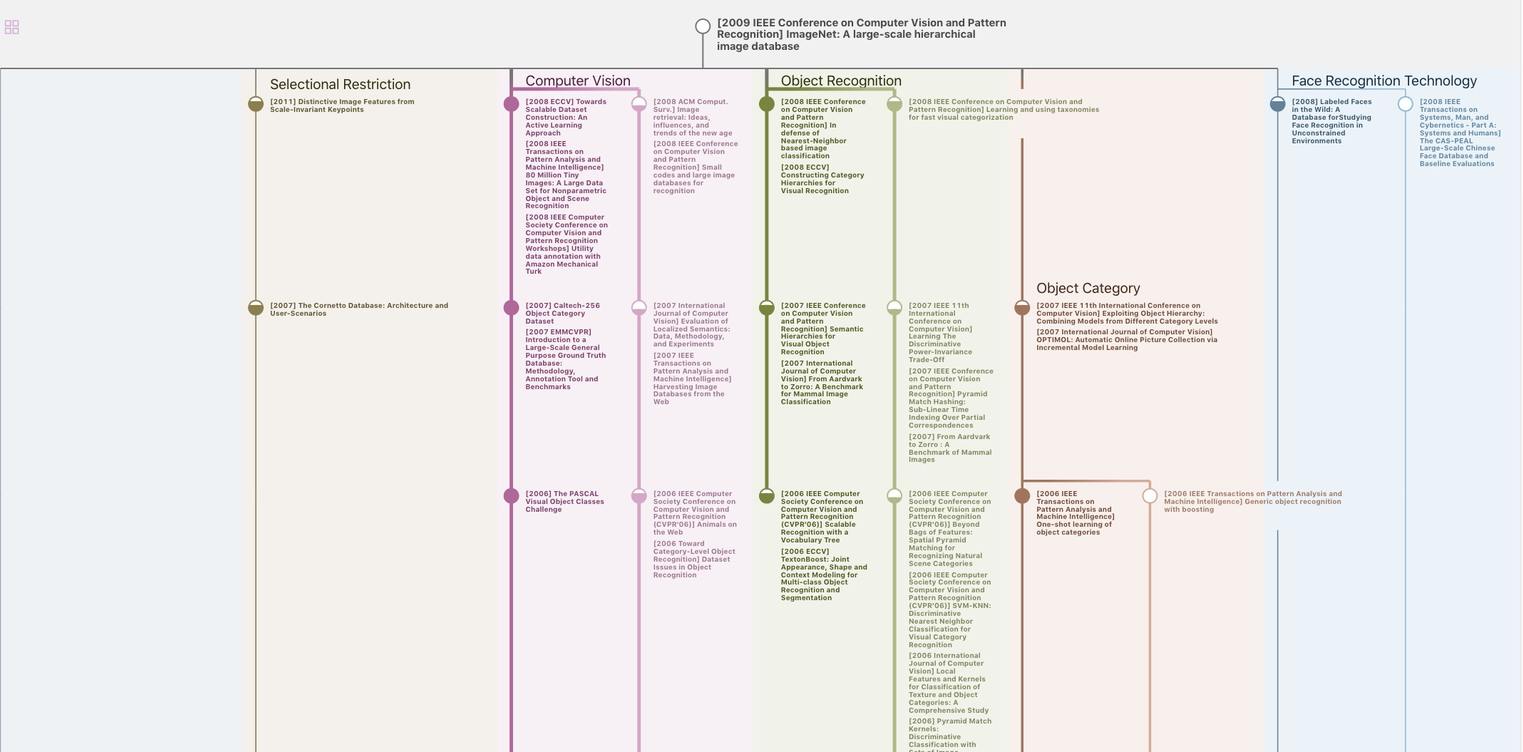
生成溯源树,研究论文发展脉络
Chat Paper
正在生成论文摘要