Dynamic Jamming Power Allocation With Incomplete Sensing Information: Improving by GAN and Opponent Modeling.
IEEE Commun. Lett.(2024)
摘要
This letter studies the problem of dynamic jamming power allocation (JPA) with incomplete sensing information in dynamic and unknown environments. Most existing studies assume that jammers have perfect sensing information, without the consideration of the reliability of sensors, leading to poor performance when facing incomplete sensing information. In response, we propose a robust intelligent JPA scheme and adopt a two-stage algorithm namely “offline training and online deploy" to guide the training and deployment process. The approach utilizes the observed values from spatially distributed sensor units as inputs and employs deep reinforcement learning (DRL) for jamming power decision-making. To handle the missing observation data, we designed a data completion module based on the generative adversarial networks (GAN) framework. In addition, we introduce the priority experience replay mechanism (PERM) and opponent modeling (OM) in the decision model to enhance the learning efficiency and decision accuracy of the network. Simulation results show that the proposed approach can achieve efficient jamming with incomplete information, and outperform conventional DRL-based approaches.
更多查看译文
关键词
Jamming power allocation,incomplete information,deep reinforcement learning,generative adversarial network,opponent modeling
AI 理解论文
溯源树
样例
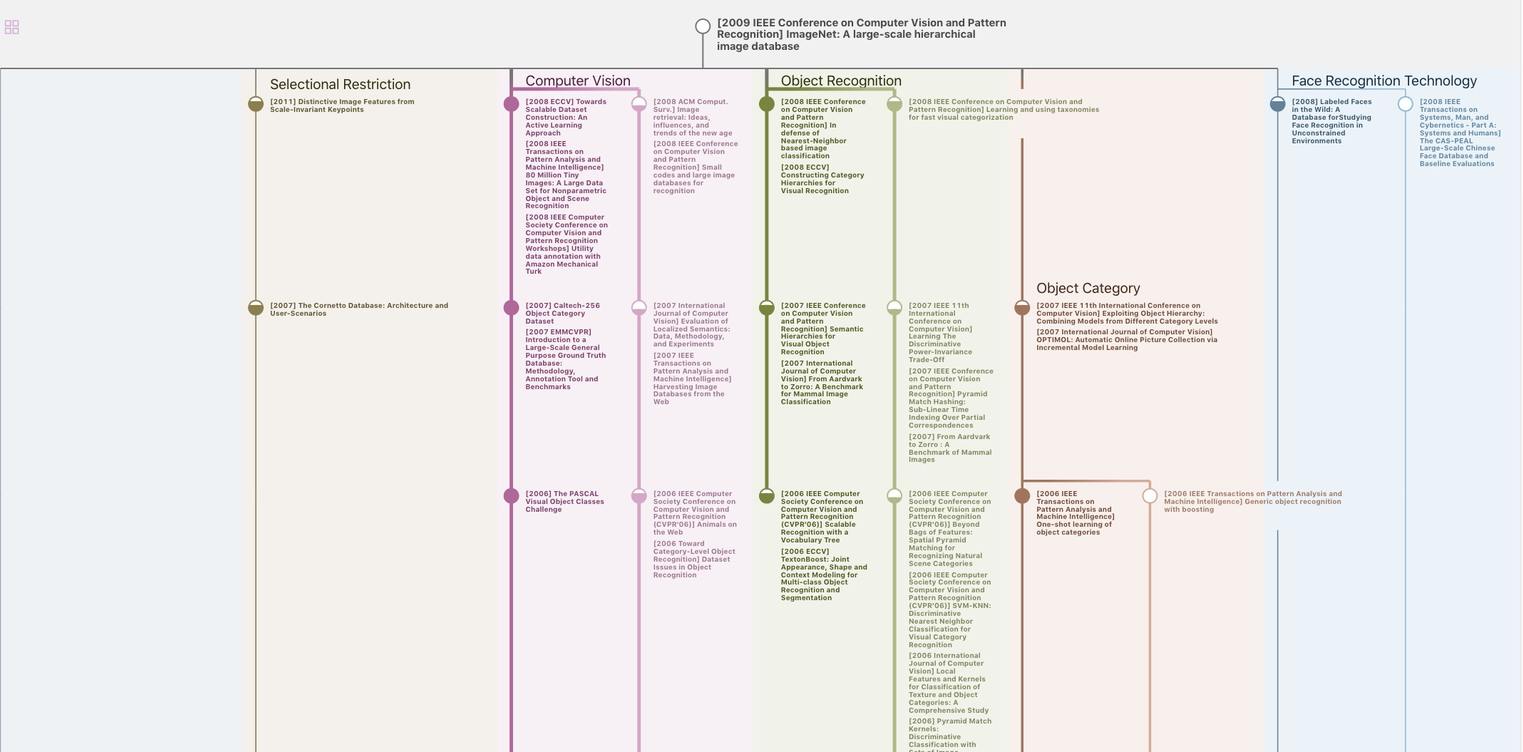
生成溯源树,研究论文发展脉络
Chat Paper
正在生成论文摘要