A Relation-Aware Heterogeneous Graph Transformer on Dynamic Fusion for Multimodal Classification Tasks
ICASSP 2024 - 2024 IEEE International Conference on Acoustics, Speech and Signal Processing (ICASSP)(2024)
摘要
Multimodal fusion aims to improve the performance of models for applications by extracting and fusing information in different modalities, including texts, images or others. Recent researches have shown that multimodal fusion is beneficial in many multimedia tasks. In this paper, we study typical multimedia classification tasks in social media posts, including sarcasm detection and sentiment analysis. This paper proposes DMF-RHGT-HPA, including dynamic Fusion multimodal fusion(DMF), a relation-aware heterogeneous graph transformer(RHGT) and hierarchical pooling alignment(HPA). To realize better multimodal fusion, the paper designs it on a heterogeneous graph with dynamic links, without any padding of texts or images. To thoroughly learn the multimodal graph and obtain the representation of nodes, the paper proposes a relation-aware heterogeneous graph transformer to fuse the node-level and edge-level features simultaneously. To get a refined representation of the multimodal graph, the paper designs a hierarchical pooling alignment to gather all nodes’ representations well. Experiments conducted on two primary and public datasets from Twitter and Yelp respectively show the ability of DMF-RHGT-HPA to gain the best performance of sarcasm detection and sentiment analysis, outperforming existing state-of-the-art baselines.
更多查看译文
关键词
Dynamic Fusion,Relation-Aware Graph Transformer,Hierarchical Pooling Alignment,Graph Classification
AI 理解论文
溯源树
样例
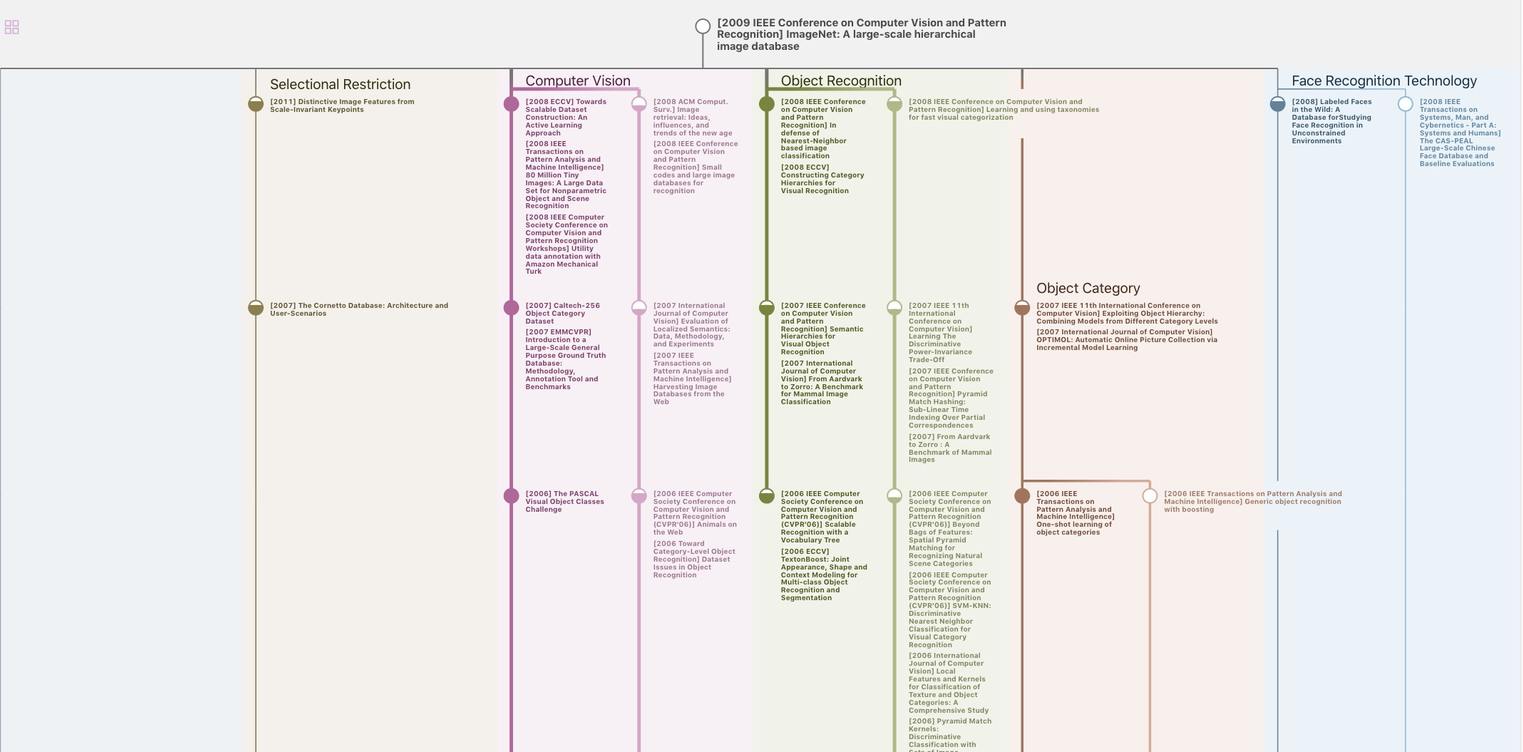
生成溯源树,研究论文发展脉络
Chat Paper
正在生成论文摘要