X-CAUNET: Cross-Color Channel Attention with Underwater Image-Enhancing Transformer
ICASSP 2024 - 2024 IEEE International Conference on Acoustics, Speech and Signal Processing (ICASSP)(2024)
摘要
Underwater image enhancement is essential to mitigate the environment-centric noise in images, such as haziness, color degradation, etc. With most existing works focused on processing an RGB image as a whole, the explicit context that can be mined from each color channel separately goes unaccounted for, ignoring the effects produced by the wavelength of light in underwater conditions. In this work, we propose a framework called X-CAUNET that addresses this research gap by using cross-attention transformers. The input image is split into three channels (R-G-B), local context is captured using convolutional layers with different receptive field sizes, and a message-passing mechanism allows for context correlation between them. To maintain consistency, another transformer is used on the original image to aggregate global context, and a weighted combination of all the outputs enhances the input degraded image. Extensive experiments demonstrate we achieve state-of-the-art PSNR and SSIM with 2.66% and 2.11% relative gains. Code is available at: https://github.com/Alik033/X-CAUNET.
更多查看译文
关键词
Cross-attention,transformer,underwater image enhancement,deep learning
AI 理解论文
溯源树
样例
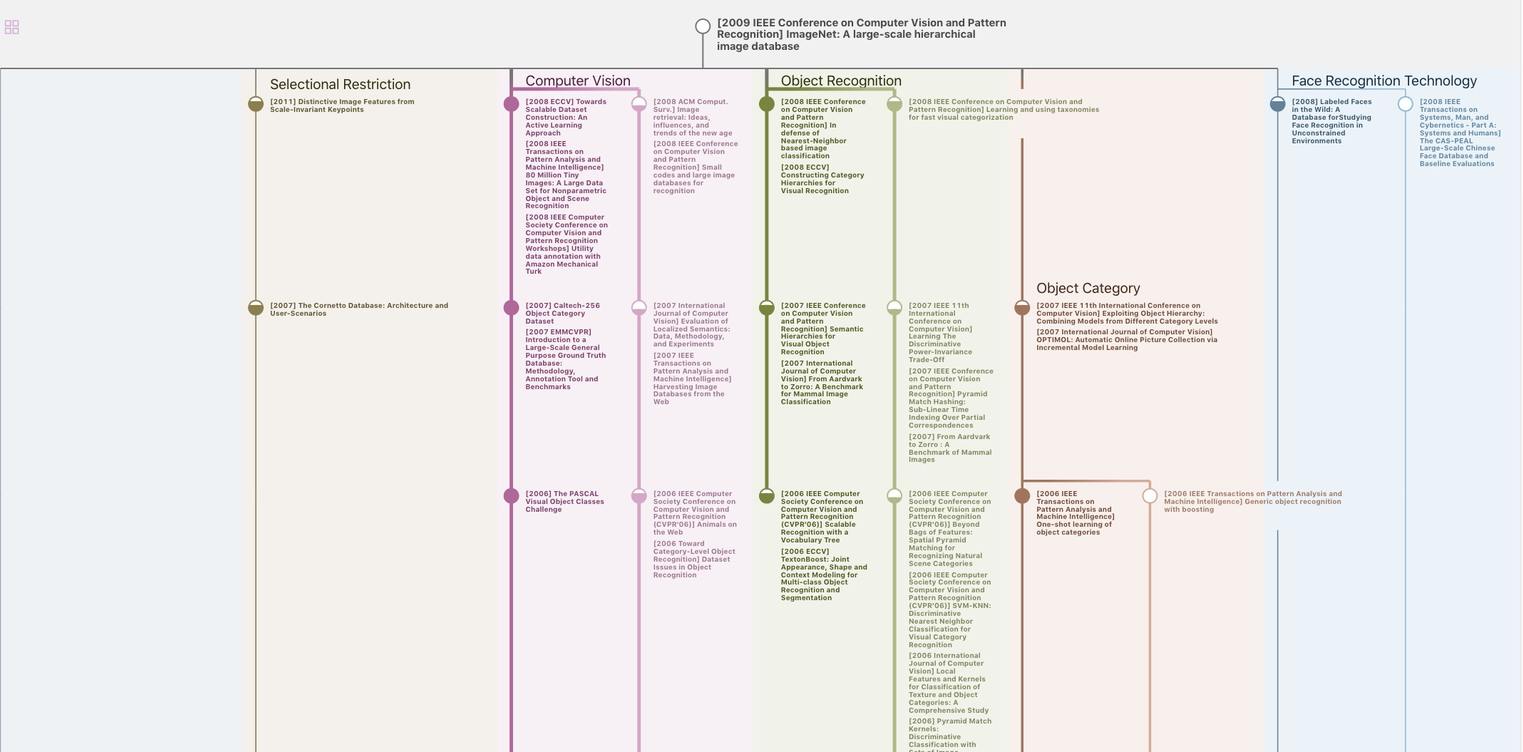
生成溯源树,研究论文发展脉络
Chat Paper
正在生成论文摘要