A Novel Cascade Instruction Tuning Method for Biomedical NER
ICASSP 2024 - 2024 IEEE International Conference on Acoustics, Speech and Signal Processing (ICASSP)(2024)
Abstract
Large language models(LLMs) have achieved remarkable performance on various tasks. However, LLMs suffer from severe limitations in domain generalisation, primarily due to inherent limitations. Closed-source LLMs face constraints in fine-tuning, while open-source LLMs contend with the scarcity of domain-specific data. Moreover, LLMs often prioritize addressing standard patterns, inadvertently neglecting intricate and domain-specific patterns. This preference hampers effective domain generalization. In this paper, inspired by curriculum learning, we explore a cascade instruction tuning method to train a domain-specific LLMs that can excel in a broad application such as information extraction. Taking biomedical name entity recognition(BioNER) as a case study, we show how to cultivate general LLMs into domain-specific LLMs with limited domain data and address the complex pattern for BioNER. To validate our method, we construct NER INSTRUCTIONS, the largest and broadest benchmark sourced from 55 publicly available NER datasets across 17 domains. We conduct extensive experiments on the dataset, and the results demonstrate the effectiveness of our proposed framework in downstream task generalisation and its ability to tackle intricate patterns.
MoreTranslated text
Key words
large language model,instruction learning,biomedical named entity recognition,domain adaption
AI Read Science
Must-Reading Tree
Example
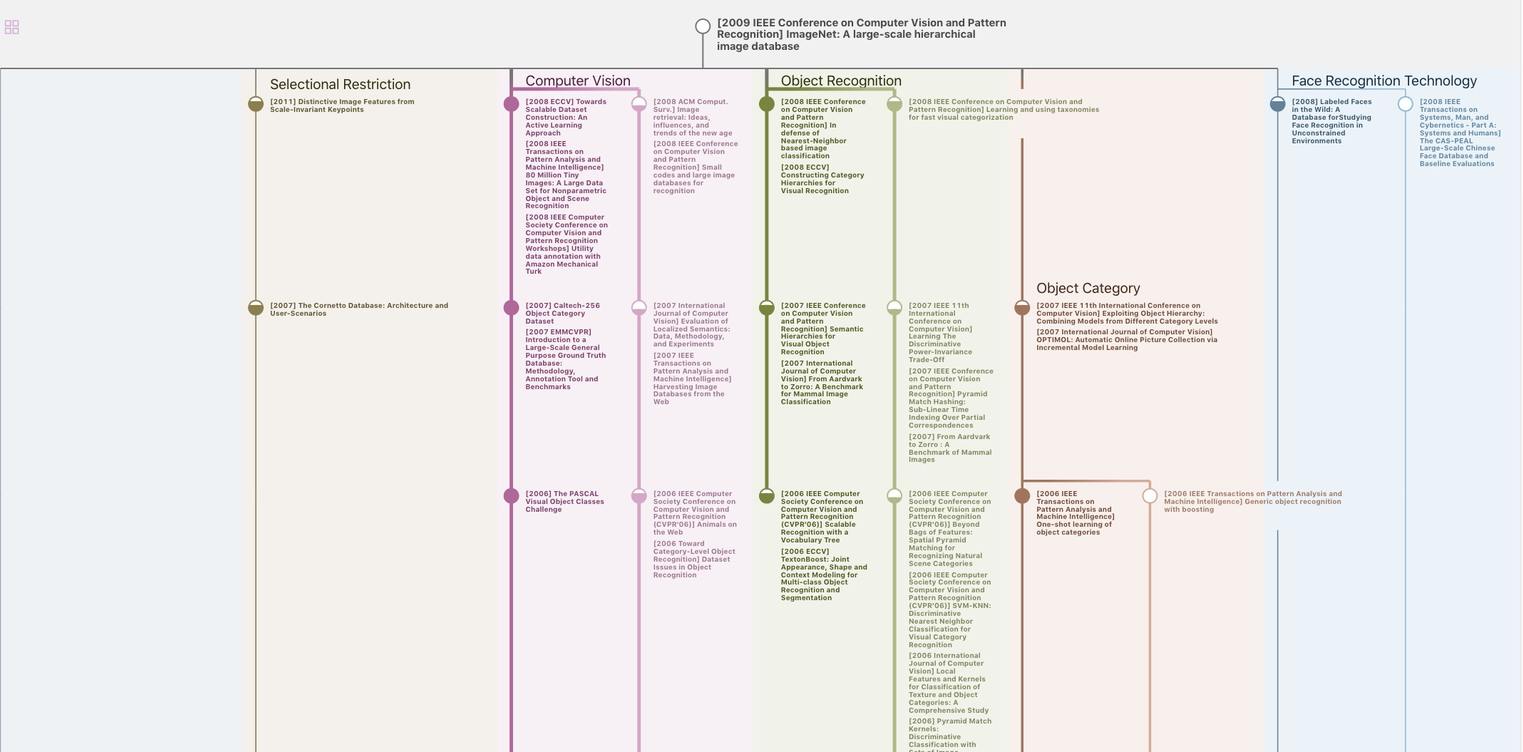
Generate MRT to find the research sequence of this paper
Chat Paper
Summary is being generated by the instructions you defined