Cross-Image Distillation for Semi-Supervised Semantic Segmentation
ICASSP 2024 - 2024 IEEE International Conference on Acoustics, Speech and Signal Processing (ICASSP)(2024)
摘要
Semi-supervised semantic segmentation approaches have drawn much more attention in recent years, which aim to exploit a large amount of unlabeled data together with a small number of labeled data. However, existing models usually regarded segmentation as pixel-wise classification, neglecting global semantic relations among pixels across various images. Moreover, scarce annotated data usually exhibits a biased distribution against the desired one, hindering performance improvement. To address these challenging problems, we propose a novel cross-image distillation framework for semi-supervised semantic segmentation. Specifically, we introduce a relation distillation module to model inter-channel correlations between features of labeled samples and unlabeled samples. In addition, we propose a style distillation strategy to explicitly calibrate the learned feature distributions of labeled and unlabeled data to be aligned. Experimental results on two popular benchmarks demonstrate that our proposed approach achieves superior performance over other state-of-the-art methods. We will release the code soon.
更多查看译文
关键词
semi-supervised learning,semantic segmentation,cross-image distillation,style distillation
AI 理解论文
溯源树
样例
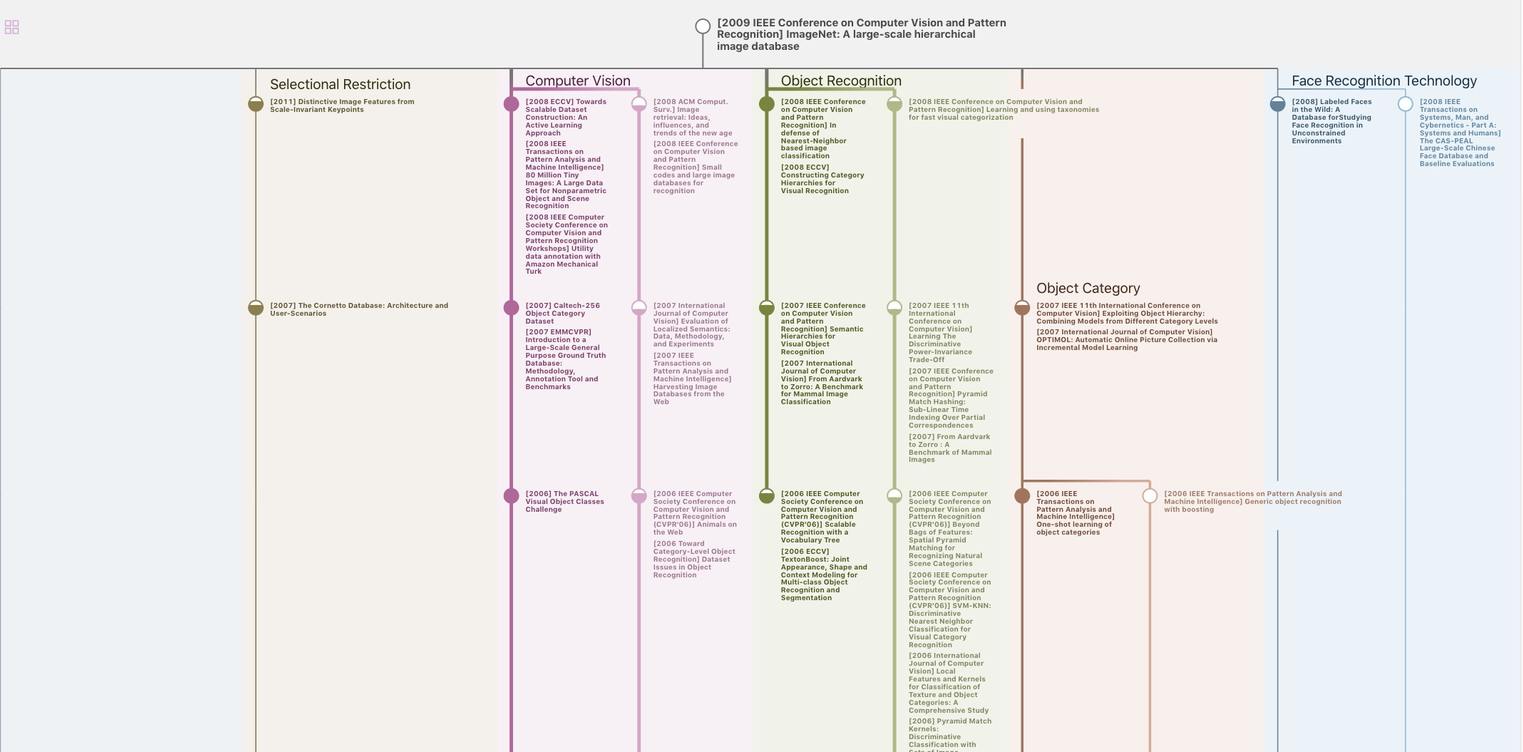
生成溯源树,研究论文发展脉络
Chat Paper
正在生成论文摘要