Long-Term Social Interaction Context: The Key to Egocentric Addressee Detection
ICASSP 2024 - 2024 IEEE International Conference on Acoustics, Speech and Signal Processing (ICASSP)(2024)
摘要
As embodied agents learn to interact, it is crucial for them to understand when, what, and to whom they should respond. While advances in natrual language processing and speech technologies have enabled conversational agents to focus on what to respond, they still struggle to determine when and to whom they should respond. In this paper, we address the addressee detection (Talking-To-Me, TTM) problem under the egocentric view. Instead of relying solely on short-term audio and video data, we propose a simple architecture SICNet with self/cross-modality attention that leverages long-term social interaction context. By leveraging long-term information, our approach has achieved a mean Average Precision (mAP) of 68.98% on the Ego4D TTM task, surpassing the previous state-of-the-art single-task model by 10.07%. We also conducted a detailed ablation study to demonstrate the effectiveness of each component in the long-term social interaction context.
更多查看译文
关键词
talking-to-me detection,social interaction detection,multimodal analysis,human-centric analysis
AI 理解论文
溯源树
样例
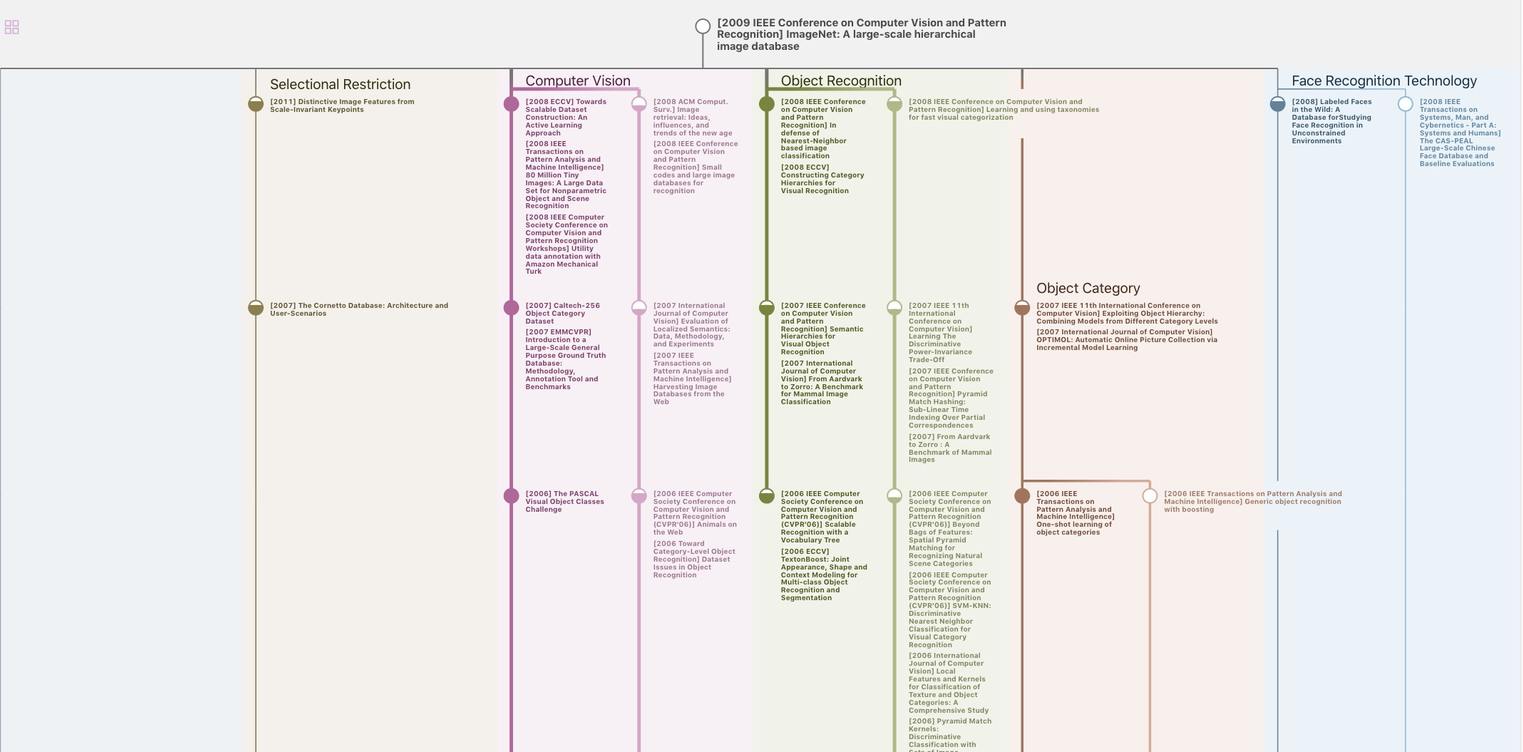
生成溯源树,研究论文发展脉络
Chat Paper
正在生成论文摘要