Heart Murmur Severity Stages Classification Using Multi-kernel Residual CNN
IEEE Sensors Journal(2024)
摘要
Computer-aided diagnosis of heart murmurs (HMs) using phonocardiogram (PCG) signals facilitates early treatment of heart valve diseases (HVDs). This work proposes a novel multi-kernel residual convolutional neural network (MK-RCNN) model to classify HM severity stages. The model employs multi-kernel CNN-based feature extractors to capture scale-specific features from raw PCG segments. Furthermore, residual learning aids in extracting relevant features from deep CNN layers without degrading performance accuracy. The effectiveness of the proposed method is verified through comprehensive testing on three open-access databases. The proposed MK-RCNN model demonstrates an overall accuracy (OA) of 98.33%, overall precision (OPr) of 96.98%, overall recall (ORe) of 97.36%, and overall F1-score (OF1) of 97.05% using the Physionet Challenge 2022 database for HM severity stage classification. We have also evaluated the efficacy of the proposed model for multi-class categories of HMs using the heart sound murmur (HSM) database and binary classification as healthy or pathological using the Physionet Challenge 2016 database. The proposed model’s accurate predictions, low computational complexity, and speedy response make it well-suited for reliable and fast HM diagnosis in primary healthcare units.
更多查看译文
关键词
Heart murmurs (HMs),residual convolutional neural network (RCNN),multi-kernel,identity mapping
AI 理解论文
溯源树
样例
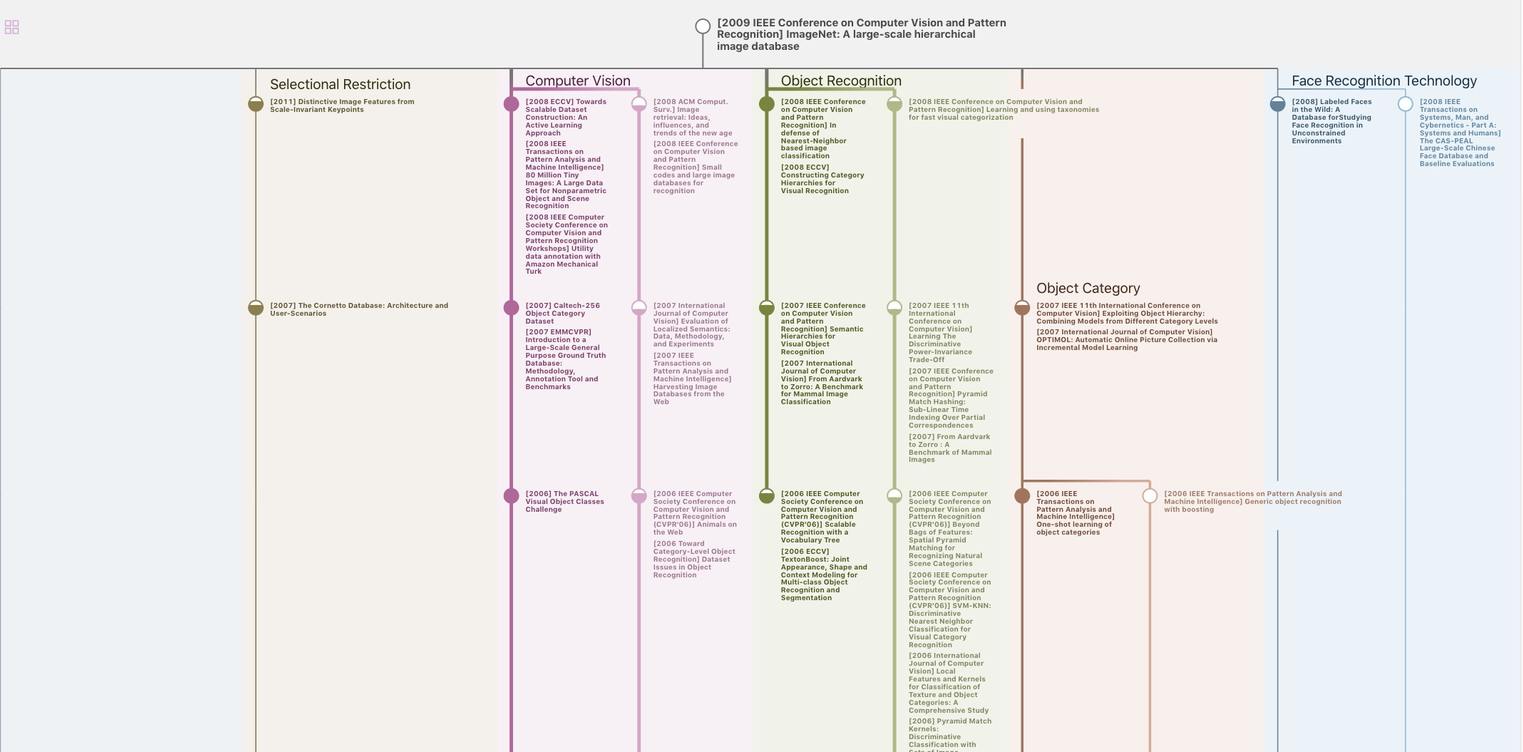
生成溯源树,研究论文发展脉络
Chat Paper
正在生成论文摘要