Graph Partial Label Learning with Potential Cause Discovering
CoRR(2024)
Abstract
Graph Neural Networks (GNNs) have garnered widespread attention for their
potential to address the challenges posed by graph representation learning,
which face complex graph-structured data across various domains. However, due
to the inherent complexity and interconnectedness of graphs, accurately
annotating graph data for training GNNs is extremely challenging. To address
this issue, we have introduced Partial Label Learning (PLL) into graph
representation learning. PLL is a critical weakly supervised learning problem
where each training instance is associated with a set of candidate labels,
including the ground-truth label and the additional interfering labels. PLL
allows annotators to make errors, which reduces the difficulty of data
labeling. Subsequently, we propose a novel graph representation learning method
that enables GNN models to effectively learn discriminative information within
the context of PLL. Our approach utilizes potential cause extraction to obtain
graph data that holds causal relationships with the labels. By conducting
auxiliary training based on the extracted graph data, our model can effectively
eliminate the interfering information in the PLL scenario. We support the
rationale behind our method with a series of theoretical analyses. Moreover, we
conduct extensive evaluations and ablation studies on multiple datasets,
demonstrating the superiority of our proposed method.
MoreTranslated text
AI Read Science
Must-Reading Tree
Example
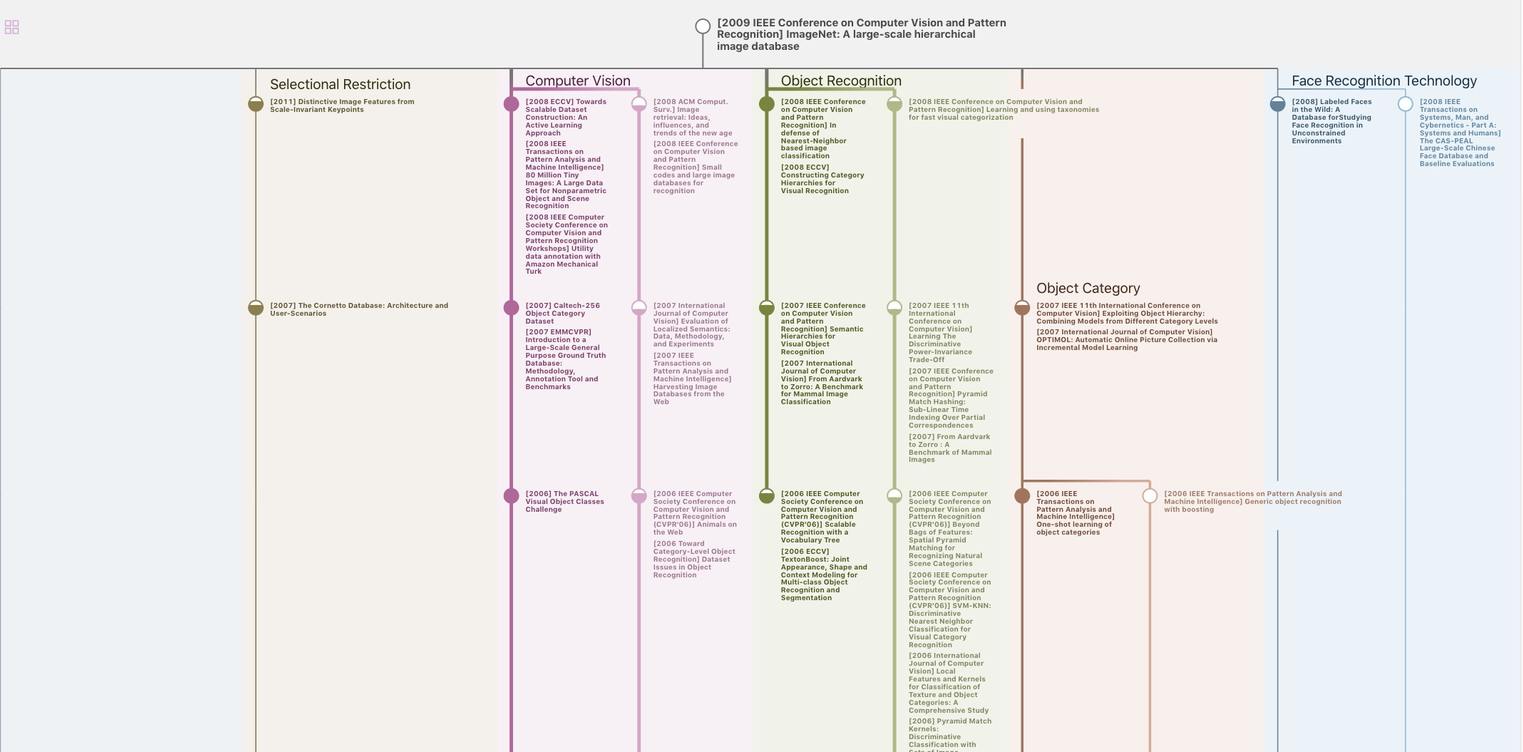
Generate MRT to find the research sequence of this paper
Chat Paper
Summary is being generated by the instructions you defined