AMNet: Learning to Align Multi-modality for RGB-T Tracking
IEEE Transactions on Circuits and Systems for Video Technology(2024)
摘要
RGB-T tracking has attracted increasing attention recently due to the all-weather and all-day working capability. However, most current RGB-T trackers usually assume that RGB data and thermal infrared (TIR) data are well spatially aligned, which is difficult to be achieved in practice. Such spatial misalignment between RGB data and TIR data may lead to the ineffective cross-modal information propagation during multi-modal feature fusion, thus reducing the tracking performance. In addition, due to the discrepancy in imaging characteristics of RGB images and TIR images, there also exist great differences between the information captured by the two modality data. The differences in characteristics of RGB and TIR modalities in different local areas will cause a single fusion strategy to be unable to fully explore the complementary information within multi-modal data. For that, we propose an RGB-T tracker, referred to as AMNet, to specifically solve such two problems with two dedicated modules, i.e., a Mutual-interacted Spatial Alignment (MSA) module and an Information Matching Fusion (IMF) module. The former spatially aligns the two modality data through three essential parts, including interactions of multi-modal features, prediction of cross-modal offset map, and enhancement of the aligned features. While the latter first discriminates different types of local regions by employing several intra-modal attention modules and then uses a divide-and-conquer fusion strategy to exploit such discriminative information within RGB and TIR features of different cases for tracking. We validate the effectiveness of our AMNet with extensive experiments on three RGB-T benchmarks, which achieves new state-of-the-art performance.
更多查看译文
关键词
RGB-T tracking,Spatial misalignment,Information differences,Divide-and-conquer fusion
AI 理解论文
溯源树
样例
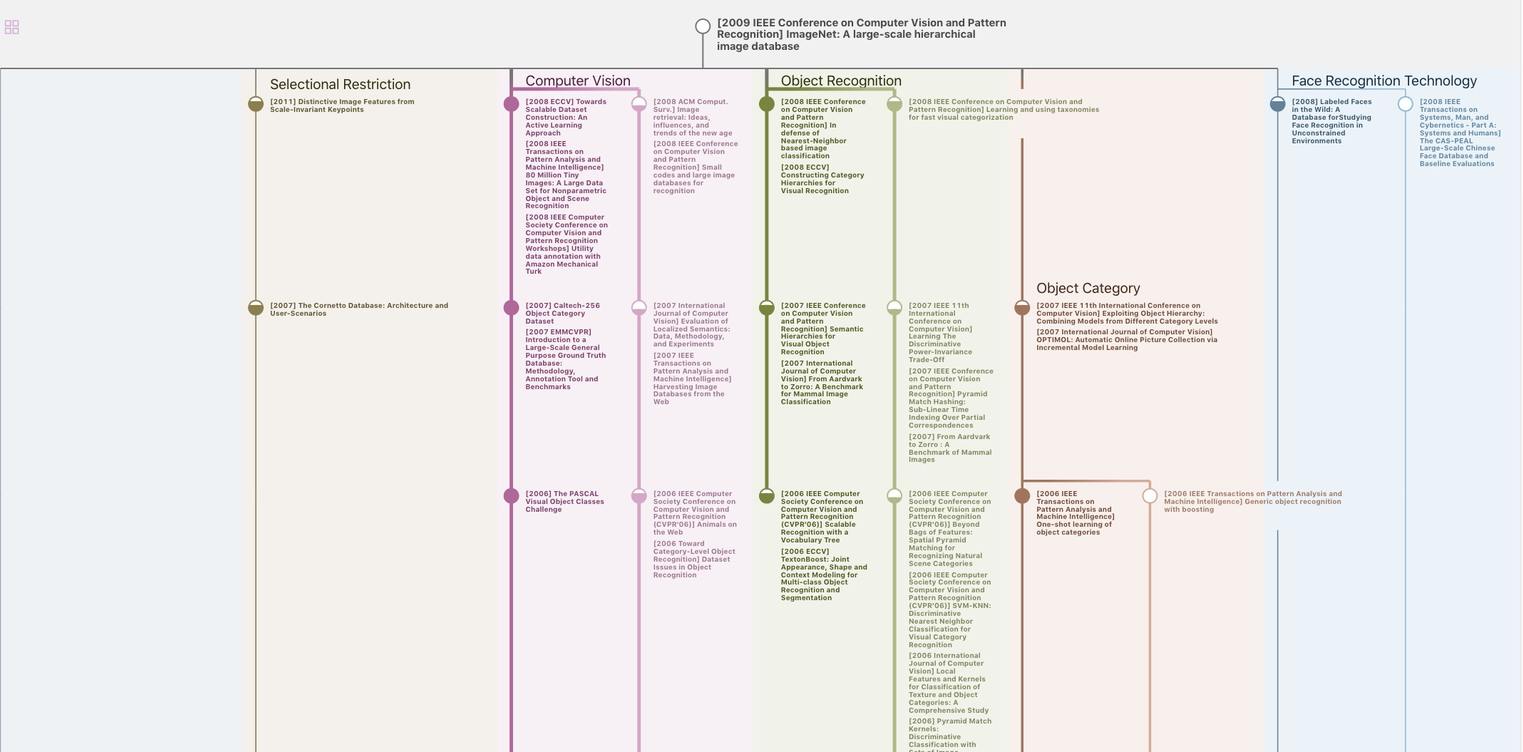
生成溯源树,研究论文发展脉络
Chat Paper
正在生成论文摘要