Local and Global: Text Matching Via Syntax Graph Calibration
ICASSP 2024 - 2024 IEEE International Conference on Acoustics, Speech and Signal Processing (ICASSP)(2024)
摘要
Pre-trained models such as BERT have achieved remarkable results in text matching tasks. However, existing models still suffer from the challenge of capturing local subtle differences when modeling complex semantic matching relationships. In this work, we find that the integration of local syntax awareness and global semantics is crucial for text matching. Meanwhile, we propose the Local and Global Syntax Graph Calibration (LG-SGC) module, which can explore local syntactic and global semantic information for the matching task. Specifically, we first introduce an auxiliary task inside BERT to capture local subtle grammatical differences. Then, we retain the original attention operation to capture global matching features. Finally, we design an information fusion layer to effectively combine local and global information to deepen the understanding of the matching task. We conduct extensive experiments on 10 benchmarks, and LG-SGC significantly outperforms previous models.
更多查看译文
关键词
syntax graph,attention calibration,semantic matching,neural language processing
AI 理解论文
溯源树
样例
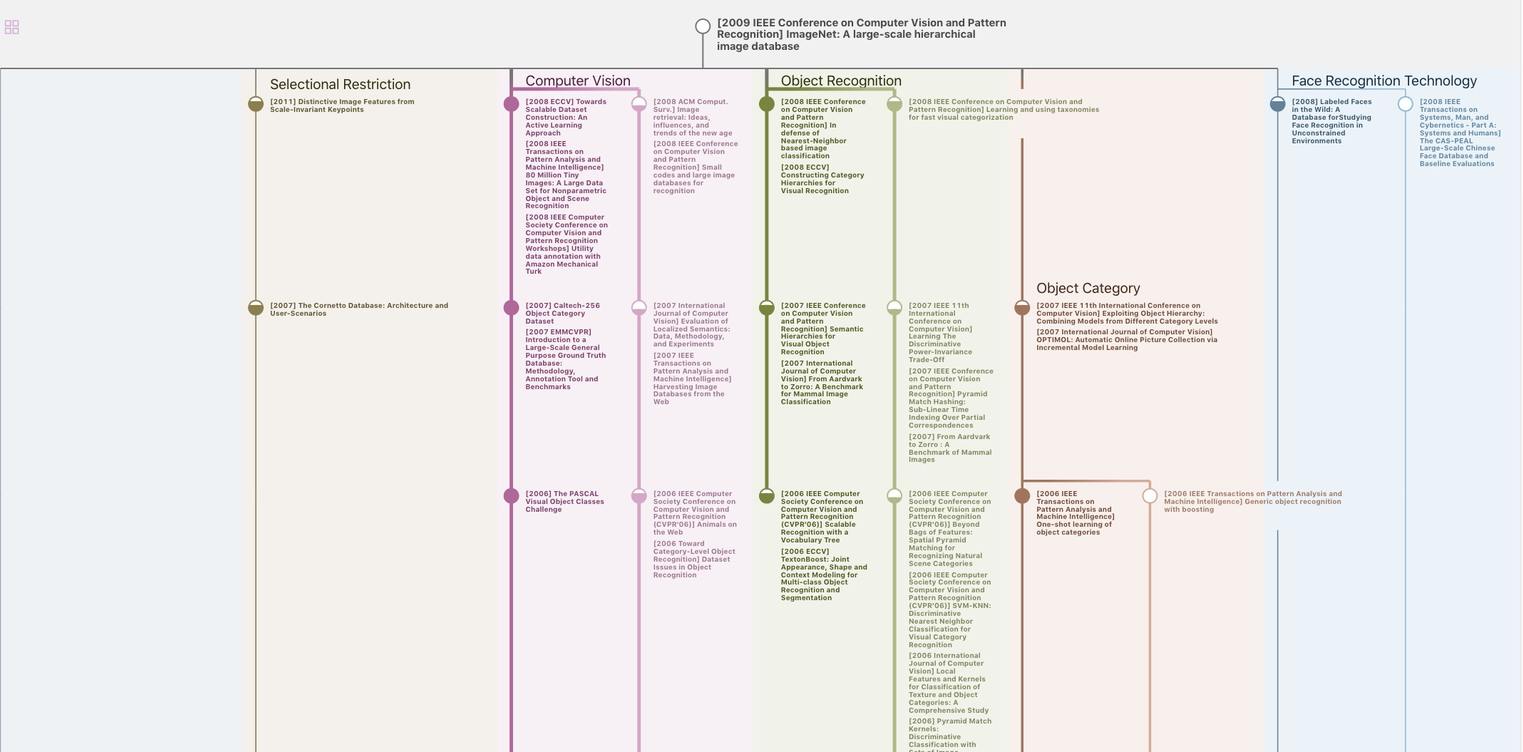
生成溯源树,研究论文发展脉络
Chat Paper
正在生成论文摘要