SADE: A Speaker-Aware Dual Encoding Model Based on Diagbert for Medical Triage and Pre-Diagnosis
ICASSP 2024 - 2024 IEEE International Conference on Acoustics, Speech and Signal Processing (ICASSP)(2024)
摘要
Medical triage and diagnosis systems are crucial in alleviating the severe burden on healthcare systems, providing great assistance and convenience to patients with limited medical knowledge. Most current studies, however, struggle to effectively learn speaker-aware information in patient-doctor dialogues. Besides, the professional terms and disease-related expressions make it difficult for traditional methods to understand medical knowledge. To solve these challenges, we propose a novel Speaker-Aware Dual Encoding (SADE) model, which employs two encoders of identical structure to separately represent patient consultations and doctor diagnoses. To grasp medical knowledge, we introduce DiagBERT, pre-trained on numerous domain-specific texts to represent input utterances. We then integrate BiLSTM and Dendrite Network into each encoder to capture sequential and interactional semantics. Moreover, we have built two large-scale Triage and Diagnosis datasets to overcome the scarcity of public medical corpora. Extensive experimental results on these datasets indicate that SADE outperforms other baseline models.
更多查看译文
关键词
Patient triage,Pre-diagnosis,Dual encoding,Pre-trained language model,Dendrite network
AI 理解论文
溯源树
样例
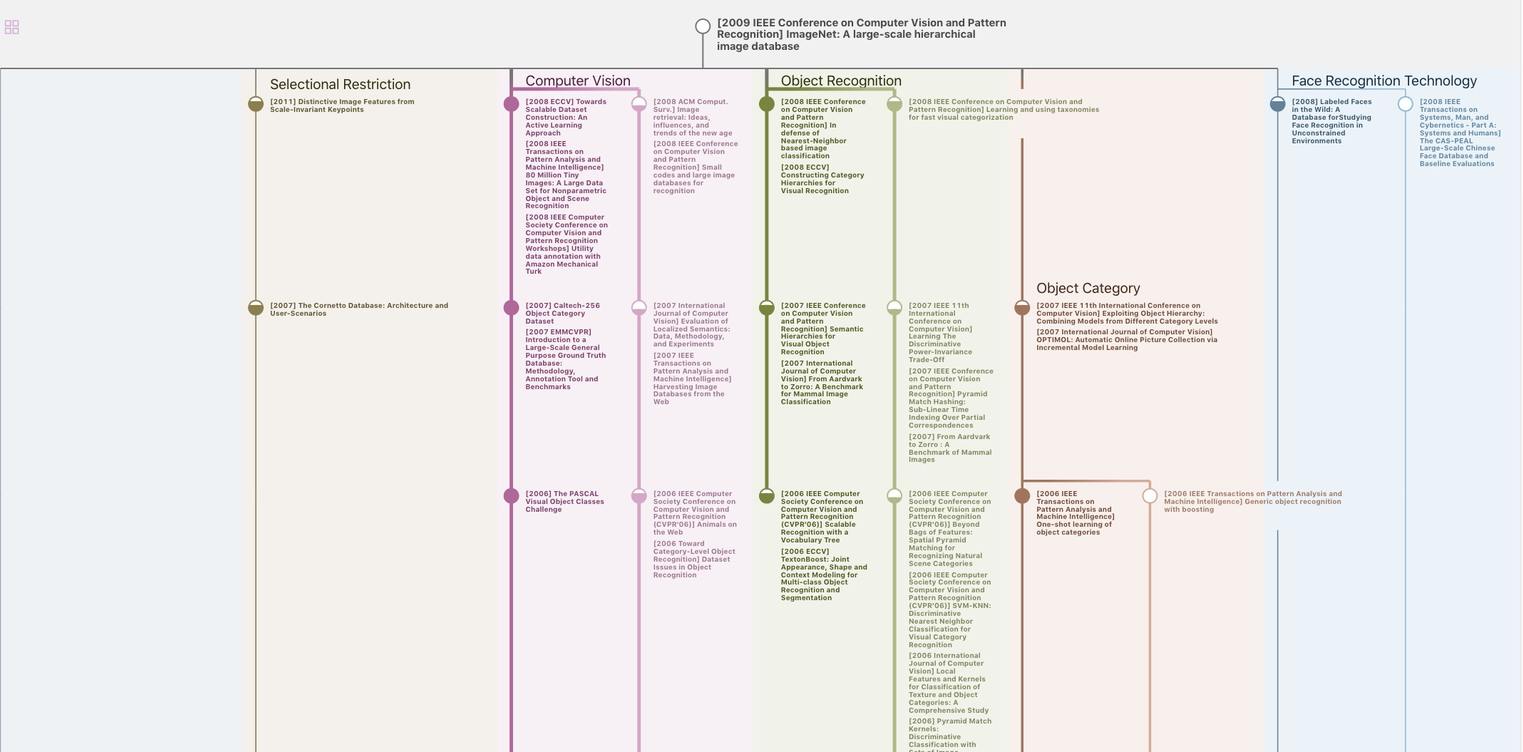
生成溯源树,研究论文发展脉络
Chat Paper
正在生成论文摘要