ADHD Diagnosis and Biomarker Detection Based on Multimodal Graph Convolutional Neural Network
ICASSP 2024 - 2024 IEEE International Conference on Acoustics, Speech and Signal Processing (ICASSP)(2024)
摘要
In this study, we apply a graph convolutional network (GCN) in attention deficit hyperactivity disorder (ADHD) classification by using multimodal data. Here, multimodal data is integrated to construct a dual graph for leveraging the modality information. Then, a GCN learning model is performed within an existing binary hypothesis testing (BHT) framework to fulfill the classification task. To preserve specifical topological structure of dual graph, we propose a graph-based feature selection approach to choose typical data (nodes) on the graphs. With these typical data, we design our GCN model, effectively learning the high-level features for identifying ADHD subjects. The experiments show the average accuracy of our method reaches 95.2% on various ADHD-200 datasets. Importantly, this result is achieved only on the right limbic system rather than the whole brain. The limited brain regions used indicate their potential ability as biomarkers. Moreover, biomarkers are found with their special topological structures. On the Peking University dataset, the found biomarkers center around the hippocampus and parahippocampal gyri, which are highly correlated with ADHD disease. Differing from isolated biomarker findings in traditional methods, our method discloses the abnormal circuit for ADHD and is more meaningful for further learning ADHD mechanism.
更多查看译文
关键词
ADHD classification,graph convolutional network,limbic system,multimodal data
AI 理解论文
溯源树
样例
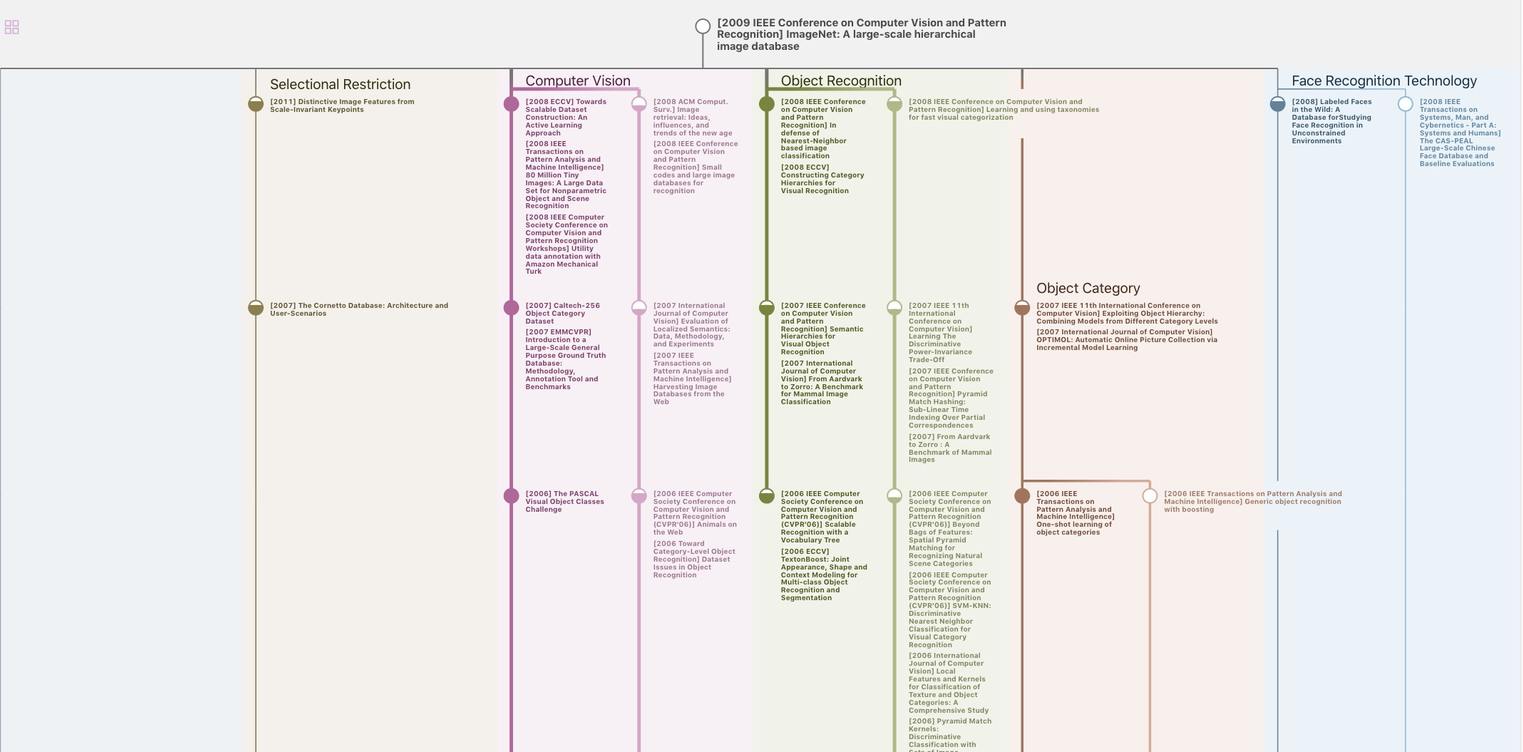
生成溯源树,研究论文发展脉络
Chat Paper
正在生成论文摘要