Rockburst Intensity Grade Prediction Based on Data Preprocessing Techniques and Multi-model Ensemble Learning Algorithms
Rock Mechanics and Rock Engineering(2024)
摘要
Rockburst is a mine dynamic disaster caused by the rapid release of elastic strain energy of surrounding rock. As the depth of engineering project operations increases, accurate classification of rockburst intensity cannot be achieved based on conventional criteria due to high uncertainty and unpredictability of rockburst. In this regard, an AOA-Voting-Soft ensemble machine learning was proposed in this study by combining seven individual classifiers, i.e., eXtreme gradient boosting, support vector machines, multilayer perceptron, k-nearest neighbor, random forest, naive Bayesian, and gradient boosting decision Tree. In addition, outliers were eliminated by means of density-based spatial clustering of applications with noise, and CURE-MeanradiusSMOTE was adopted to obtain a balanced data structure. Furthermore, the optimal combination of classifiers in Voting was determined by the game theory and the exhaustive search method. Weights of individual learners in Voting were determined through the arithmetic optimization algorithm and fivefold cross-validation. The results show that the prediction accuracy of the ensemble algorithm proposed in this study is 4.4
更多查看译文
关键词
Rockburst grade,Classification prediction,Data preprocessing,Ensemble learning,Game theory
AI 理解论文
溯源树
样例
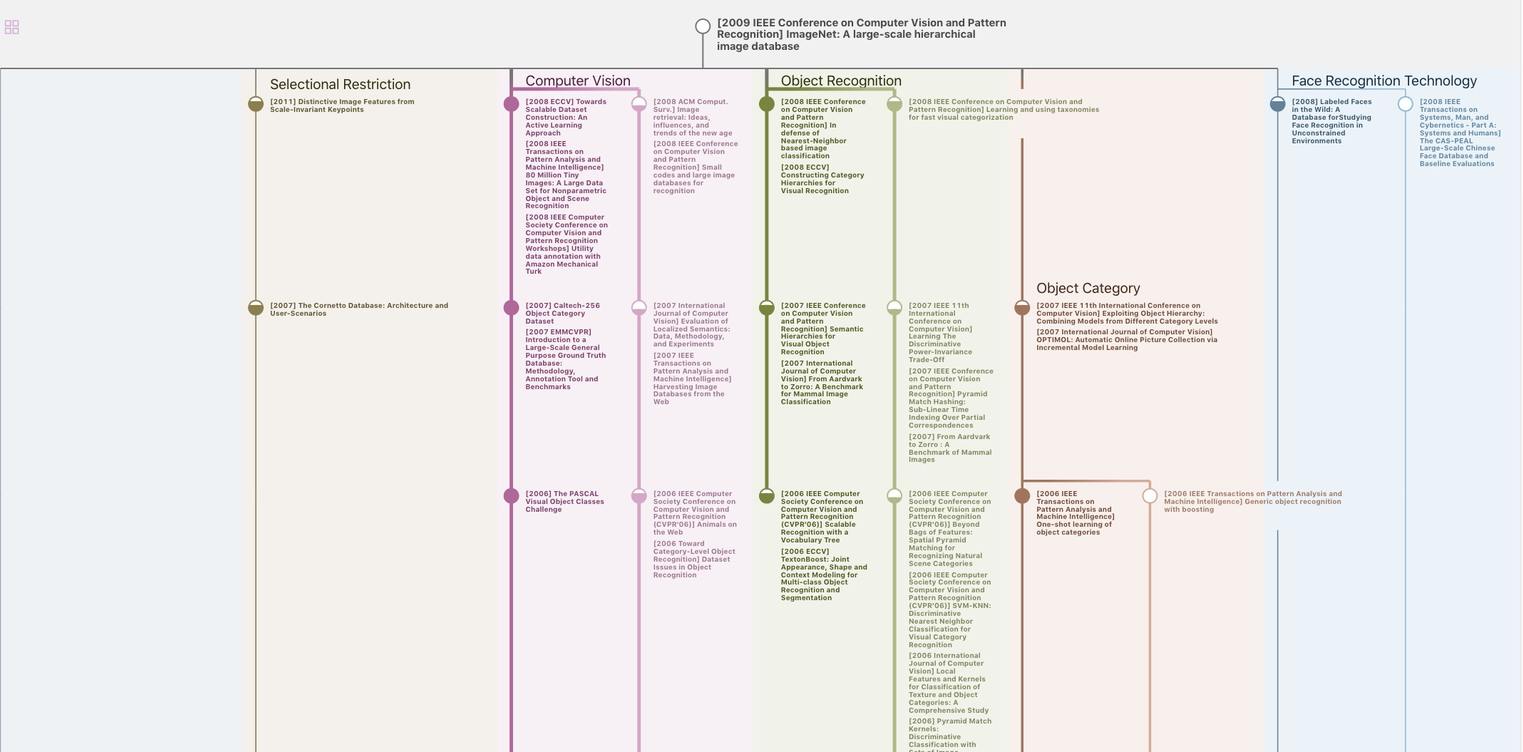
生成溯源树,研究论文发展脉络
Chat Paper
正在生成论文摘要