Graph Convolutional Neural Networks In The Companion Model
ICASSP 2024 - 2024 IEEE International Conference on Acoustics, Speech and Signal Processing (ICASSP)(2024)
摘要
Graph Convolutional Neural Networks (graph CNNs) adapt the traditional CNN architecture for use on graphs, replacing convolution layers with graph convolution layers. Although similar in architecture, graph CNNs are used for geometric deep learning whereas conventional CNNs are used for deep learning on grid-based data, such as audio or images, with seemingly no direct relationship between the two classes of neural networks.This paper shows that under certain conditions traditional CNNs can be used with graph data as a good approximation to graph CNNs, avoiding the need for graph CNNs. We show this by using an alternative graph signal representation – the graph companion model that we recently proposed in [1]. Instead of using the given graph and signal in the nodal domain, the graph companion model uses the equivalent companion graph and signal representation in the companion domain. By this way, the graph CNN architecture in the nodal domain is equivalent to our deep learning architecture: a traditional CNN in the companion domain with appropriate boundary conditions (b.c.). The paper shows that we obtain similar results on graph classification experiments using a traditional CNN in the companion domain vs. the usual graph CNNs in the nodal domain.
更多查看译文
关键词
Graph Convolution,Companion Model,Convolutional Neural Networks,Graph Signal Processing,Graph Classification,Graph Neural Networks
AI 理解论文
溯源树
样例
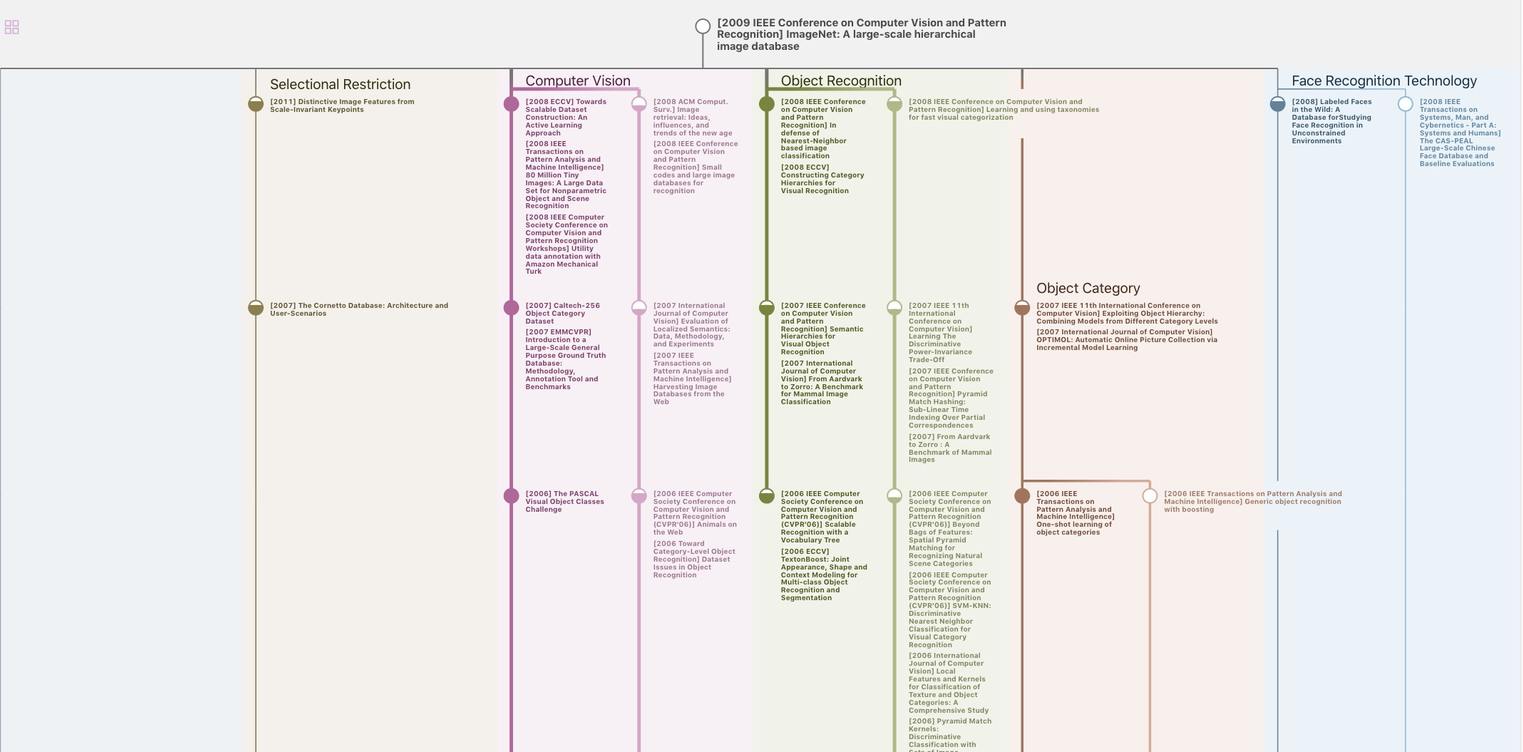
生成溯源树,研究论文发展脉络
Chat Paper
正在生成论文摘要