Transcription factor prediction using protein 3D structures
crossref(2024)
摘要
Motivation: Transcription factors (TFs) are DNA-binding proteins that regulate expressions of genes in an organism. Hence, it is important to identify novel TFs. Traditionally, novel TFs have been identified by their sequence similarity to the DNA-binding domains (DBDs) of known TFs. However, this approach can miss to identify a novel TF that is not sequence similar to any of the known DBDs. Hence, computational methods have been developed for the TF prediction task that, instead of relying on known DBDs, use sequence features of proteins to train a machine learning model, in order to capture sequence patterns that distinguish TFs from other proteins. Because 3-dimensional (3D) structure of a protein captures more information than its sequence, using 3D protein structures can more correctly predict novel TFs. Results: We propose the first deep learning-based TF prediction method (named StrucTFactor) based on 3D protein structures. We compare StrucTFactor with a recent state-of-the-art TF prediction method that relies only on protein sequences. We evaluate the considered methods on ~550,000 proteins across 12 datasets, capturing different aspects of data bias (including sequence redundancy and 3D protein structural quality) that can influence a method's performance. We find that StrucTFactor significantly (p-value < 0.001) outperforms the existing state-of-the-art TF prediction method, improving performance by up to 23% based on Matthews correlation coefficient. Our results show the importance of using 3D protein structures to predict novel TFs. We provide StrucTFactor as a computational pipeline.
更多查看译文
AI 理解论文
溯源树
样例
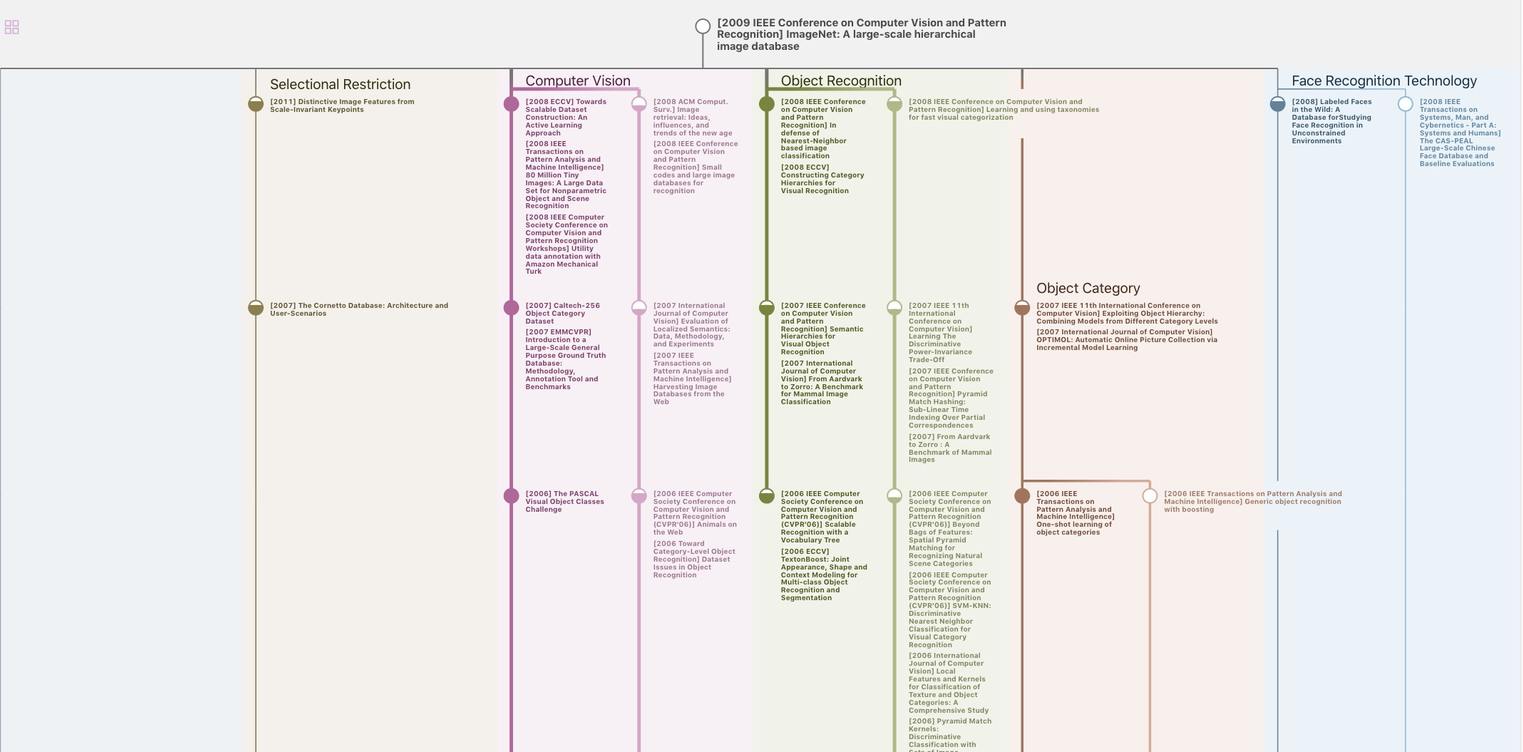
生成溯源树,研究论文发展脉络
Chat Paper
正在生成论文摘要