A Deep-learning surrogate model for the fast calculation of temperature distribution of ±500kV RIP bushings
crossref(2024)
摘要
As a vital component of converter transformers, resin impregnated paper (RIP) bushings withstand high voltage and loading current for the long time operation, which makes the temperature distribution become one of the key issue to characterize its status. However, the temperature monitoring of RIP bushing used to be based on infrared observation in the past, the internal temperature field distribution is difficult to analyze and evaluate in real-time. Therefore, this paper proposes a deep learning surrogate model (DLSM) to quickly and accurately predict the temperature field of the RIP bushing. DLSM consists of two different models, which including the POD reduced-order model based on BP neural network(BPNN) and the CNN, and it calculate the temperature field distribution inside and outside the bushing respectively to improve accuracy. The effect of train size and simulation boundary condition perturbation on the calculation results is analyzed. The results show that comparing with the finite element method(FEM) simulation results, the average relative error of the temperature field is less than 0.7%. The train size of at least 108 samples can have good accuracy for DLSM. When the input disturbance is within 5%, the results are in good agreement with the FEM simulation results. In addition, the calculation time of DLSM is 104 orders faster than the CFD solver, verifying that the model has excellent accuracy, robustness and real-time performance. This method can provide an efficient analysis tool for visualizing the internal status of bushing, and provide a reliable and competitive option for digital twins and thermal fault analysis of bushing.
更多查看译文
AI 理解论文
溯源树
样例
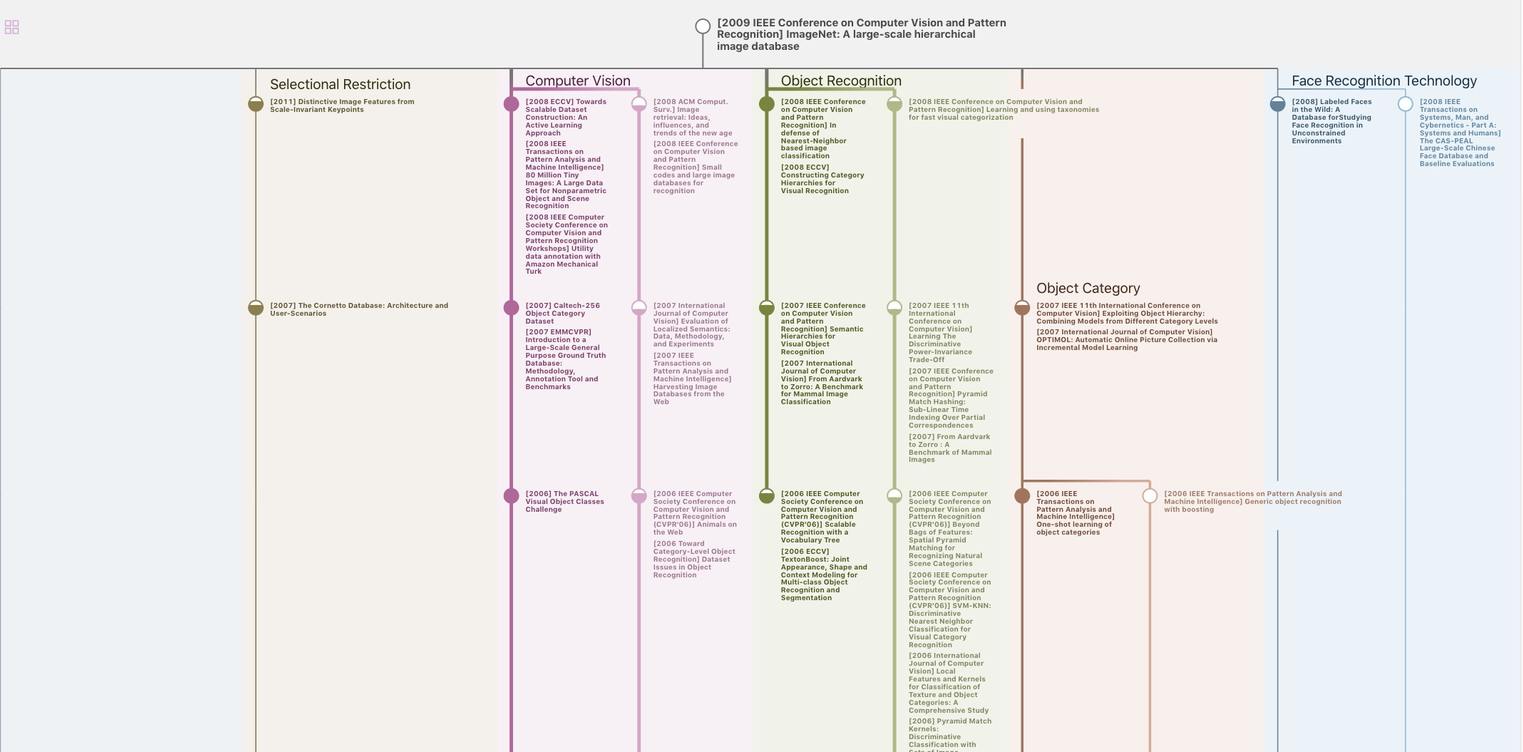
生成溯源树,研究论文发展脉络
Chat Paper
正在生成论文摘要