Sample Size Optimization for Digital Soil Mapping: An Empirical Example
LAND(2024)
摘要
In the evolving field of digital soil mapping (DSM), the determination of sample size remains a pivotal challenge, particularly for large-scale regional projects. We introduced the Jensen-Shannon Divergence (DJS), a novel tool recently applied to DSM, to determine optimal sample sizes for a 2790 km2 area in Ontario, Canada. Utilizing 1791 observations, we generated maps for cation exchange capacity (CEC), clay content, pH, and soil organic carbon (SOC). We then assessed sample sets ranging from 50 to 4000 through conditioned Latin hypercube sampling (cLHS), feature space coverage sampling (FSCS), and simple random sampling (SRS) to calibrate random forest models, analyzing performance via concordance correlation coefficient and root mean square error. Findings reveal DJS as a robust estimator for optimal sample sizes-865 for cLHS, 874 for FSCS, and 869 for SRS, with property-specific optimal sizes indicating the potential for enhanced DSM accuracy. This methodology facilitates a strategic approach to sample size determination, significantly improving the precision of large-scale soil mapping. Conclusively, our research validates the utility of DJS in DSM, offering a scalable solution. This advancement holds considerable promise for improving soil management and sustainability practices, underpinning the critical role of precise soil data in agricultural productivity and environmental conservation.
更多查看译文
关键词
Jensen-Shannon divergence,sample size,sample density,conditioned Latin hypercube,feature space coverage,simple random sampling,calibration,learning curve
AI 理解论文
溯源树
样例
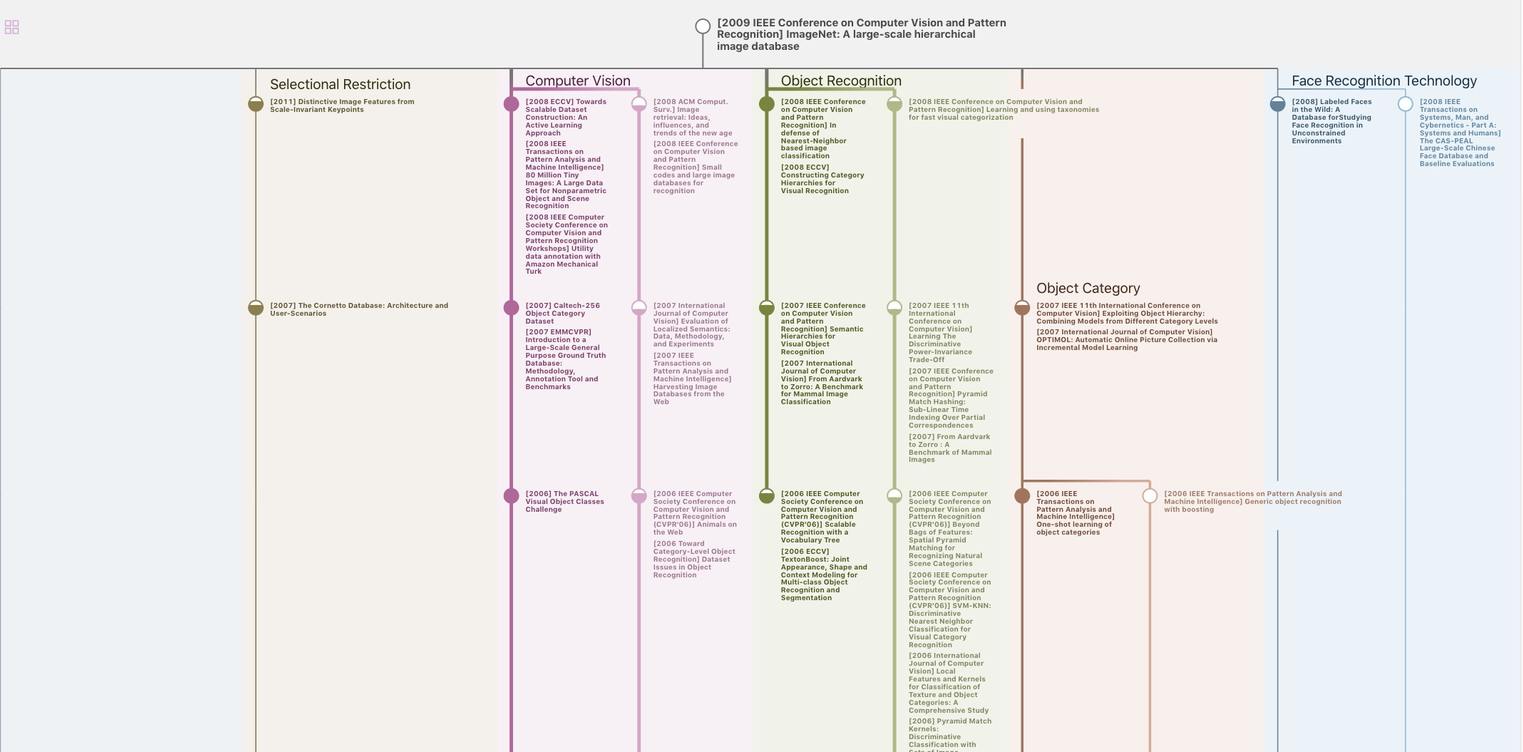
生成溯源树,研究论文发展脉络
Chat Paper
正在生成论文摘要