Underwater target detection and embedded deployment based on lightweight YOLO_GN
The Journal of Supercomputing(2024)
摘要
In order to solve the problem of missing various targets due to the limited memory and computing power of underwater equipment and also the complexity of the underwater environment, a lightweight and efficient underwater target detection algorithm YOLO_GN (YOLO with Ghost network) is proposed. Based on the basic framework of YOLOv5s, the algorithm designs a new backbone using GhostNetV2 and proposes Ghost_BottleneckV2 combined with dynamic sparse attention BiFormer to reduce computational costs and improve detection accuracy. The lightweight multi-scale convolutional LW-GSConv combined with VOV-GSCSP is used to capture the complex features of the input data more accurately and improve the network expression ability. In view of the imbalance of a large number of detection samples in underwater targets, the SlideLoss function is introduced and the optimizer of original model is updated to Sophia, so that the algorithm model has better generalization ability. Finally, the YOLO_GN algorithm is equipped with the Raspberry Pi 4B development board, and the camera is called up to realize real-time detection of underwater targets. Simulation results show that the proposed method can achieve 85.35
更多查看译文
关键词
Object detection,GhostNetV2,YOLOv5s,Lightweight,Backbone network,Embedded deployment
AI 理解论文
溯源树
样例
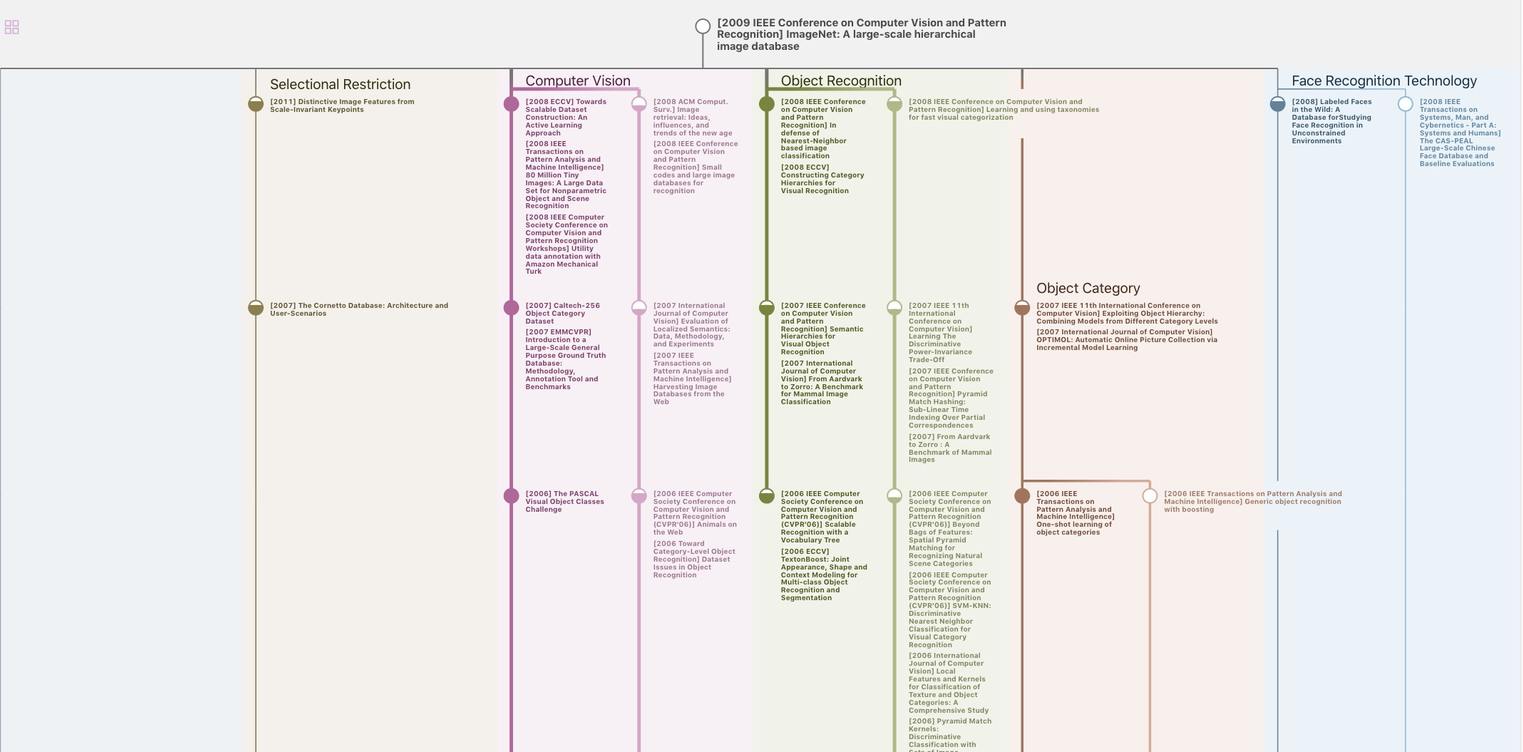
生成溯源树,研究论文发展脉络
Chat Paper
正在生成论文摘要