Model-based approaches to profit-aware recommendation
Expert Systems with Applications(2024)
摘要
Recommender systems are traditionally optimized to facilitate content discovery for consumers by ranking items based on predicted relevance. As such, these systems often do not consider the varying profitability of items for service providers. Since the purpose of recommender systems is usually to create value for both consumers and providers, we hypothesize that integrating profit awareness into recommender systems, considering both consumer relevance and provider profitability, can enhance recommendation outcomes from a provider point of view. In this study, we design and evaluate novel modeling approaches for different families of collaborative filtering algorithms to overcome the existing limitations of conventional reranking methods. Specifically, we show how to embed the business value perspective directly into the loss functions during model training. Through empirical evaluations on three datasets, we show that our proposed models effectively generate recommendations that balance profitability and relevance. Overall, our analyses indicate that these models offer a promising alternative to traditional reranking approaches, particularly because they exhibit improved efficiency during prediction.
更多查看译文
关键词
Recommender systems,Economic recommendations,Business value,Profit awareness,Multi-stakeholder systems
AI 理解论文
溯源树
样例
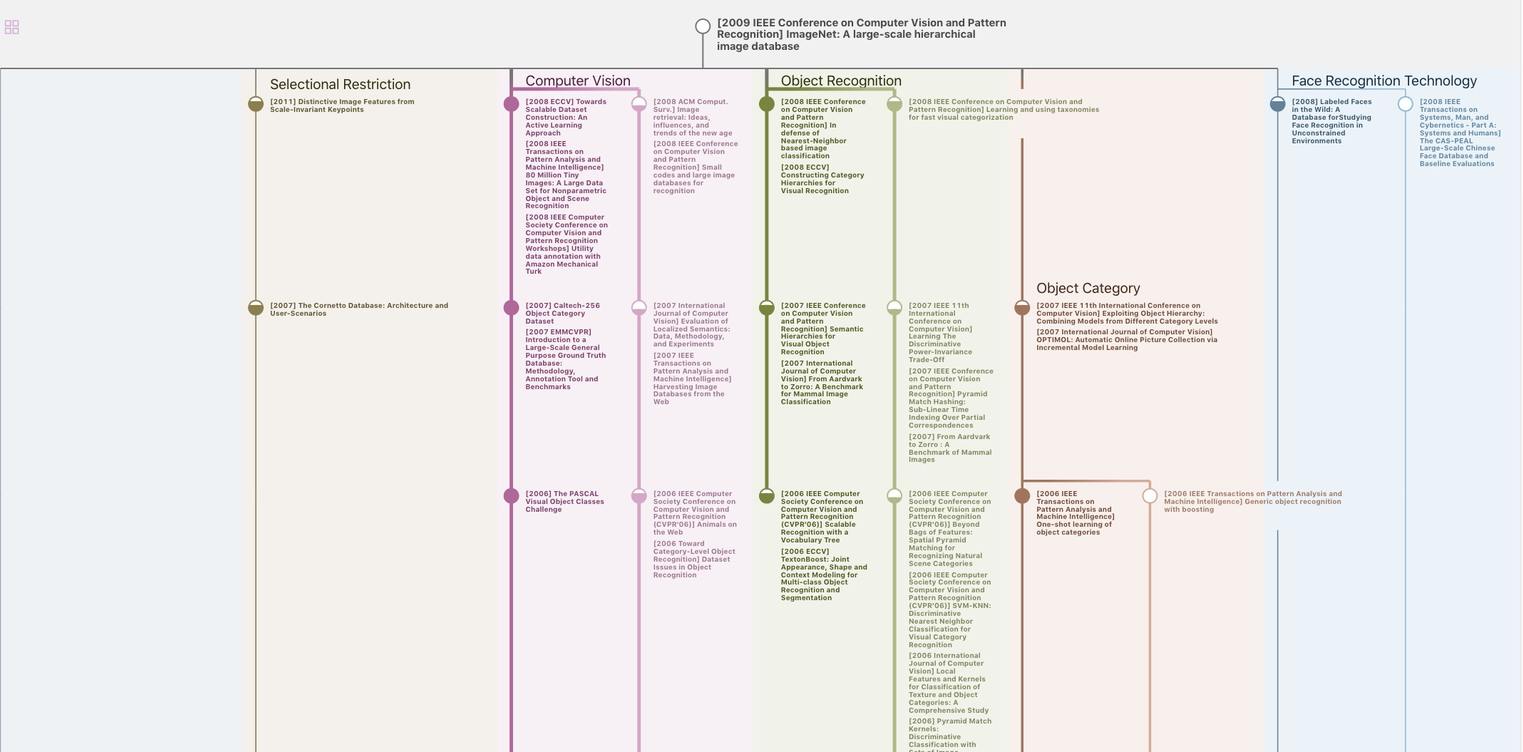
生成溯源树,研究论文发展脉络
Chat Paper
正在生成论文摘要