A novel framework to assess apple leaf nitrogen content: Fusion of hyperspectral reflectance and phenology information through deep learning
Computers and Electronics in Agriculture(2024)
摘要
Rapid and accurate assessment of apple-tree leaf nitrogen content (LNC) plays an important role in smart orchards for accurate fertilization. Hyperspectral remote sensing technology provides a reliable, economical, and fast method to estimate LNC. However, high-dimensional spectral data contain redundant information that is irrelevant to the target parameters, necessitating the development of robust hyperspectral analysis techniques to estimate apple-tree LNC. Additionally, the role of phenological information in improving LNC estimates across multiple growth stages remains uncertain. The present study thus proposes a novel hyperspectral data-processing framework (Boruta-Iteration-DNN) to optimize estimates of apple-tree LNC and investigates the potential of phenological information to improve LNC estimates of multi-fertility apple trees. The proposed framework incorporates the Boruta feature-selection method for identifying important spectral bands and assessing how they affect model performance through iterative feature analysis. A deep neural network (DNN) regression model is then developed for LNC estimation. In addition, a widely used Pearson-DNN method is used for comparison to demonstrate the superiority of the proposed approach. Furthermore, the potential of incorporating phenological information (Day After Anthesis, DAA) into the estimation models is examined to evaluate its ability to better estimate LNC. The results demonstrate that the proposed framework not only enhances the accuracy of apple-tree LNC estimates with respect to the full-spectrum model (without DAA: validation R2 improved from 0.69 to 0.75, NRMSE reduced from 11.92 % to 9.97 %; with DAA: validation R2 improved from 0.72 to 0.79, NRMSE reduced from 11.09 % to 9.06 %), but also reduces the complexity and dimensionality of the models by eliminating 96 % of the redundant spectral bands (2015 bands). Moreover, the inferior results of the Pearson-Iteration-DNN framework further underscore the effectiveness of the Boruta method in selecting relevant spectral bands. Additionally, including the DAA phenological information enhances the accuracy of all models (the total R2 of training and validation increased by 0.05 ∼ 0.12, and the total NRMSE reduced by 1.49 % ∼ 1.93 %). These findings highlight the ability of DNN models integrated with hyperspectral data and phenological information to accurately assess LNC in apple trees, thereby offering valuable guidance to orchard management for developing precise fertilization strategies.
更多查看译文
关键词
Apple,Leaf Nitrogen Content (LNC),Phenology,DNN,Data-Processing
AI 理解论文
溯源树
样例
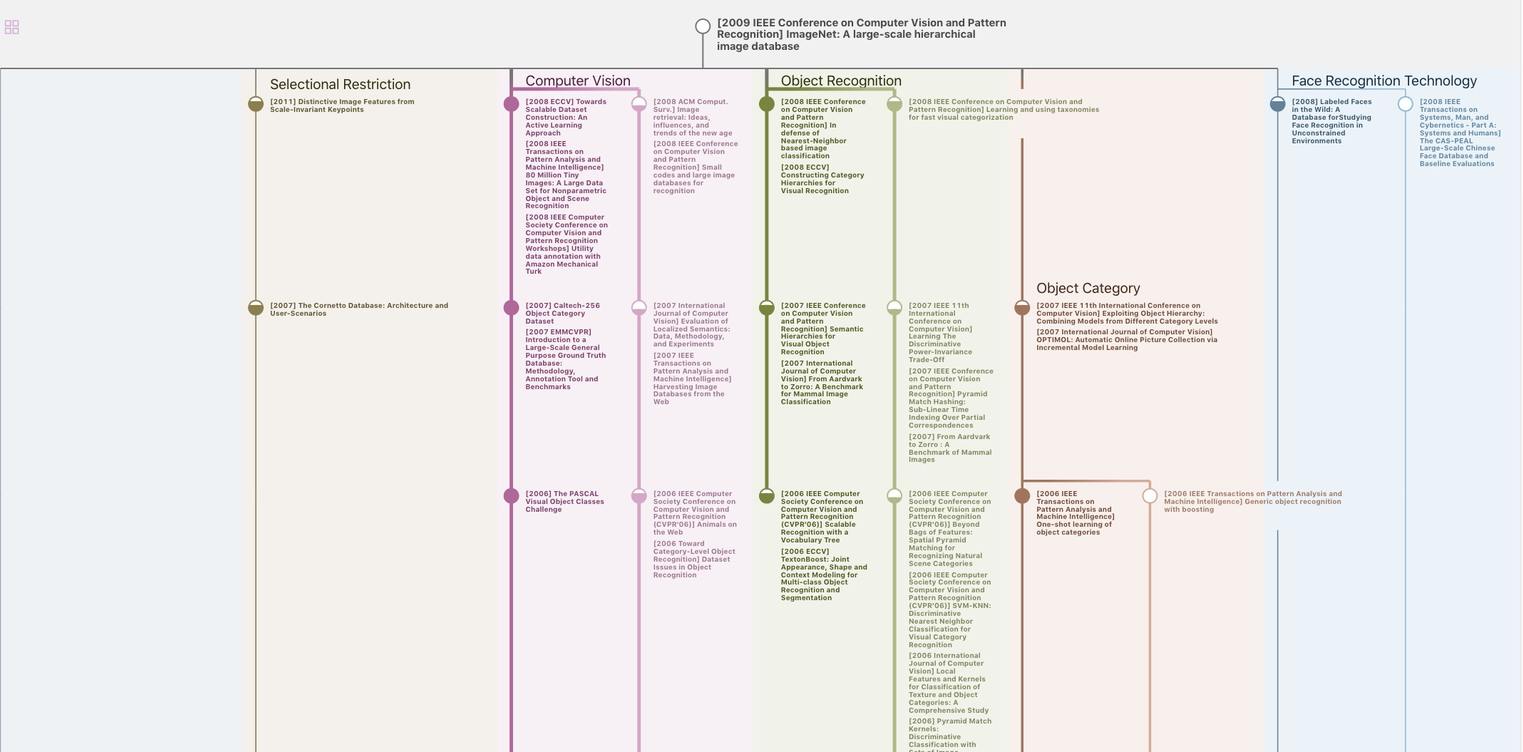
生成溯源树,研究论文发展脉络
Chat Paper
正在生成论文摘要