Introducing vacancy defects by rapid quenching in FCC metal for deep learning micrograph dataset
Materials Characterization(2024)
摘要
Accurate detection of crystal defects from microstructure micrographs is essential for evaluating the mechanical and functional properties of metallic materials. Emerging deep learning techniques have great potential for application in crystal defects detection, but the excellent detection accuracy requires domain knowledge and micrograph dataset. Here we target to detect and distinguish nanoscale dislocation loop (DL) and stacking fault tetrahedron (SFT) in transmission electronic microscope (TEM) micrographs. The micrograph datasets of crystal defects derive from copper prepared by rapid quenching and post-annealing. Applying standard U-Net architecture, the maximum Mean Intersection over Union (mIOUmax) of 3-class model is 54.29%, while it is 73.81% and 74.67% of DL 2-class and SFT 2-class model. Results suggest that excellent detection accuracy of deep learning model could be achieved by learning features from specific defect dataset. These findings provide a new strategy for accurate detection of crystal defects in the field of material engineering.
更多查看译文
关键词
Deep learning,Dislocation loop,Stacking fault tetrahedron,Micrograph dataset,Rapid quenching
AI 理解论文
溯源树
样例
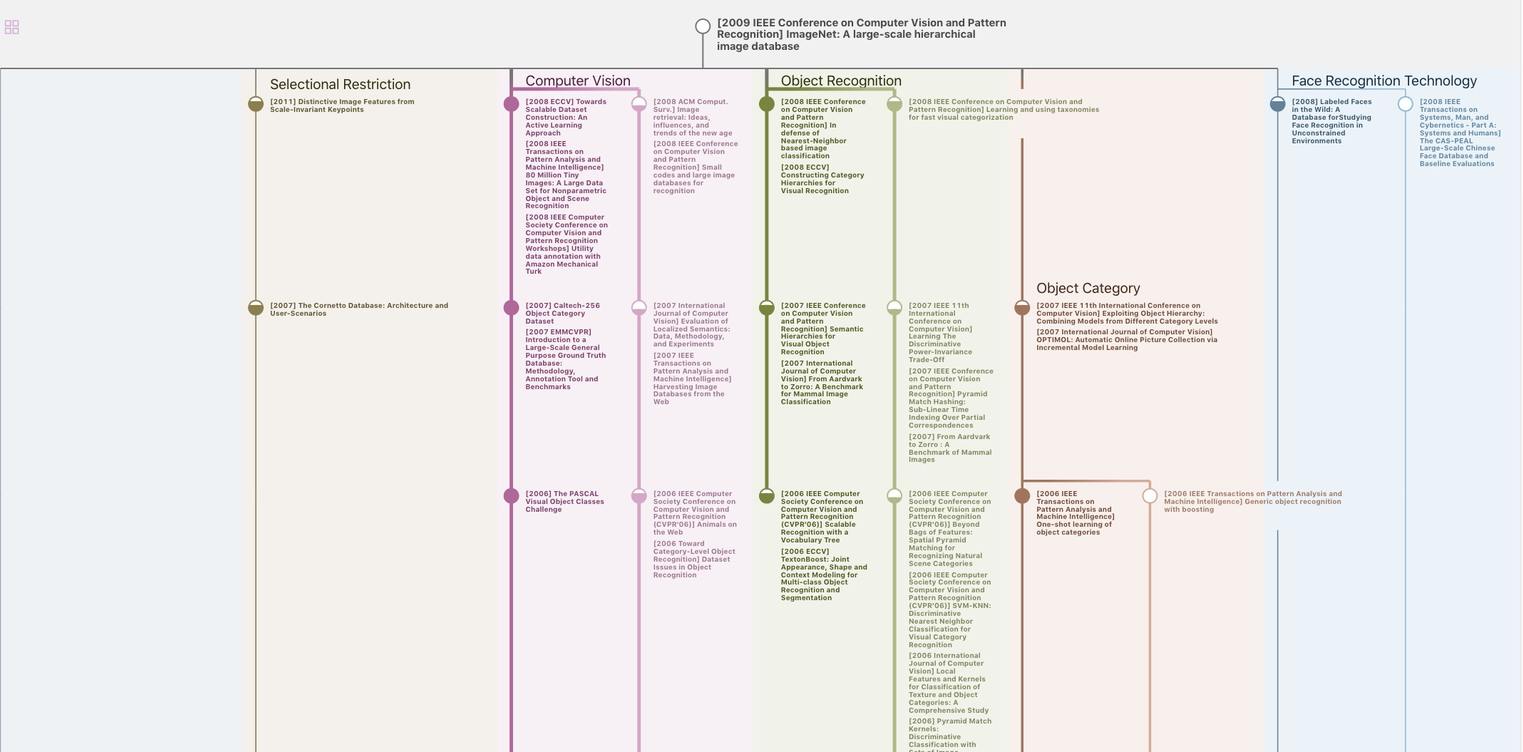
生成溯源树,研究论文发展脉络
Chat Paper
正在生成论文摘要