Improving Ground-Level NO2 Estimation in China Using GEMS Measurements and a Nested Machine Learning Model
crossref(2024)
摘要
Abstract. The major bridge linking satellite-derived vertical column densities (VCDs) of nitrogen dioxide (NO2) with ground-level concentration is theoretically the NO2 mixing height (NMH). Various meteorological parameters have been used as a proxy of NMH in existing studies. This study developed a nested machine learning model to convert VCDs of NO2 into ground-level NO2 concentrations across China using Geostationary Environmental Monitoring Spectrometer (GEMS) measurements. This nested model was designed to directly incorporate NMH into the methodological framework and explore its impact on performance. The inner machine learning model predicted the NMH from the meteorological parameters, which were then input into the main machine learning model to predict the ground-level NO2 concentrations from its VCDs. The inclusion of NMH significantly enhanced the accuracy of estimating ground-level NO2 concentration, reducing bias and improving R² values to 0.93 in 10-fold cross-validation and 0.99 in the fully-trained model. Furthermore, NMH was identified as the second most important predictor variable, following the VCDs of NO2. Subsequently, satellite-derived ground-level NO2 data were analyzed across subregions with varying geolocations and urbanization levels. Highly populated areas typically experienced peak NO2 concentrations during early morning rush hours, whereas areas categorized as lightly populated observed a slight increase in NO2 levels one or two hours later, likely due to regional pollutant dispersion from urban sources. This study underscores the importance of incorporating NMH in estimating ground-level NO2 from satellite column measurements and highlights the significant advantages of geostationary satellites in providing detailed air pollution information at an hourly resolution.
更多查看译文
AI 理解论文
溯源树
样例
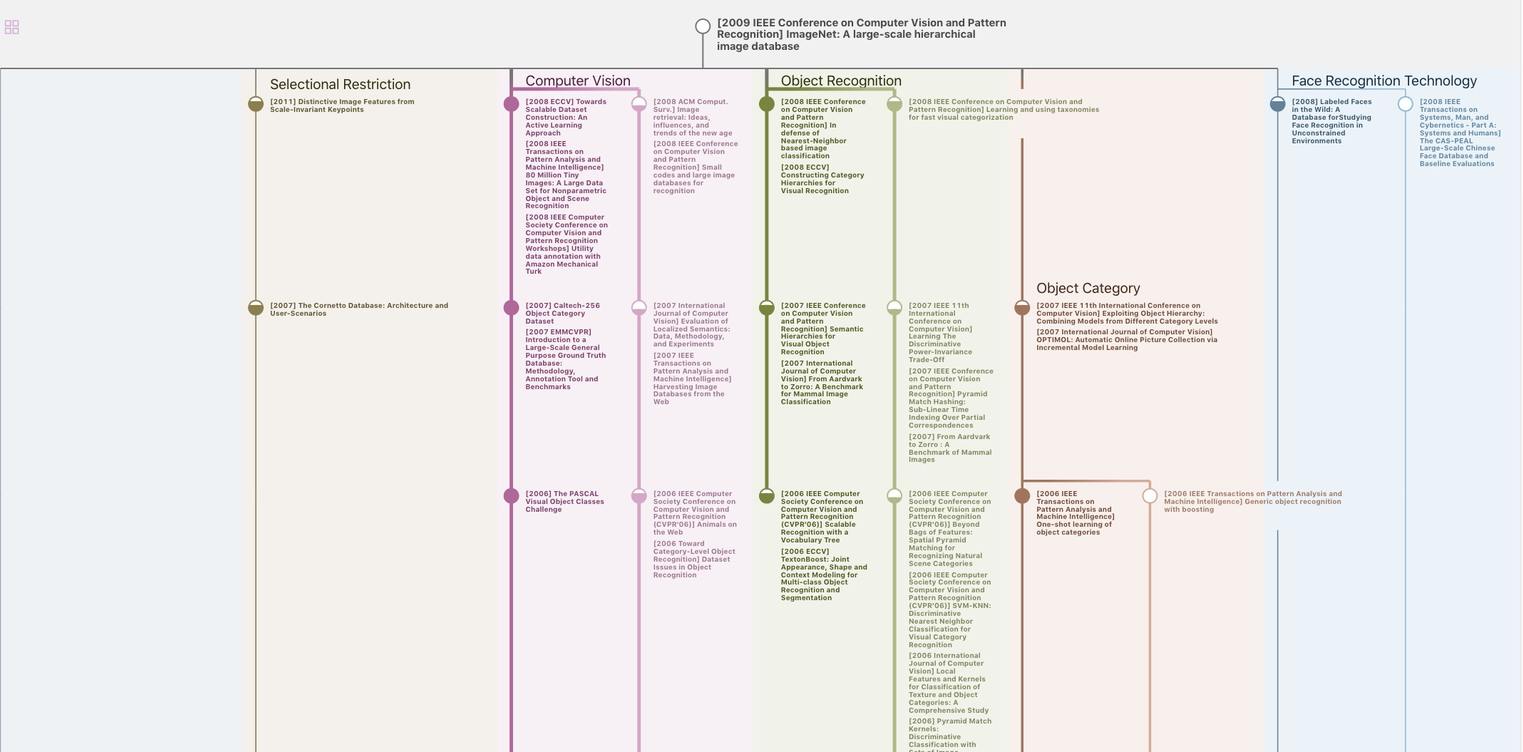
生成溯源树,研究论文发展脉络
Chat Paper
正在生成论文摘要