Joint Spatio-Temporal Similarity and Discrimination Learning for Visual Tracking
IEEE Transactions on Circuits and Systems for Video Technology(2024)
摘要
Visual tracking is a task of localizing a target unceasingly in a video with an initial target state at the first frame. The limited target information makes this problem an extremely challenging task. Existing tracking methods either perform matching based similarity learning or optimization based discrimination reasoning. However, these two types of tracking methods suffer from the problem of ineffectiveness for distinguishing target objects from background distractors and the problem of insufficiency in maintaining spatio-temporal consistency among successive frames, respectively. In this paper, we design a joint spatio-temporal similarity and discrimination learning (STSDL) framework for accurate and robust tracking. The designed framework is composed of two complementary branches: a similarity learning branch and a discrimination learning branch. The similarity learning branch uses an effective transformer encoder-decoder to gather rich spatio-temporal context information to generate a similarity map. In parallel, the discrimination learning branch exploits an efficient model predictor to train a target model to produce a discriminative map. Finally, the similarity map and the discriminative map are adaptively fused for accurate and robust target localization. Experimental results on six prevalent datasets demonstrate that the proposed STSDL can obtain satisfactory results, while it retains a real-time tracking speed of 50 FPS on a single GPU.
更多查看译文
关键词
video object tracking,joint learning,spatio-temporal similarity,spatio-temporal discrimination,adaptive response map fusion
AI 理解论文
溯源树
样例
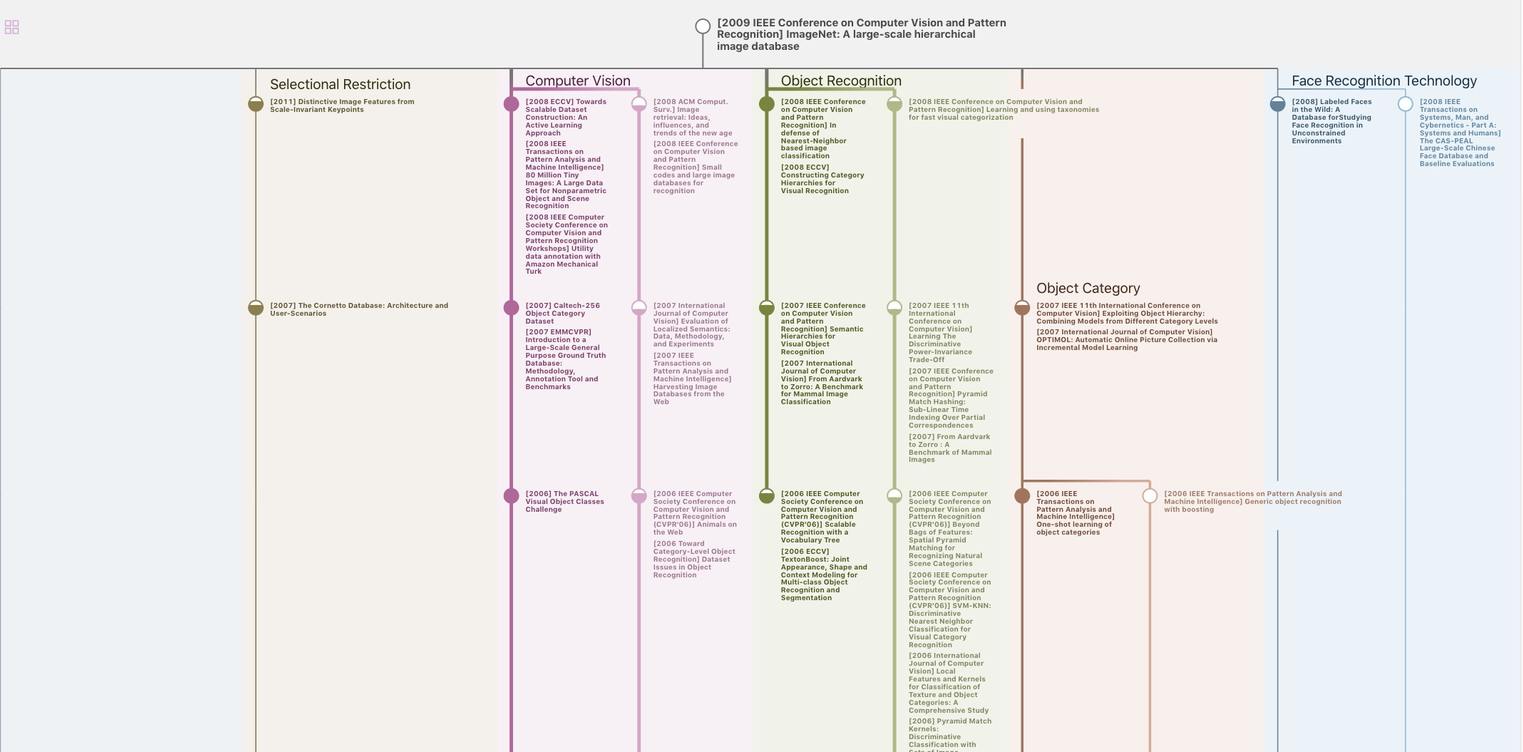
生成溯源树,研究论文发展脉络
Chat Paper
正在生成论文摘要