A novel multi-fidelity cokriging model assisted by multiple non-hierarchical low-fidelity datasets
Structural and Multidisciplinary Optimization(2024)
摘要
Multi-fidelity (MF) surrogate models for incorporating multiple non-hierarchical low-fidelity (LF) datasets, whose rank of fidelity level is unknown, have attracted much attention in engineering problems. However, most of existing approaches either need to build extra surrogate models for LF datasets in the fitting process or ignore the cross-correlations among these LF datasets, resulting in accuracy deterioration of an MF model. To address this, a novel multi-fidelity cokriging model is proposed in this article, termed as MCOK, which can incorporate arbitrary number of non-hierarchical LF datasets without building extra LF surrogate models. A self-contained derivation of MCOK predictor and its mean square error are presented. It puts all the covariances between any two MF datasets into a single matrix and introduces additional parameters “gamma” to account for their cross-correlations. A novel method for tuning these additional parameters in a latent space is developed to deal with the problem associated with non-positive definite correlation matrix. The proposed MCOK method is then validated against a set of numerical test cases and further demonstrated via an engineering example of aerodynamic data fusion for FDL-5A flight vehicle. Results from current test cases show that MCOK outperforms existing non-hierarchical cokriging, linear regression MF surrogate model, and latent-map Gaussian processes model, with more accurate and robust predictions, which makes it more practical for engineering modeling problems.
更多查看译文
关键词
Multi-fidelity surrogate model,Non-hierarchical datasets,Cokriging model,Aerodynamic data fusion,Correlation matrix
AI 理解论文
溯源树
样例
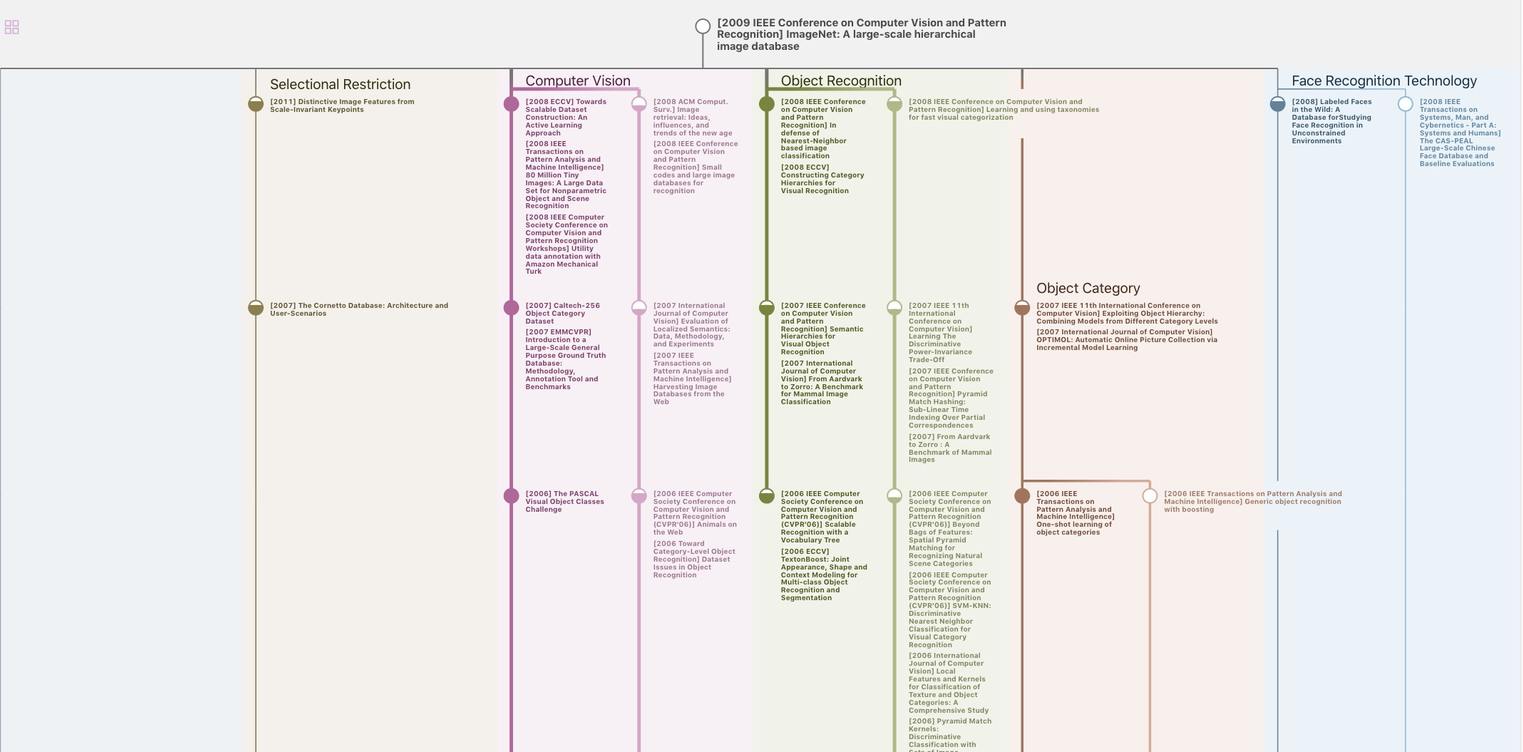
生成溯源树,研究论文发展脉络
Chat Paper
正在生成论文摘要