The Development and Performance of a Machine Learning Based Mobile Platform for Visually Determining the Etiology of Penile Pathology
arxiv(2024)
摘要
Machine-learning algorithms can facilitate low-cost, user-guided visual
diagnostic platforms for addressing disparities in access to sexual health
services. We developed a clinical image dataset using original and augmented
images for five penile diseases: herpes eruption, syphilitic chancres, penile
candidiasis, penile cancer, and genital warts. We used a U-net architecture
model for semantic pixel segmentation into background or subject image, the
Inception-ResNet version 2 neural architecture to classify each pixel as
diseased or non-diseased, and a salience map using GradCAM++. We trained the
model on a random 91
and evaluated the model on the remaining 9
sensitivity), precision, specificity, and F1-score (accuracy). Of the 239
images in the validation dataset, 45 (18.8
were of HSV infection, 29 (12.1
penile candidiasis, 37 (15.5
of non-diseased penises. The overall accuracy of the model for correctly
classifying the diseased image was 0.944. Between July 1st and October 1st
2023, there were 2,640 unique users of the mobile platform. Among a random
sample of submissions (n=437), 271 (62.0
(14.6
Kingdom, and 21 (4.8
18 and 30 years old. We report on the development of a machine-learning model
for classifying five penile diseases, which demonstrated excellent performance
on a validation dataset. That model is currently in use globally and has the
potential to improve access to diagnostic services for penile diseases.
更多查看译文
AI 理解论文
溯源树
样例
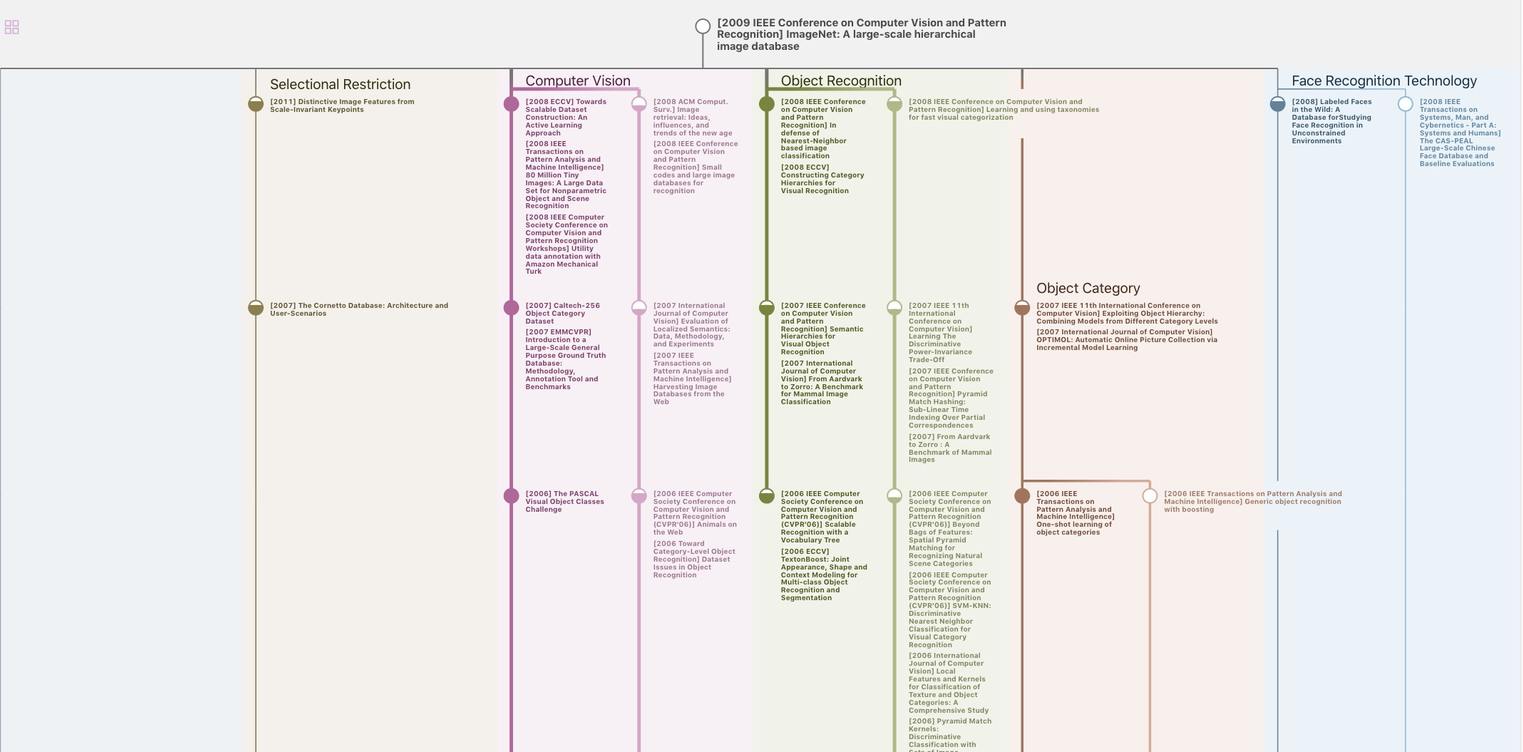
生成溯源树,研究论文发展脉络
Chat Paper
正在生成论文摘要