Question-Directed Reasoning With Relation-Aware Graph Attention Network for Complex Question Answering Over Knowledge Graph
IEEE/ACM Transactions on Audio, Speech, and Language Processing(2024)
摘要
Complex knowledge graph question answering (KGQA) aims at answering natural language questions by entities retrieving from a knowledge graph (KG). Recently, the relation path-based models have shown the unique advantage for complex KGQA. However, these existing models ignore the dependency between different relation paths, which leads to aimless reasoning over the KG. To resolve this issue, we propose the question-directed reasoning with relation-aware graph attention network (QRGAT) that encodes the reasoning process as a reasoning graph. The relation-aware GAT can recognize neighbor entities along with the corresponding relations for each entity. With the relation-aware GAT stacked in multiple layers, it can collaboratively capture the dependency of different relation paths for each entity. The question-directed reasoning utilizes the information learned by the relation-aware GAT to solve the aimless reasoning on the KG by constructing a reasoning graph. Extensive experiments demonstrate that our QRGAT outperforms the baseline models on both popular datasets WebQuestionsSP and ComplexWebQuestions. Compared with the strong GNN-based baseline NSM
$_{+h}$
, our QRGAT achieves the performance improvements of 2.3% on WebQuestionsSP and 3.6% on ComplexWebQuestions by the metric Hits@1.
更多查看译文
关键词
Graph attention network,information retrieval,knowledge graph,question answering
AI 理解论文
溯源树
样例
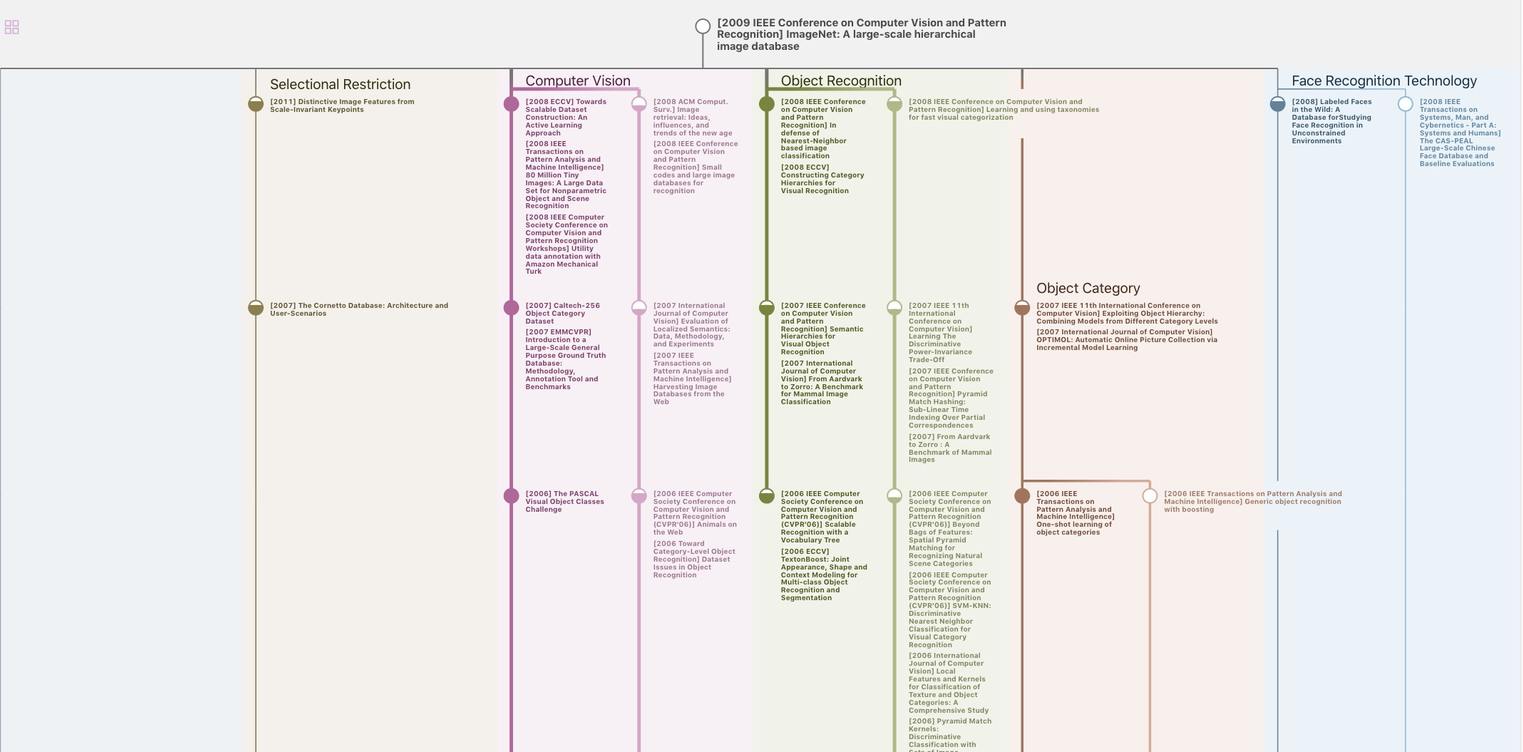
生成溯源树,研究论文发展脉络
Chat Paper
正在生成论文摘要