LAMPlace: Legalization-Aided Reinforcement Learning Based Macro Placement for Mixed-Size Designs With Preplaced Blocks
IEEE Transactions on Circuits and Systems II: Express Briefs(2024)
摘要
Macro placement is a crucial and complex task in chip design. In recent years, reinforcement learning (RL) has emerged as a promising approach for autonomously generating effective macro placement on a grid-based chip canvas. However, existing RL-based macro placers encounter challenges when handling macros of varying sizes and preplaced blocks, due to using coarse-grained grids leads to wastage of placement space, while employing fine-grained grids results in an exponentially growing solution space and significant computational overhead. This brief proposes a legalization-aided RL-based macro placement (LAMPlace) for mixed-size designs with preplaced blocks. LAMPlace leverages RL to generate a global macro placement that allows possible overlap, followed by a macro legalization algorithm to ensure the legality of macro placement. LAMPlace can effectively address macros of various sizes and preplaced blocks because the action mask generation algorithm in RL is well coupled with the macro legalization algorithm. Compared with the state-of-the-art works, experimental results on publicly mixed-size placement benchmarks show that LAMPlace is effective.
更多查看译文
关键词
Physical design,reinforcement learning,artificial neural network,macro placement,mixed-size designs
AI 理解论文
溯源树
样例
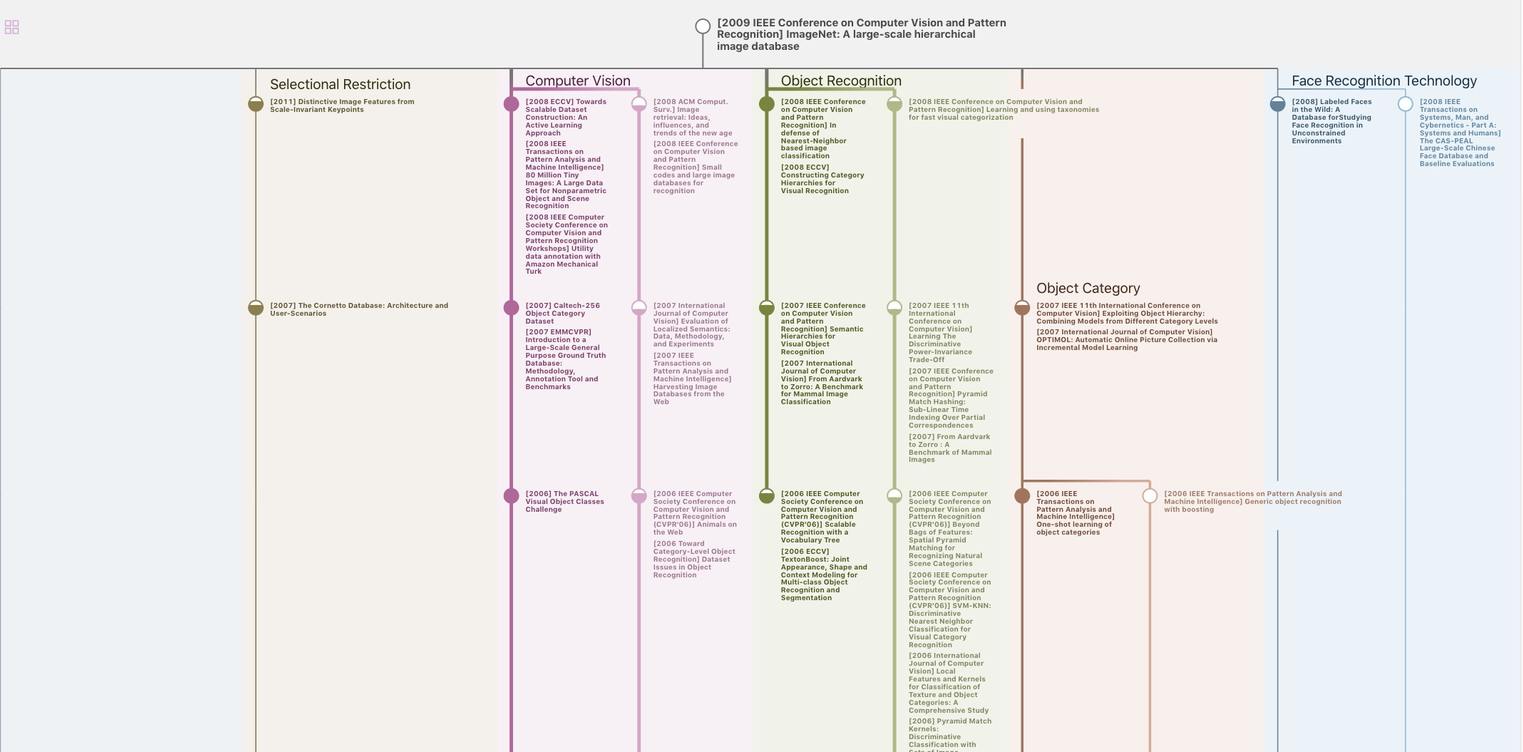
生成溯源树,研究论文发展脉络
Chat Paper
正在生成论文摘要