VLKEB: A Large Vision-Language Model Knowledge Editing Benchmark
CoRR(2024)
Abstract
Recently, knowledge editing on large language models (LLMs) has received
considerable attention. Compared to this, editing Large Vision-Language Models
(LVLMs) faces extra challenges from diverse data modalities and complicated
model components, and data for LVLMs editing are limited. The existing LVLM
editing benchmark, which comprises three metrics (Reliability, Locality, and
Generality), falls short in the quality of synthesized evaluation images and
cannot assess whether models apply edited knowledge in relevant content.
Therefore, we employ more reliable data collection methods to construct a new
Large $\textbf{V}$ision-$\textbf{L}$anguage Model $\textbf{K}$nowledge
$\textbf{E}$diting $\textbf{B}$enchmark, $\textbf{VLKEB}$, and extend the
Portability metric for more comprehensive evaluation. Leveraging a multi-modal
knowledge graph, our image data are bound with knowledge entities. This can be
further used to extract entity-related knowledge, which constitutes the base of
editing data. We conduct experiments of different editing methods on five
LVLMs, and thoroughly analyze how do they impact the models. The results reveal
strengths and deficiencies of these methods and hopefully provide insights for
future research. The codes and dataset are available at:
$\href{https://github.com/VLKEB/VLKEB}{\text{https://github.com/VLKEB/VLKEB}}$.
MoreTranslated text
AI Read Science
Must-Reading Tree
Example
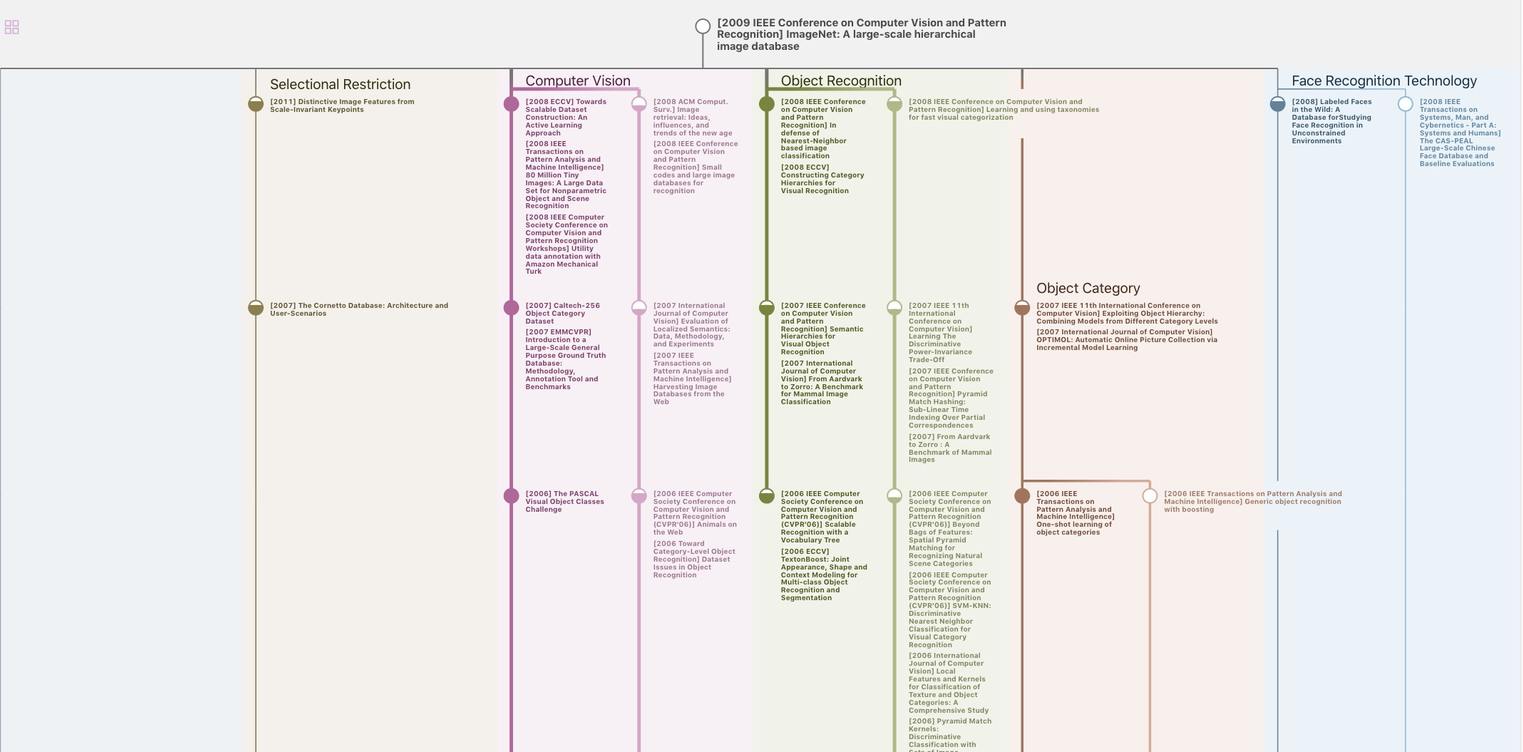
Generate MRT to find the research sequence of this paper
Chat Paper
Summary is being generated by the instructions you defined