AdaNovo: Adaptive De Novo Peptide Sequencing with Conditional Mutual Information
arxiv(2024)
Abstract
Tandem mass spectrometry has played a pivotal role in advancing proteomics,
enabling the analysis of protein composition in biological samples. Despite the
development of various deep learning methods for identifying amino acid
sequences (peptides) responsible for observed spectra, challenges persist in
de novo peptide sequencing. Firstly, prior methods struggle to identify
amino acids with post-translational modifications (PTMs) due to their lower
frequency in training data compared to canonical amino acids, further resulting
in decreased peptide-level identification precision. Secondly, diverse types of
noise and missing peaks in mass spectra reduce the reliability of training data
(peptide-spectrum matches, PSMs). To address these challenges, we propose
AdaNovo, a novel framework that calculates conditional mutual information (CMI)
between the spectrum and each amino acid/peptide, using CMI for adaptive model
training. Extensive experiments demonstrate AdaNovo's state-of-the-art
performance on a 9-species benchmark, where the peptides in the training set
are almost completely disjoint from the peptides of the test sets. Moreover,
AdaNovo excels in identifying amino acids with PTMs and exhibits robustness
against data noise. The supplementary materials contain the official code.
MoreTranslated text
AI Read Science
Must-Reading Tree
Example
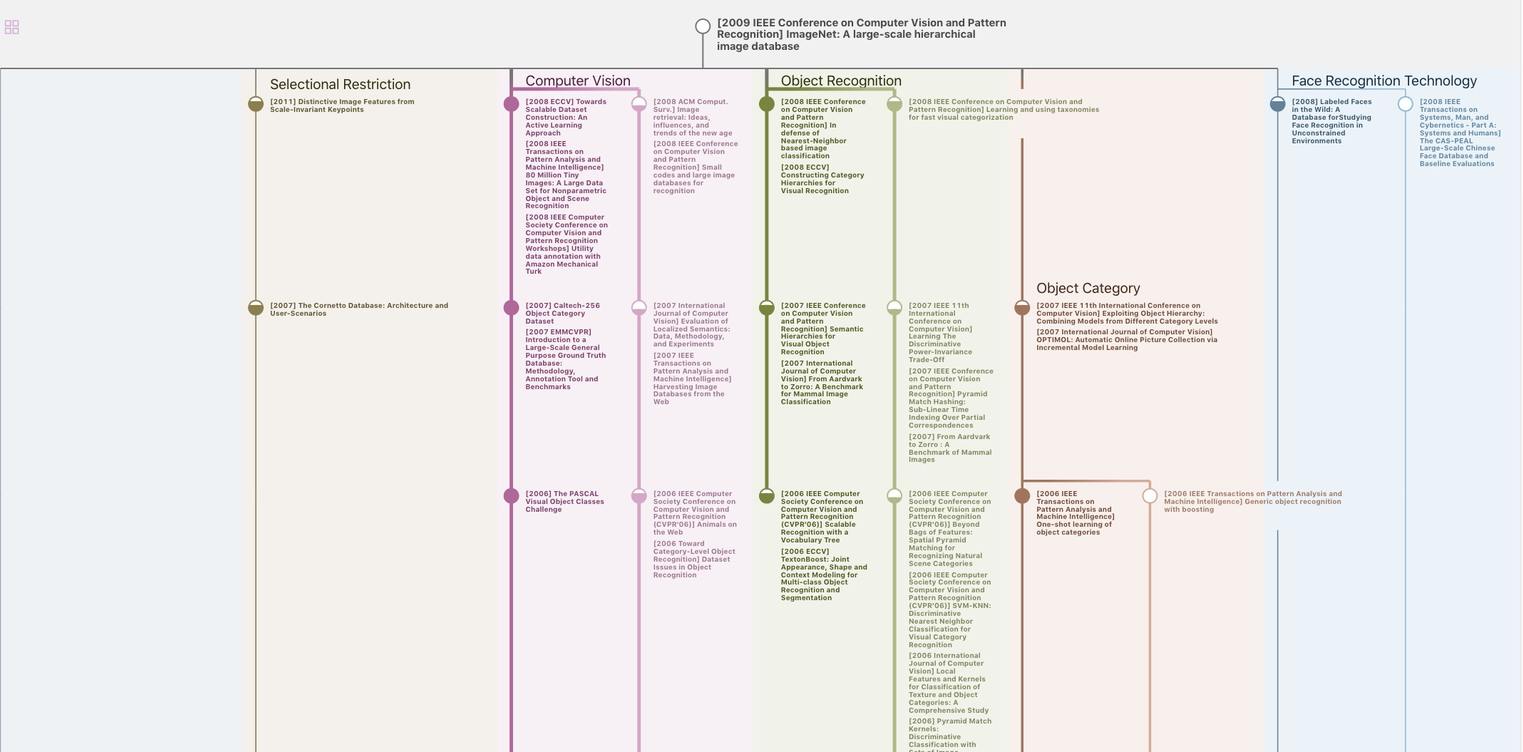
Generate MRT to find the research sequence of this paper
Chat Paper
Summary is being generated by the instructions you defined