Semi-Supervised 3D Shape Segmentation via Self Refining.
IEEE transactions on image processing : a publication of the IEEE Signal Processing Society(2024)
摘要
3D shape segmentation is a fundamental and crucial task in the field of image processing and 3D shape analysis. To segment 3D shapes using data-driven methods, a fully labeled dataset is usually required. However, obtaining such a dataset can be a daunting task, as manual face-level labeling is both time-consuming and labor-intensive. In this paper, we present a semi-supervised framework for 3D shape segmentation that uses a small, fully labeled set of 3D shapes, as well as a weakly labeled set of 3D shapes with sparse scribble labels. Our framework first employs an auxiliary network to generate initial fully labeled segmentation labels for the sparsely labeled dataset, which helps in training the primary network. During training, the self-refine module uses increasingly accurate predictions of the primary network to improve the labels generated by the auxiliary network. Our proposed method achieves better segmentation performance than previous semi-supervised methods, as demonstrated by extensive benchmark tests, while also performing comparably to supervised methods.
更多查看译文
关键词
3D shape segmentation,Semi-Supervised,Deep neural network
AI 理解论文
溯源树
样例
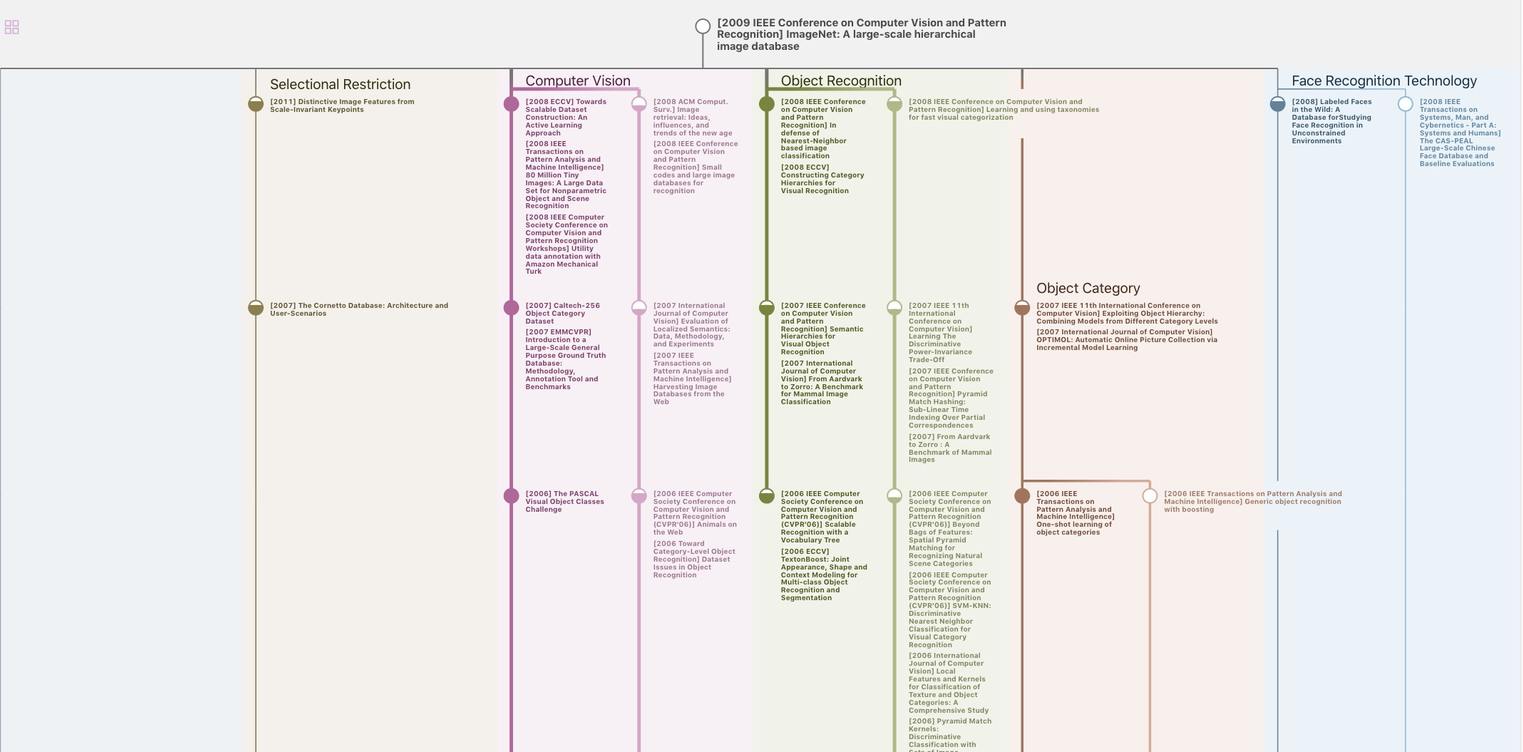
生成溯源树,研究论文发展脉络
Chat Paper
正在生成论文摘要