Magnifying Networks for Histopathological Images with Billions of Pixels.
Diagnostics (Basel, Switzerland)(2024)
摘要
Amongst the other benefits conferred by the shift from traditional to digital pathology is the potential to use machine learning for diagnosis, prognosis, and personalization. A major challenge in the realization of this potential emerges from the extremely large size of digitized images, which are often in excess of 100,000 × 100,000 pixels. In this paper, we tackle this challenge head-on by diverging from the existing approaches in the literature-which rely on the splitting of the original images into small patches-and introducing magnifying networks (MagNets). By using an attention mechanism, MagNets identify the regions of the gigapixel image that benefit from an analysis on a finer scale. This process is repeated, resulting in an attention-driven coarse-to-fine analysis of only a small portion of the information contained in the original whole-slide images. Importantly, this is achieved using minimal ground truth annotation, namely, using only global, slide-level labels. The results from our tests on the publicly available Camelyon16 and Camelyon17 datasets demonstrate the effectiveness of MagNets-as well as the proposed optimization framework-in the task of whole-slide image classification. Importantly, MagNets process at least five times fewer patches from each whole-slide image than any of the existing end-to-end approaches.
更多查看译文
关键词
histology,histopathology,deep learning,whole slide image,digital pathology,gigapixel images
AI 理解论文
溯源树
样例
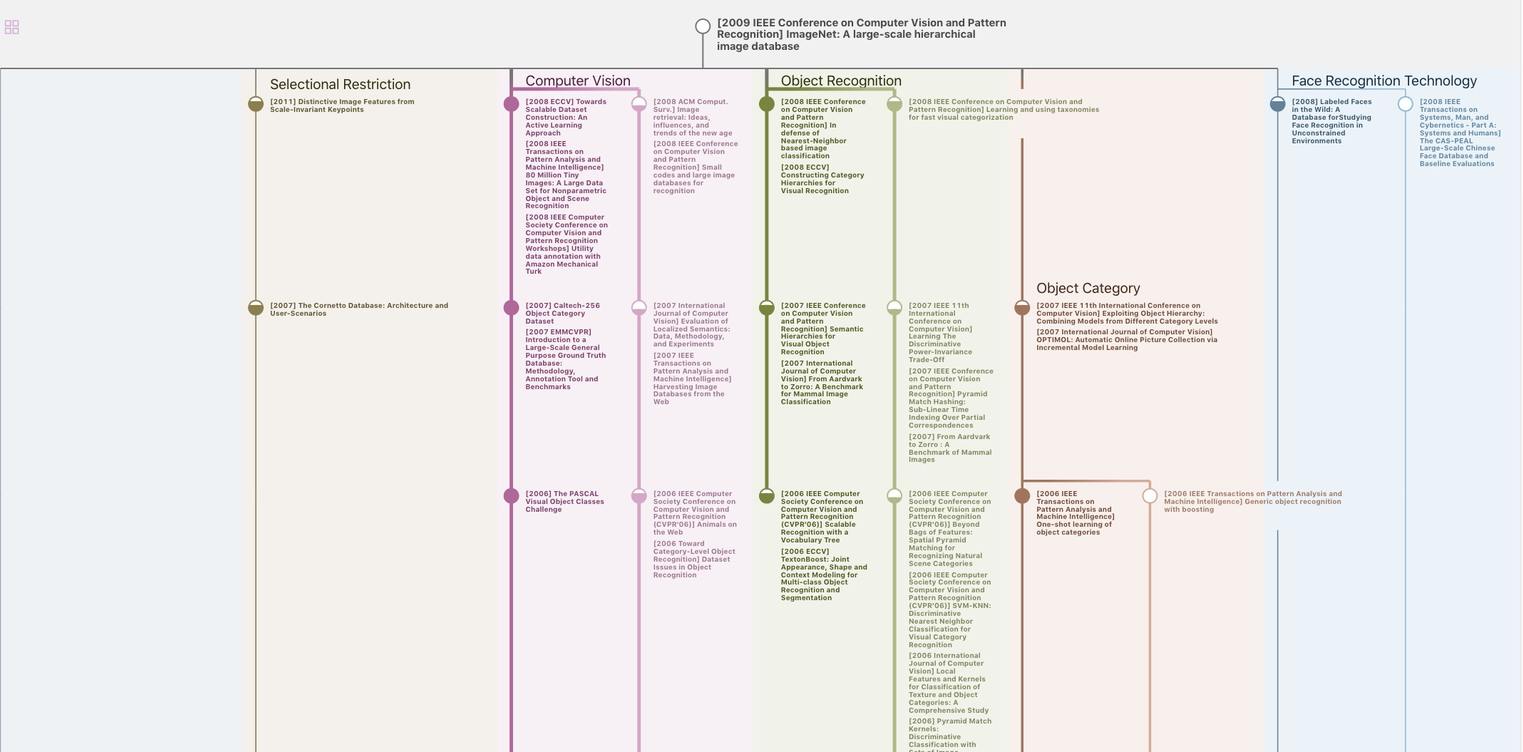
生成溯源树,研究论文发展脉络
Chat Paper
正在生成论文摘要